Structural Equivariance Self-Supervised Learning for Facial Pose Estimation
2023 IEEE INTERNATIONAL CONFERENCE ON MULTIMEDIA AND EXPO, ICME(2023)
摘要
In this paper, we propose a self-supervised learning method for robust facial pose estimation. Conventional methods usually split the coherent head motion into discrete and finite outputs, likely leading to bias prediction because the performance of head pose estimation highly relies on structural facial appearance. To address this issue, our model achieves structural equivariance to poses through a self-supervised learning strategy from extrinsic attributes of face neighbors and underlying local associations. Specifically, we construct a complete neighbor graph to capture the extrinsic properties of face neighbors, where different latent semantic attributes are assigned to each subgraph. Accordingly, we design a set of proxy tasks based on different attribute subgraphs, where the model is encouraged to learn the underlying relation of local features under pose variation. Extensive experimental results on the challenging, widely evaluated datasets indicate the effectiveness of our model compared with the state of the arts.
更多查看译文
关键词
Facial pose estimation, face alignment, self-supervised learning, structural learning, biometrics
AI 理解论文
溯源树
样例
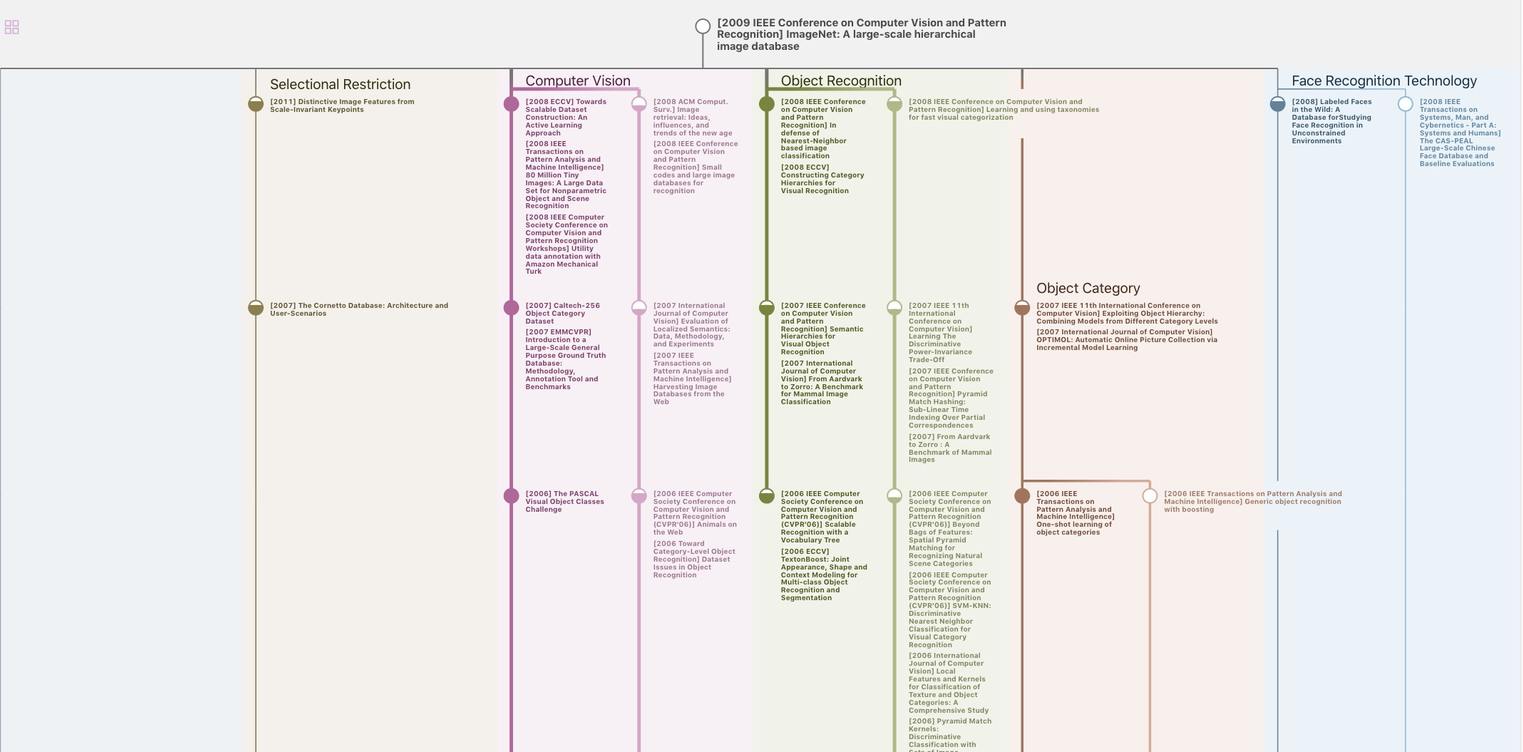
生成溯源树,研究论文发展脉络
Chat Paper
正在生成论文摘要