Identifying heart disease risk factors from electronic health records using an ensemble of deep learning method
IISE TRANSACTIONS ON HEALTHCARE SYSTEMS ENGINEERING(2023)
摘要
Heart disease is a leading cause of death worldwide. For decades, cardiologists have attempted to identify heart-disease risk factors to facilitate its prediction, prevention, and treatment. In recent years, electronic health records (EHRs) have become a valuable source for detecting these risk factors (e.g. smoking, obesity, and diabetes). However, challenges persist as EHRs include clinical notes in free-form and unstructured text, making it tedious for cardiologists to retrieve relevant information. To resolve this problem, we devised a deep-learning-based ensemble approach to automatically identify heart-disease risk factors from EHRs. This proposed approach can efficiently extract semantic information from EHRs and automate risk-factor identification with high performance. In particular, this approach does not require any external domain knowledge about the disease because a powerful Bidirectional Encoder Representations from Transformers (BERT) method is implemented to encode the discriminative features of clinical notes. The extracted features are then fed to conditional random fields (CRF) to identify all possible risk-factor indicators. Experimental results show that, in a scenario where no external knowledge is available, the proposed approach achieves state-of-the-art performance.
更多查看译文
关键词
Deep learning,natural language processing,electronic health record,heart disease,risk factors
AI 理解论文
溯源树
样例
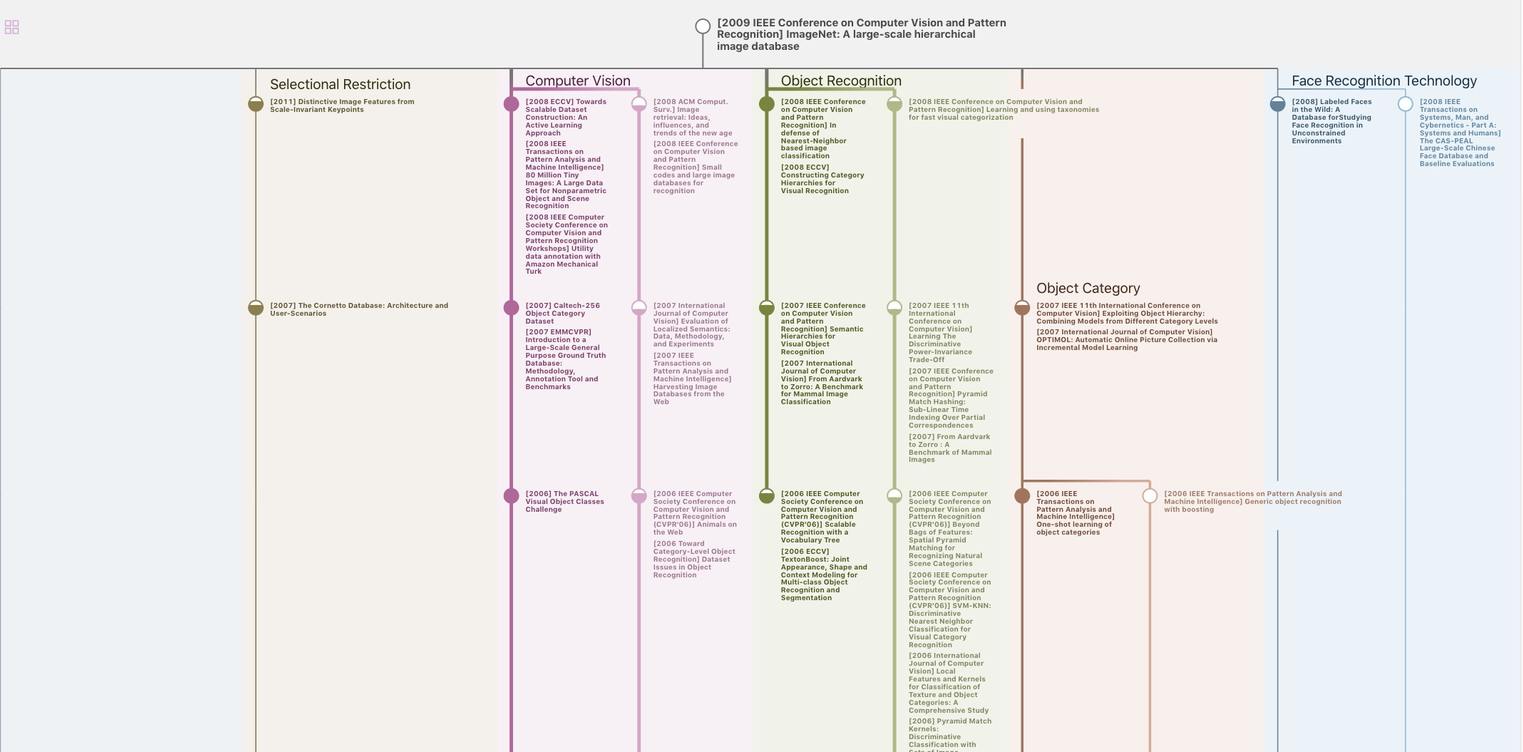
生成溯源树,研究论文发展脉络
Chat Paper
正在生成论文摘要