MR image reconstruction using iterative up and downsampling network
EXPERT SYSTEMS WITH APPLICATIONS(2024)
摘要
Magnetic resonance imaging (MRI) is one of the most significant modalities in medical imaging. It suffers from rather lengthy acquisition times, which lead to prolonged patient restriction, patient discomfort, and imaging artifacts. Hence, satisfactory MRI reconstruction from undersampled data sequences constitutes an important research problem. With the advances in deep learning (DL), a plethora of new models have been proposed to solve the MRI reconstruction problem using deep networks. On the other hand, single image super-resolution (SR) is another well-studied field that benefited from the success of DL, and it has applications in various imaging modalities. SR is the process of recovering a high-resolution image from a low-resolution image. SR models work on low-resolution images to recover missing details. MR image reconstruction on the other hand is a battle to eliminate the aliasing artifacts which originate from data downsampling. The motivation for the proposed work is based on the premise that SR approaches can possibly get adapted to MR image reconstruction. Hence in this study, inspired by the great success of deep SR networks, we customize an architecture introduced in SR setting to MRI reconstruction. This novel approach uses the iterative up and downsampling framework labeled as Iterative Up and Down Network (IUDN) for MRI reconstruction. We design two variants of the proposed network with different number of scale factors. We present extensive simulations for the proposed architecture using multiple k-space undersampling ratios. The simulation results indicate cutting edge MRI reconstruction performance for the proposed models. The networks were trained on fastMRI dataset and tested on both fastMRI and IXI datasets to show the robustness of the method. The proposed models achieved improved results in terms of PSNR and visual quality of the reconstructed image when compared to some recent state-of-the-art solutions for MRI reconstruction.
更多查看译文
关键词
Magnetic resonance imaging,Image reconstruction,Deep learning,Super-resolution,Iterative up and downsampling
AI 理解论文
溯源树
样例
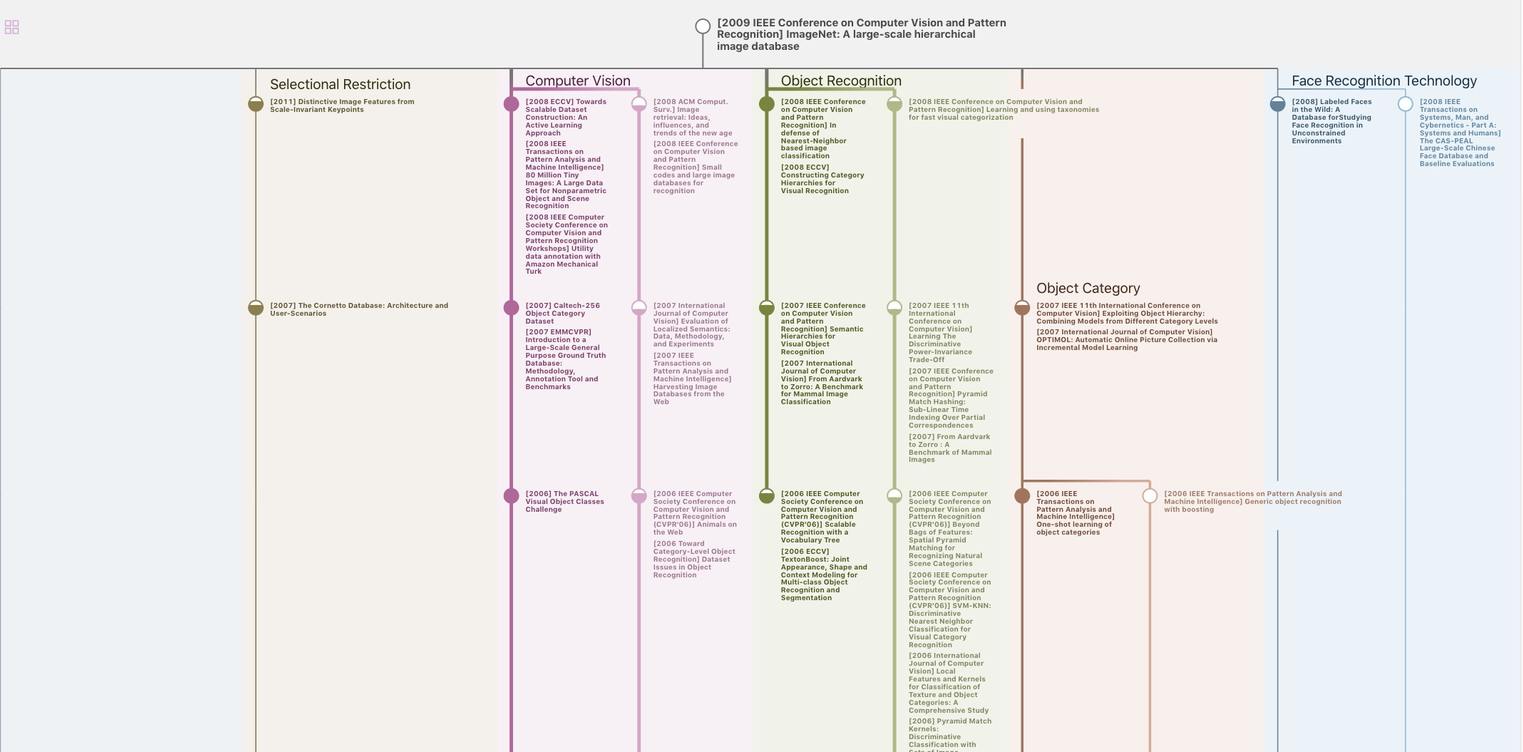
生成溯源树,研究论文发展脉络
Chat Paper
正在生成论文摘要