A learning and potential area-mining evolutionary algorithm for large-scale multi-objective optimization
EXPERT SYSTEMS WITH APPLICATIONS(2024)
摘要
For large-scale multi-objective optimization problems, it is not easy for existing multi-objective evolutionary algorithms to search the entire decision variable space with limited computation resources. To alleviate this problem, we propose a learning and potential area-mining evolutionary algorithm to explore and exploit key regions for accelerating optimization. First, we mine promising areas by clustering the population-gathered regions. Then, potential directions are determined by a multi-guiding point scheme in these promising areas. Subsequently, we design a local search and global search scheme to enhance population convergence while ensuring diversity. Finally, a mutation strategy is used to improve diversity. We execute numerical experiments on two widely-used LSMOP benchmarks and compare the proposed algorithm with three state-of-the-art algorithms. The statistical results indicate that the proposed algorithm has significant performance.
更多查看译文
关键词
Area mining,Machine learning,Potential directions,Multi-guiding points,Large-scale optimization,Multi-objective optimization
AI 理解论文
溯源树
样例
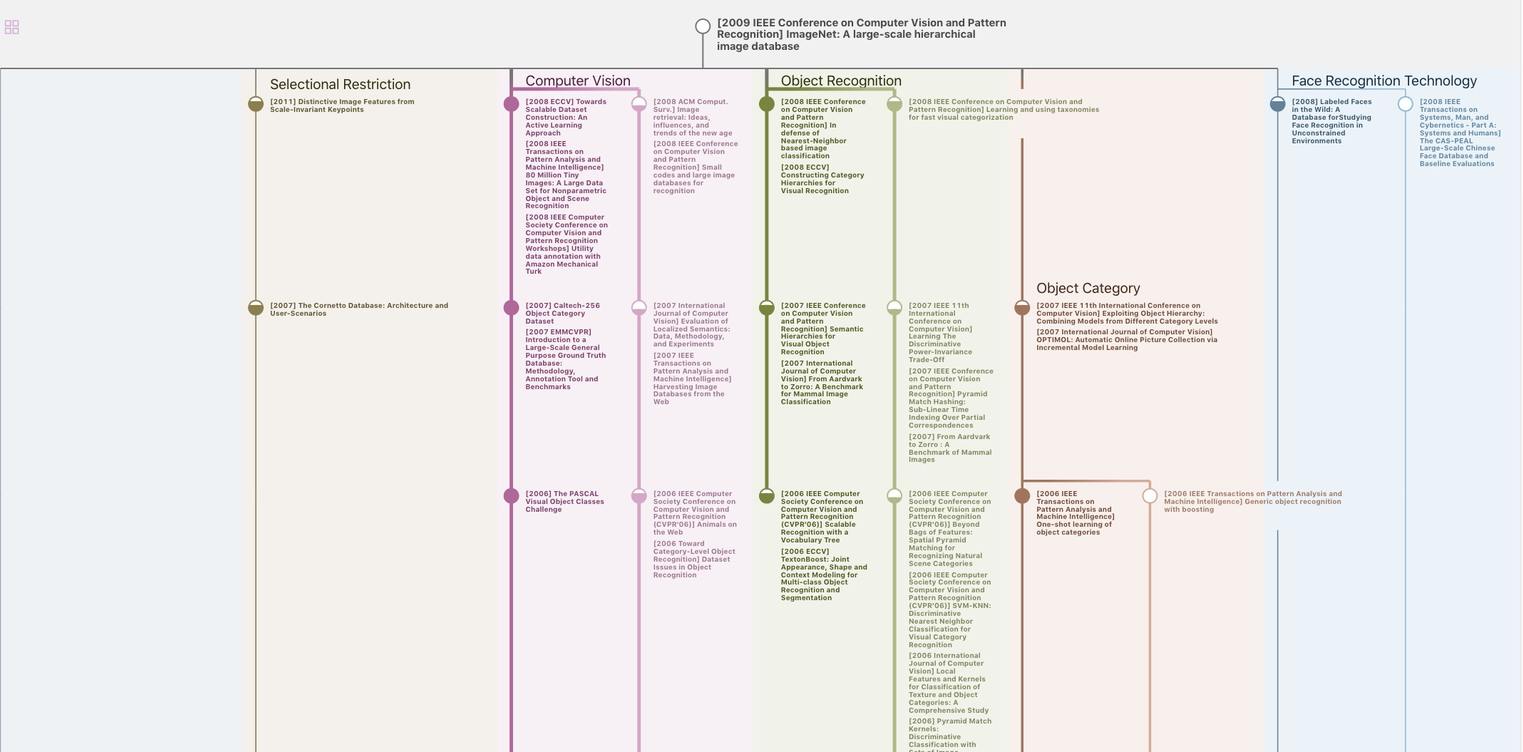
生成溯源树,研究论文发展脉络
Chat Paper
正在生成论文摘要