Explainable FinTech lending
JOURNAL OF ECONOMICS AND BUSINESS(2023)
摘要
Lending activities, especially for small and medium enterprises (SMEs), are increasingly based on financial technologies, facilitated by the availability of advanced machine learning (ML) methods that can accurately predict the financial performance of a company from the available data sources. However, despite their high predictive accuracy, ML models may not give users sufficient interpretation of the results. Therefore, it may not be adequate for informed decision-making, as stated, for example, in the recently proposed artificial intelligence (AI) regulations. To fill the gap, we employed Shapley values in the context of model selection. Thus, we propose a model selection method based on predictive accuracy that can be employed for all types of ML models, those with a probabilistic background, as in the current state-of-the-art. We applied our proposal to a credit-scoring database with more than 100,000 SMEs. The empirical findings indicate that the risk of investing in a specific SME can be predicted and interpreted well using a machinelearning model which is both predictively accurate and explainable.
更多查看译文
关键词
Fintech, Credit scoring, Artificial intelligence, Machine learning, Shapley values
AI 理解论文
溯源树
样例
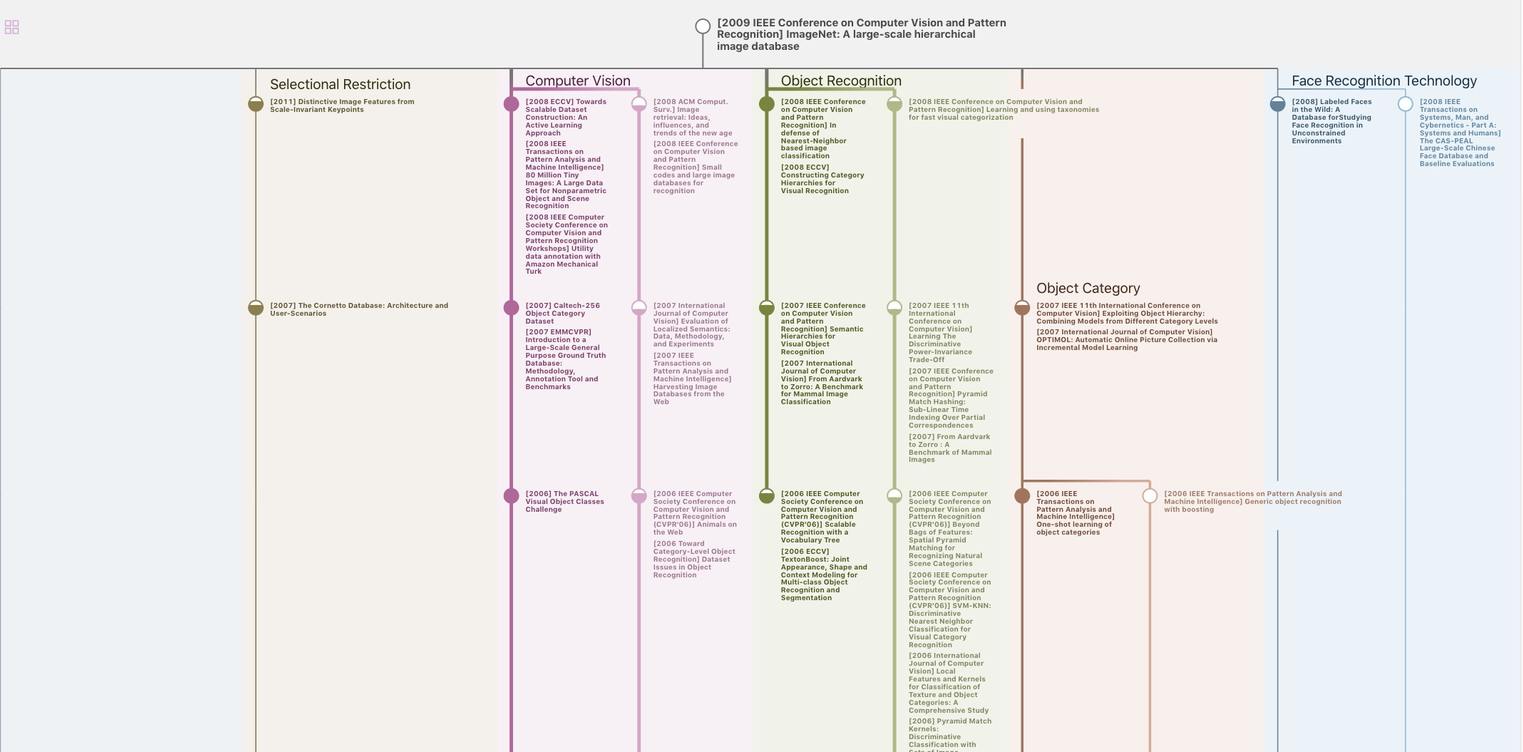
生成溯源树,研究论文发展脉络
Chat Paper
正在生成论文摘要