PICGAN: Conditional adversarial neural network-based permittivity inversions for ground penetrating radar data
JOURNAL OF APPLIED GEOPHYSICS(2023)
摘要
A network called Permittivity Inversions Conditional Generative Adversarial Network (PICGAN) has been proposed to address the challenge of mapping ground penetrating radar (GPR) B-scan data to permittivity maps of subsurface structures. The PICGAN comprises a generator and a discriminator. The generator uses an EncoderDecoder network to extract low-dimensional features from the GPR B-scan while performing high-dimensional permittivity distribution mapping. The discriminator is designed with convolutional and pooling networks. The consistency of the corresponding relation between the input data is enhanced by simultaneously feeding both undetermined permittivity and real data to the discriminator. Meanwhile, the advantages of Markovian discriminator (PatchGAN) and gradient penalty Wasserstein GAN (WGAN-GP) are combined in PICGAN to propose a novel loss function with a regularization term and gradient penalty Wasserstein distance. The results of actual and numerical simulation experiments reveal that the PICGAN network is capable of reconstructing underground targets with clearer boundaries. Comparative results with Encoder-Decoder network and PatchGAN network also demonstrate the superiority of the PICGAN. The results also show that the PICGAN network can effectively reconstruct the position and permittivity of underground targets using GPR B-scan data with lower permittivity inversion errors.
更多查看译文
关键词
Ground penetrating radar,Deep neural networks,Permittivity inversion,CGAN,Wasserstein GAN,PICGAN
AI 理解论文
溯源树
样例
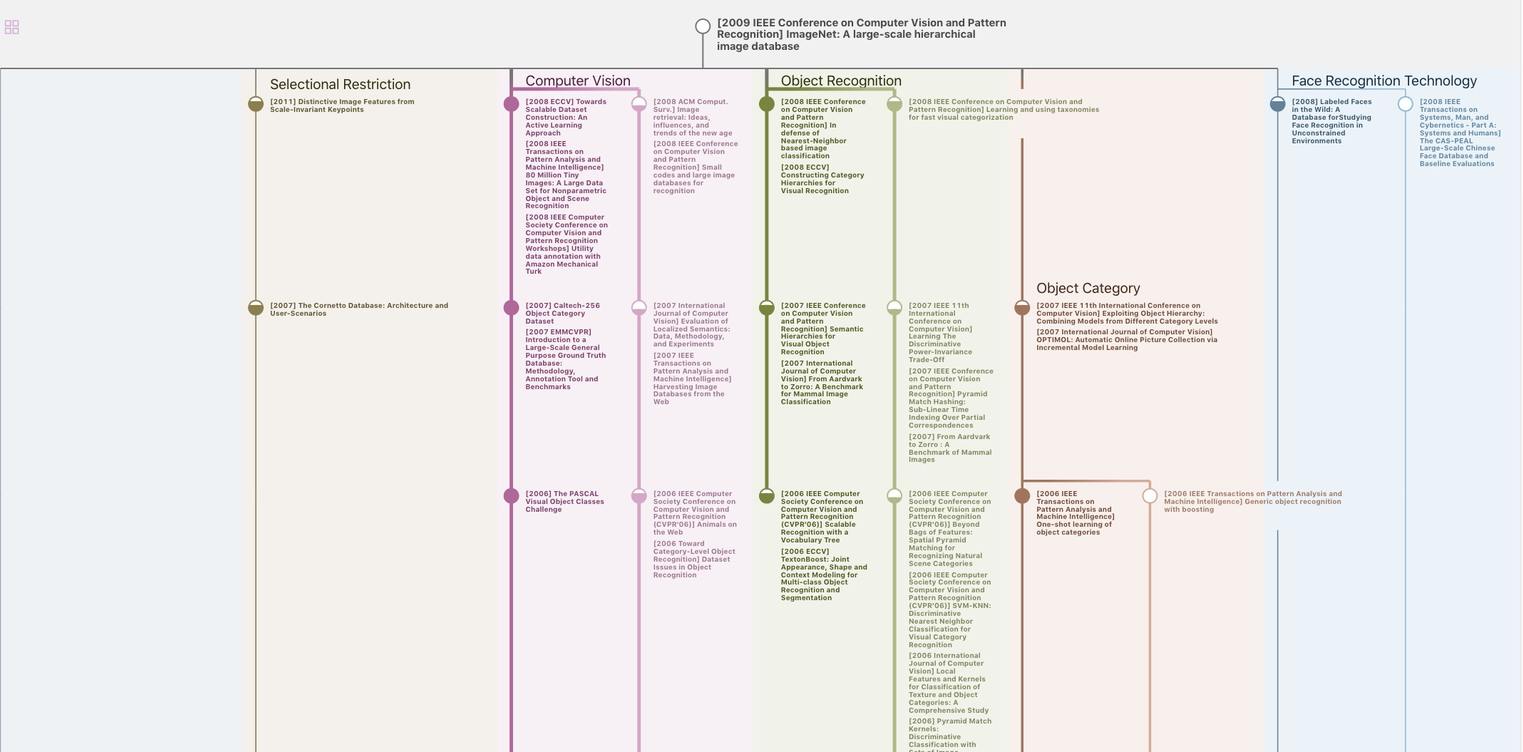
生成溯源树,研究论文发展脉络
Chat Paper
正在生成论文摘要