From Regression to Classification: Fuzzy Multikernel Subspace Learning for Robust Prediction and Drug Screening
IEEE TRANSACTIONS ON INDUSTRIAL INFORMATICS(2023)
摘要
Data-driven machine learning is increasingly involved in human life and industrial development due to its large-scale testing and low time cost. However, existing learning algorithms are not suitable for real-world applications with data dilemmas, such as extremely high-dimension-low-sample-size problems, non-Gaussian noise, and uncertainty. In this article, we propose a novel fuzzy multikernel subspace learning (FMKSL) to address these problems, which provides a robust multikernel representation with a fuzzy constraint and sparse coding. We then develop an adaptive learner chain optimization method based on the iterative process of FMKSL to speed up learning and achieve the best performance. Different from previous methods, we also design a flexible data augmentation method, namely generalized correntropy-based adaptive data augmentation (GC-ADA), to effectively use the alpha-order statistics between samples to transform the exact value prediction task into a simpler classification one. It is important that our general framework only needs an extremely small dataset to predict the related ranking of the sample since the exact label value measured by different institutions in reality varies largely. A typical scenario is the drug screening task, i.e., the inhibitory potency prediction of the nicotinamide phosphoribosyltransferase inhibitors. Extensive experiments on nine real-world datasets (four tasks) show that our framework outperforms state-of-the-art methods in prioritizing candidate samples and chemicals for experimental research and analysis via a data-driven computational approach.
更多查看译文
关键词
Data-driven machine learning,drug screening,fuzzy multikernel subspace learning (FMKSL),robust
AI 理解论文
溯源树
样例
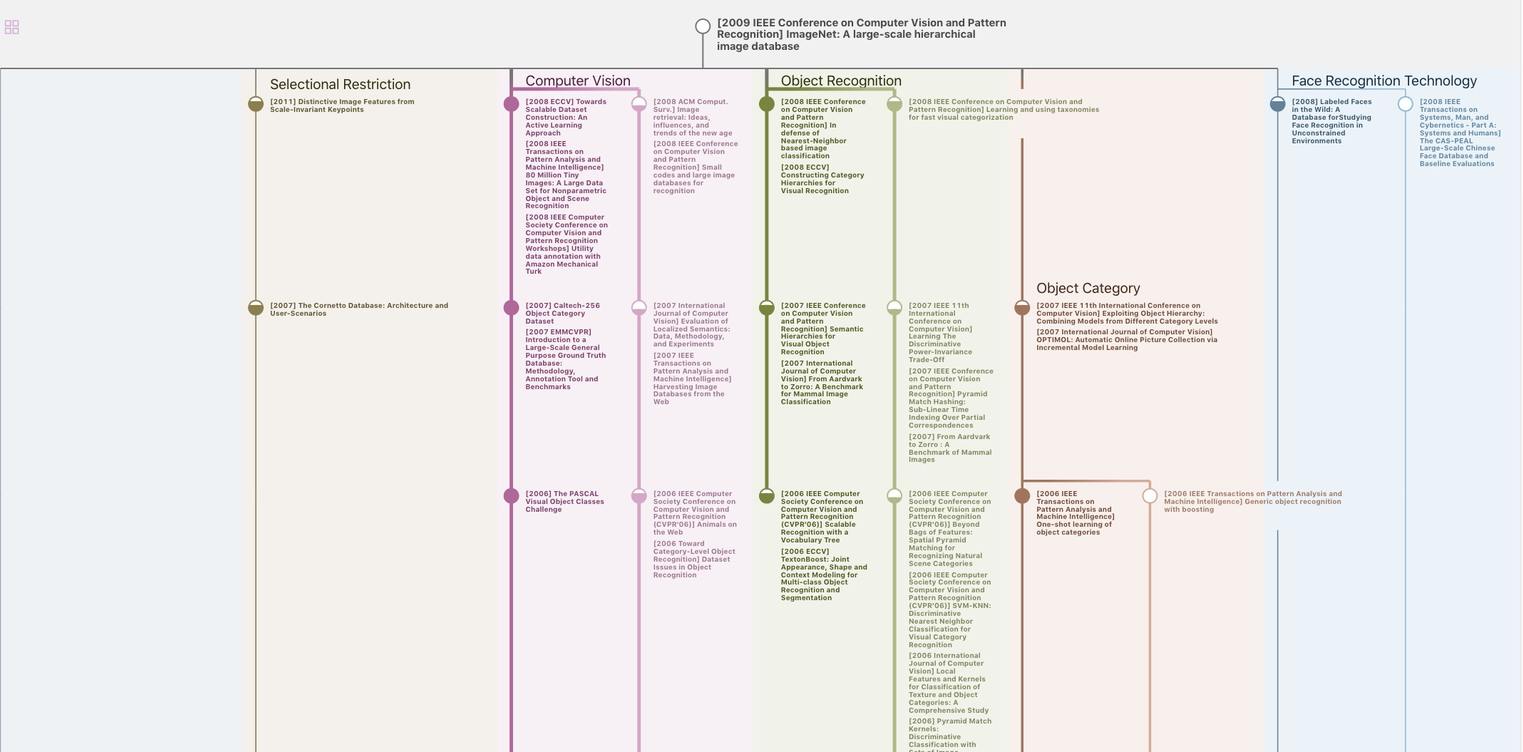
生成溯源树,研究论文发展脉络
Chat Paper
正在生成论文摘要