State-input affine approximate modeling based on a differential neural network identifier
APPLIED MATHEMATICAL MODELLING(2024)
摘要
This work presents the development of a state-input affine differential neural network that approximates a class of nonlinear systems with uncertain dynamics and perturbations. The feasibility of using differential neural networks is based on the approximation capabilities of artificial neural networks, assuring that the approximation error of the right-hand side of the system with uncertain dynamics is bounded. The dynamics of the free parameters of the differential neural network is obtained using the Lyapunov's second stability method, which provides the convergence of the identification error to an invariant set around the origin. The approximation capabilities of the proposed identifier are compared with a classical differential neural network, aiming to identify the dynamics of a Stewart platform, which is used as testing example. The results show that the cumulative norm of identification's error is smaller using the state-input affine differential neural network, which demonstrates better identification outcomes with the proposed modified identifier. An additional advantage of the state-input affine differential neural network is that it has a quasi-linear structure that could help formulate a controller for several systems, which is a further research interest.
更多查看译文
关键词
System identification,Artificial neural networks,Lyapunov control functions,State-input affine systems,System linearization,Pseudo linear systems control,Stewart platform
AI 理解论文
溯源树
样例
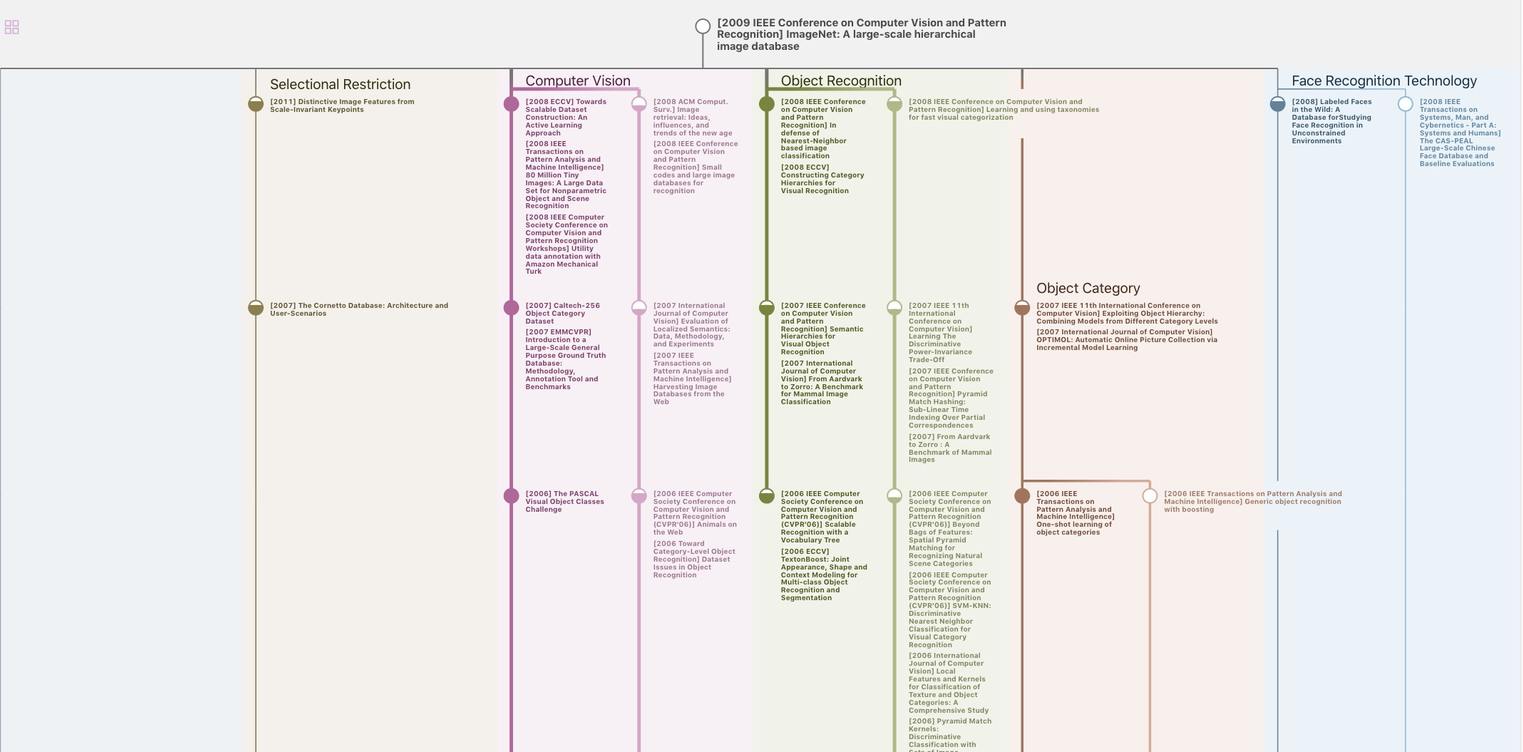
生成溯源树,研究论文发展脉络
Chat Paper
正在生成论文摘要