Hierarchical Attention Learning for Multimodal Classification
2023 IEEE INTERNATIONAL CONFERENCE ON MULTIMEDIA AND EXPO, ICME(2023)
摘要
Multimodal learning aims to integrate complementary information from different modalities for more reliable decisions. However, existing multimodal classification methods simply integrate the learned local features, which ignore the underlying structure of each modality and the higher-order correlation across modalities. In this paper, we propose a novel Hierarchical Attention Learning Network (HALNet) for multi-modal classification. Specifically, HALNet has three merits: 1) A hierarchical feature fusion module is proposed to learn multi-level features, aggregating multi-level features for a global feature representation with the attention mechanism and progressive fusion tactics. 2) A cross-modal higher-order fusion module is introduced to capture the prospective cross-modal correlations at label space. 3) A dual prediction pattern is designed to generate credible decisions. Extensive experiments on three real-world multimodal datasets demonstrate that HALNet achieves competitive performance compared to the state-of-the-art.
更多查看译文
关键词
Multimodal classification,hierarchical attention learning,cross-modal fusion,dual prediction
AI 理解论文
溯源树
样例
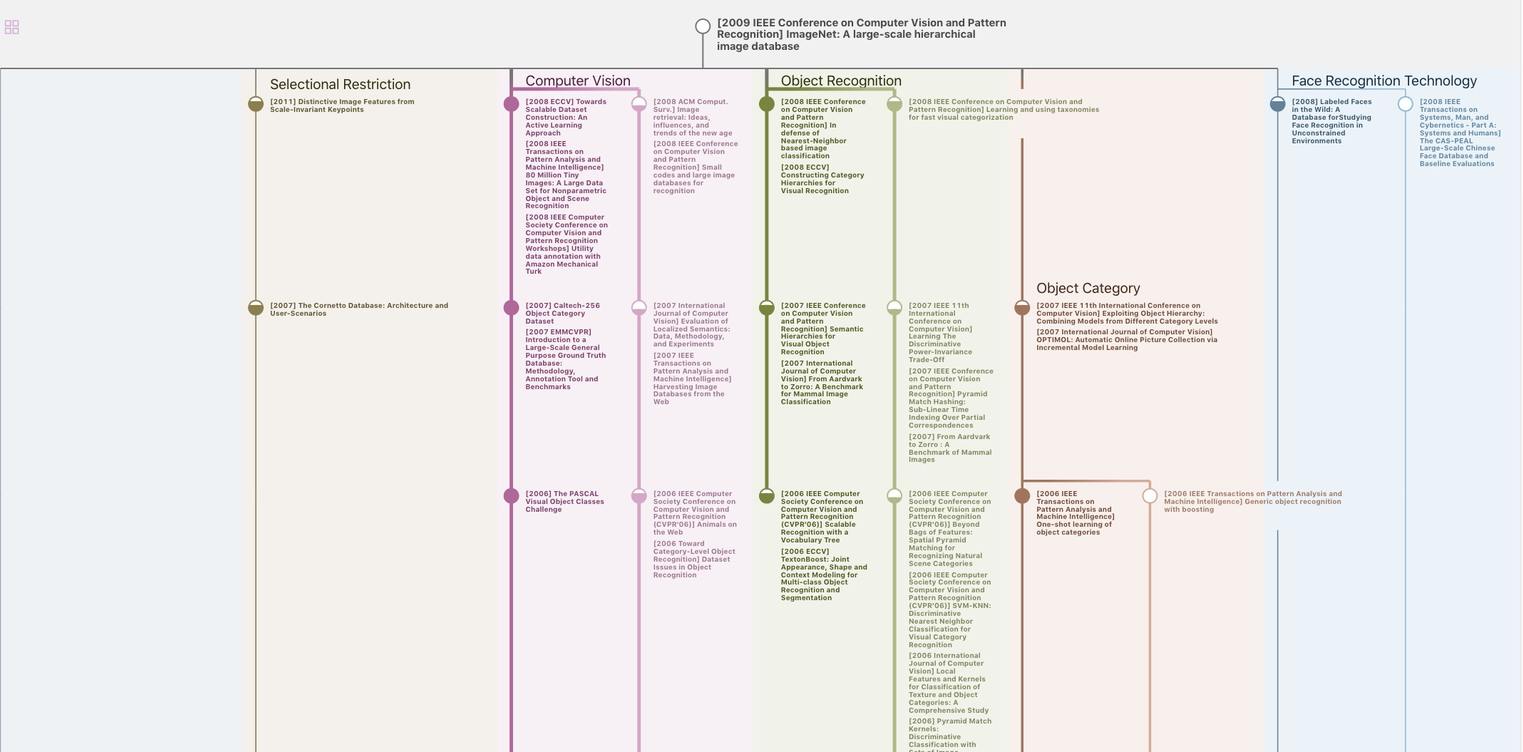
生成溯源树,研究论文发展脉络
Chat Paper
正在生成论文摘要