MSEN: A Multi-Scale Evolutionary Network for Modeling the Evolution of Temporal Knowledge Graphs
FUTURE INTERNET(2023)
摘要
Temporal knowledge graphs play an increasingly prominent role in scenarios such as social networks, finance, and smart cities. As such, research on temporal knowledge graphs continues to deepen. In particular, research on temporal knowledge graph reasoning holds great significance, as it can provide abundant knowledge for downstream tasks such as question answering and recommendation systems. Current reasoning research focuses primarily on interpolation and extrapolation. Extrapolation research aims to predict the likelihood of events occurring in future timestamps. Historical events are crucial for predicting future events. However, existing models struggle to fully capture the evolutionary characteristics of historical knowledge graphs. This paper proposes a multi-scale evolutionary network (MSEN) model that leverages Hierarchical Transfer aware Graph Neural Network (HT-GNN) in a local memory encoder to aggregate rich structural semantics from each timestamp's knowledge graph. It also utilizes Time Related Graph Neural Network (TR-GNN) in a global memory encoder to model temporal-semantic dependencies of entities across the global knowledge graph, mining global evolutionary patterns. The model integrates information from both encoders to generate entity embeddings for predicting future events. The proposed MSEN model demonstrates strong performance compared to several baselines on typical benchmark datasets. Results show MSEN achieves the highest prediction accuracy.
更多查看译文
关键词
knowledge graph,temporal knowledge graph,knowledge graph reasoning,evolution of temporal knowledge graphs
AI 理解论文
溯源树
样例
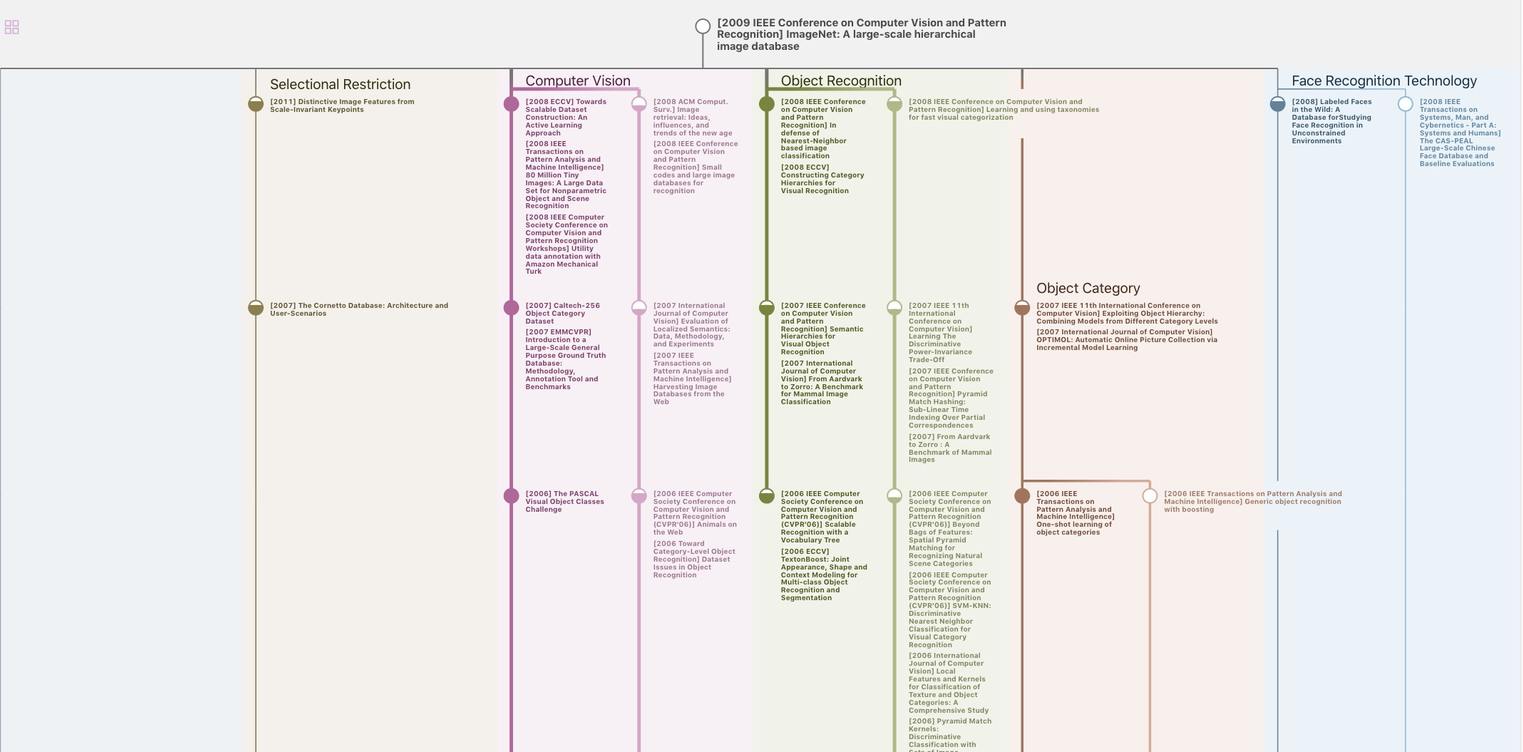
生成溯源树,研究论文发展脉络
Chat Paper
正在生成论文摘要