Get a head start: targeted labeling at source with limited annotation overhead for semi-supervised learning
2023 IEEE INTERNATIONAL CONFERENCE ON MULTIMEDIA AND EXPO, ICME(2023)
摘要
Semi-supervised learning (SSL), which leverages limited labeled data and a large amount of unlabeled data for model training, has been widely studied to mitigate the requirement for expensive and time-consuming annotations. Recently proposed methods have achieved promising yet unstable results, which presume that initial samples are randomly selected and labeled. For improving the fluctuated performance while saving annotation overhead, effective prior labeling for SSL on the source cluttered unlabeled dataset is challenging but significant. In this paper, we propose a novel criterion and a distribution balance strategy to automatically achieve targeted labeling without access to the test set and any labels. Comprehensive experiments are conducted on commonly-used datasets to demonstrate the effectiveness of our method. Furthermore, targeted labeling is orthogonal to existing framework-centric SSL methods and can achieve state-of-the-art performance.
更多查看译文
关键词
Semi-supervised learning,Targeted labeling,Feature,Distribution,Annotation overhead
AI 理解论文
溯源树
样例
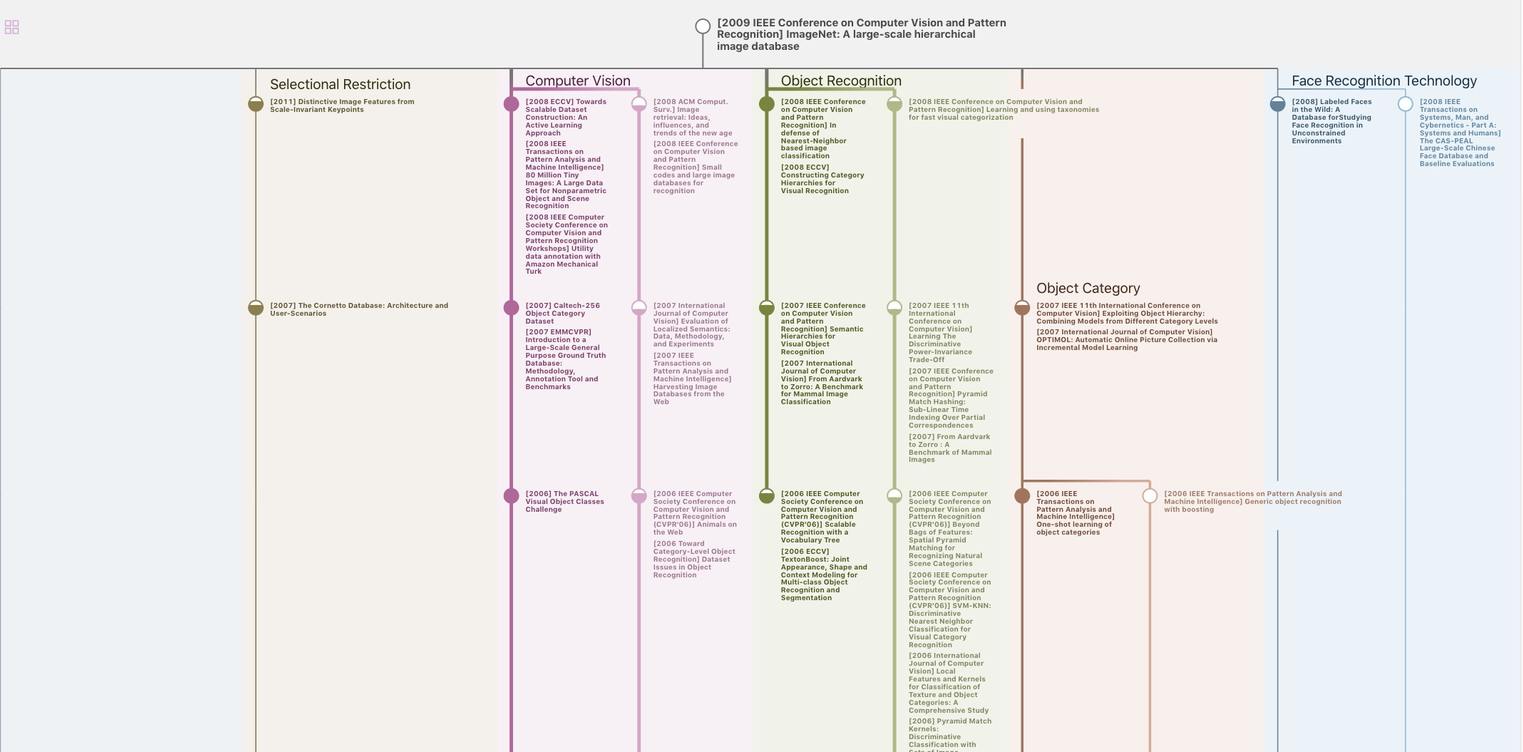
生成溯源树,研究论文发展脉络
Chat Paper
正在生成论文摘要