Reinforcement learning for real-time process control in high-temperature superconductor manufacturing
INTERNATIONAL JOURNAL OF ADVANCED MANUFACTURING TECHNOLOGY(2023)
摘要
With high efficiency and low energy loss, high-temperature superconductors (HTS) have demonstrated their profound applications in various fields, such as medical imaging, transportation, accelerators, microwave devices, and power systems. The high-field applications of HTS tapes have raised the demand for producing cost-effective tapes with long lengths in superconductor manufacturing. However, achieving the uniform and enhanced performance of a long HTS tape is challenging due to the unstable growth conditions in the manufacturing process. Although it is confirmed that the process parameters during the advanced metal organic chemical vapor deposition (A-MOCVD) process influence the uniformity of the produced HTS tapes, the high-dimensional process parameter signals and their complicated interactions make it difficult to develop an effective control policy. In this paper, we propose a local measure for the uniformity of HTS tapes to provide instant feedback for our control policy. Then, we model the manufacturing of HTS tapes as a Markov decision process (MDP) with continuous state and action spaces to assess the instant reward in real time in our feedback control model. As our MDP involves continuous and high-dimensional state and action spaces, a neural fitted Q-iteration (NFQ) algorithm is adopted to solve the MDP with artificial neural network (ANN) function approximation. The collinearity of process parameters can restrict our capability of adjusting the process parameters, which is addressed by the principal component analysis (PCA) in our method. The control policy adjusts the PCA of process parameters using the NFQ algorithm. Based on our case studies on real A-MOCVD dataset, the obtained control policy increases the average uniformity of tapes by 5.6% and performs especially well on sample HTS tapes with a low uniformity.
更多查看译文
关键词
High-temperature superconductor,Reinforcement learning,Function approximation,Artificial neural network,Process control
AI 理解论文
溯源树
样例
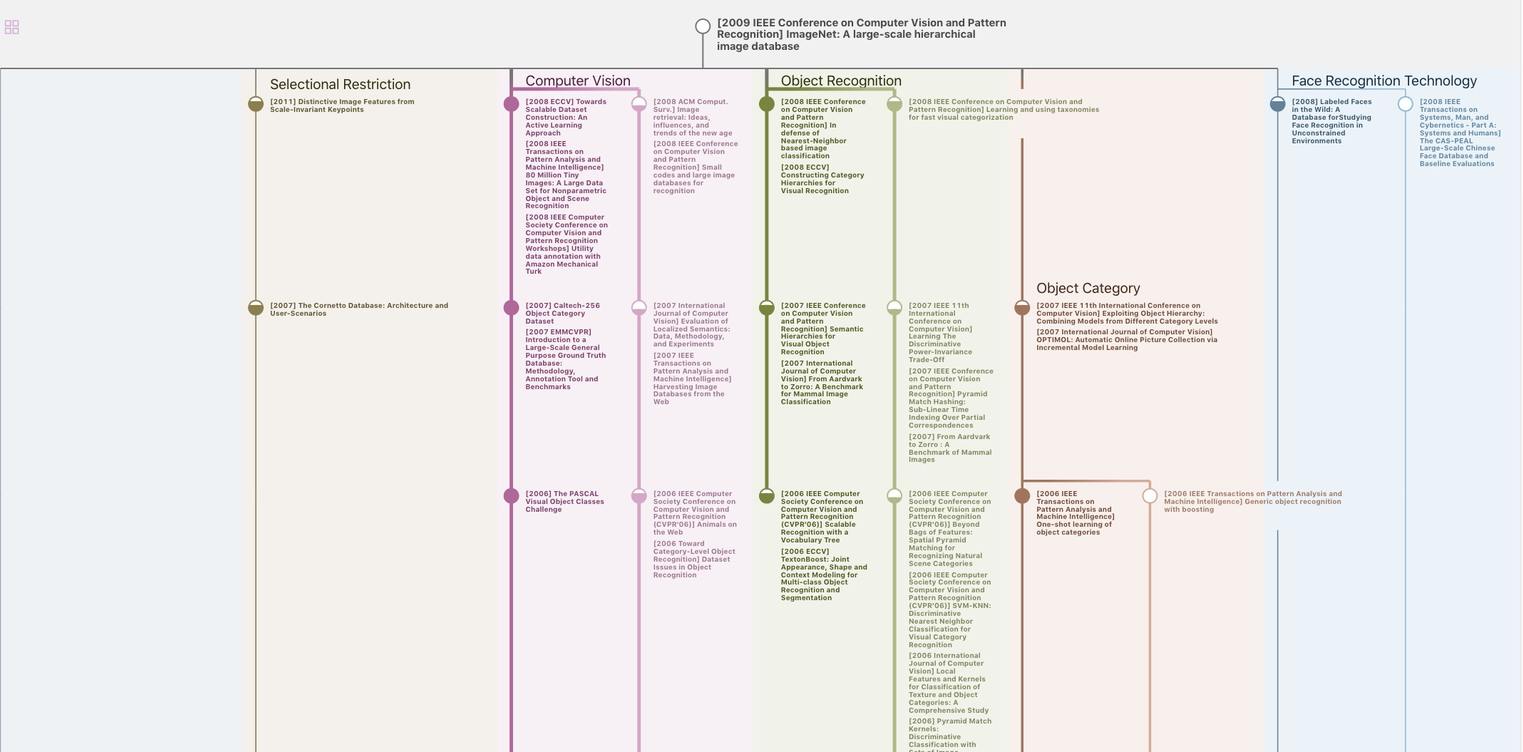
生成溯源树,研究论文发展脉络
Chat Paper
正在生成论文摘要