Representation of materials by kernel mean embedding
PHYSICAL REVIEW B(2023)
摘要
For using machine learning to predict material properties, the feature representation of the materials given to the model plays a fundamental role. A model describes material properties as a function of any given material system expressed as a fixed-length numeric vector, often called a descriptor. However, in most cases, the variables of interest are nontrivial for encoding their compositional or structural features, such as molecules, crystal systems, chemical compositions, and composite materials, into a fixed-length vector. Conventionally, to translate such a multicomponent system into a fixed-length vector, the distribution of predefined component features is summarized into a few summary statistics. The disadvantage of this reduction operation is that some distributional information, such as multimodality, is lost in the vectorization process. Here, we present a general class of material descriptors motivated by the machine-learning theory of kernel mean embedding. Unlike conventional descriptors, kernel mean embedding can retain all information regarding the distribution of component features in the vectorization process. Furthermore, the kernel mean descriptor uniquely determines the inverse map to the original material space. We demonstrate the expressive power and versatility of the kernel mean descriptor in various applications, including the prediction of the formation energy of inorganic compounds, prediction of the chemical composition to form quasicrystalline materials, and the use of force-field parameters to characterize polymeric materials.
更多查看译文
关键词
materials,kernel,representation
AI 理解论文
溯源树
样例
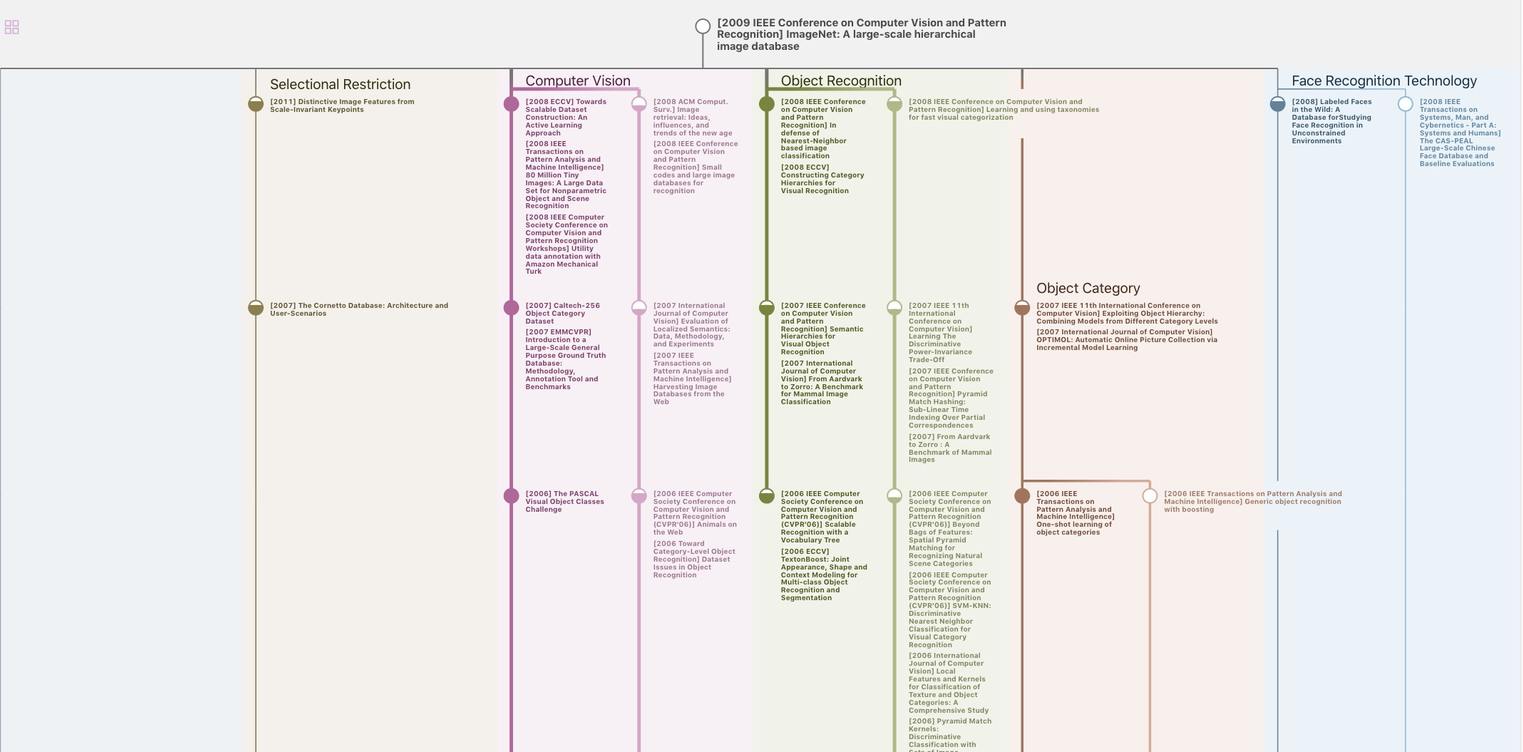
生成溯源树,研究论文发展脉络
Chat Paper
正在生成论文摘要