Machine learning prediction of physical properties and nitrogen content of porous carbon from agricultural wastes: Effects of activation and doping process
FUEL(2024)
摘要
In the study, machine learning models for prediction specific surface area (SSA), total pore volume (TPV), microporosity (MP) and nitrogen content (NC) were developed based on three tree-based models by collecting published experimental data on the agricultural wastes for the preparation of nitrogen-doped porous carbon, in which the physicochemical properties of raw materials and activation conditions were considered and compared as input features. By randomly combining different groups of input features in the three models, gradient boosted decision tree (GBDT) with the combination of all features presented the highest test R2 of 0.939, 0.835, 0.861 and 0.906 for the four prediction targets with high accuracy and good generalization ability. The visual analysis of the model showed that the activation temperature and impregnation ratio were the most critical factors for the targets, while the NC was additionally influenced by the nitrogen-doping ratio. The SSA, TPV and MP peaked around the activation temperature of 800 degrees C and the impregnation ratio of 3, while NC also increased with the amount of nitrogen doping and reached saturation of nitrogen fixation at the nitrogen-doped ratio of 3. This datadriven scientific tool effectively guided the synthesis of porous carbon and broadened the utilization of biomass energy.
更多查看译文
关键词
Agricultural waste,Porous carbon,Nitrogen-doped,Machine learning
AI 理解论文
溯源树
样例
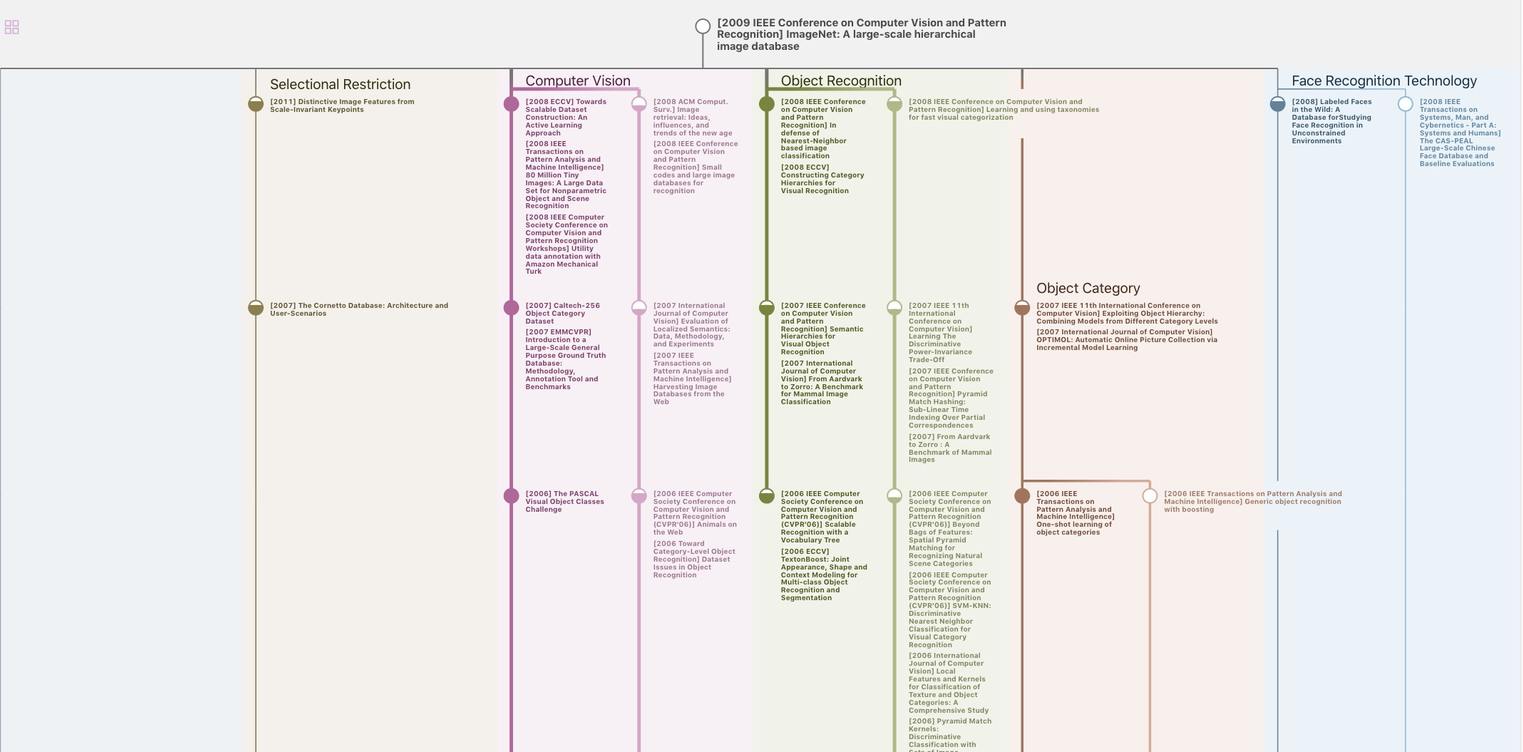
生成溯源树,研究论文发展脉络
Chat Paper
正在生成论文摘要