Safety-critical control for autonomous underwater vehicles with unknown disturbance using function approximator
OCEAN ENGINEERING(2023)
摘要
Safety and stability are two primary factors that affect the task execution performance of autonomous underwater vehicles (AUVs), especially when they operate in a disturbed environment. The unknown disturbances and unmodeled dynamics will severely degrade the performance, resulting in unsafe and unstable behaviors. This paper presents a safety-critical control framework for AUVs in the presence of unknown disturbances using a neural network function approximator. The high-order control barrier functions (CBFs) are utilized to address the safe constraints of relative degree two. A nominal controller is designed in which the unknown lumped disturbances are compensated by a radial basis function neural network, thus ensuring that the tracking errors are uniformly ultimately bounded. Besides, the function approximation and the associated bounded approximation errors are adopted to estimate the unknown lumped disturbance terms in the second-order derivative of CBFs, which alleviates the mismatch of the forward invariance condition for the safety set. The minimally invasive quadratic program (QP) is formulated to make minimal sacrifice on the performance of nominal controller. The proposed method encompasses a straightforward structure for disturbance estimation, alongside a highly efficient explicit estimating rule that promotes rapid convergence. Integrated with computationally efficient QP, the proposed method demonstrates substantial promise for real-time implementation. Comparative numerical results with disturbance observer and input-to-state safety-based robust methods reveal an improved trade-off between safety and control performance.
更多查看译文
关键词
Autonomous underwater vehicle,Control barrier functions,Safety-critical control,Set invariance,Neural network compensation
AI 理解论文
溯源树
样例
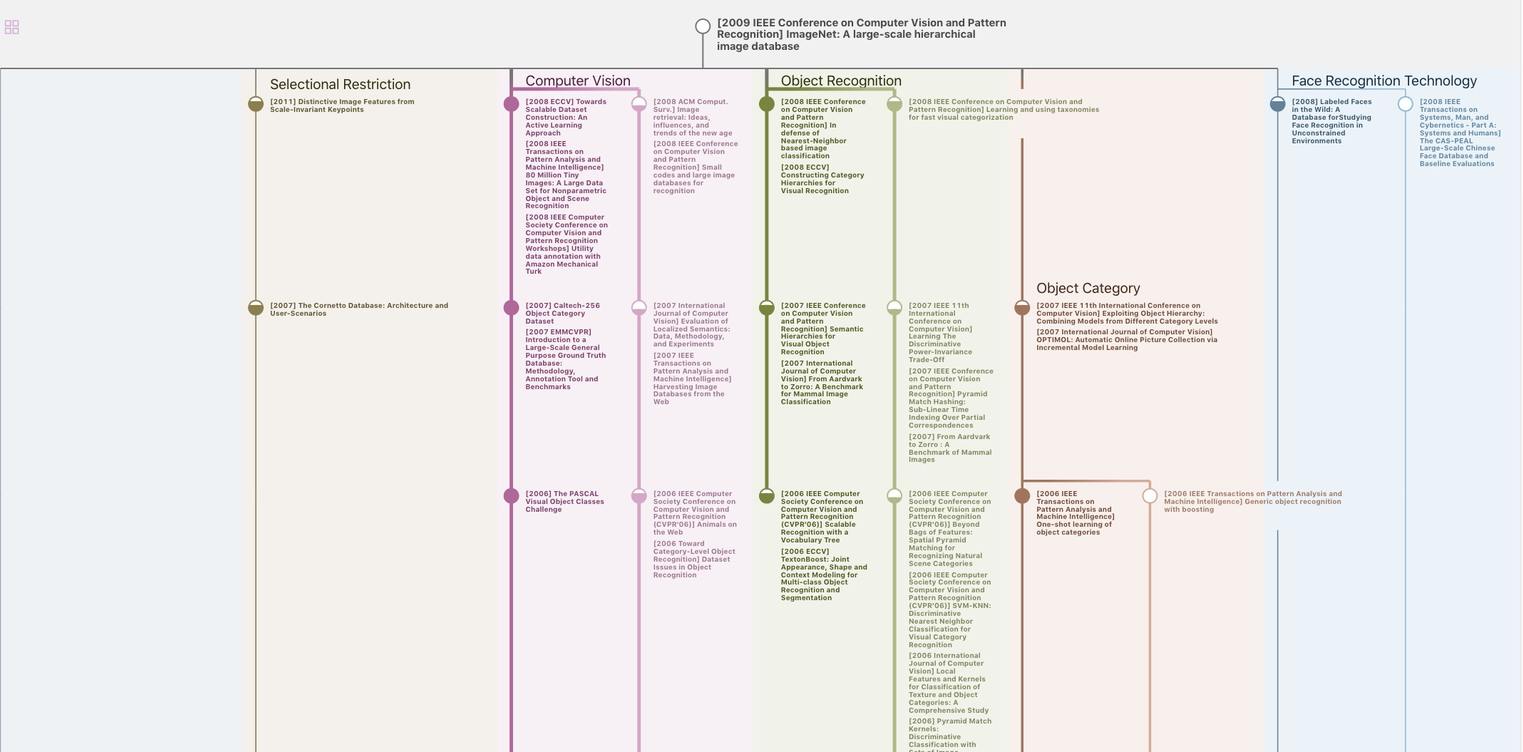
生成溯源树,研究论文发展脉络
Chat Paper
正在生成论文摘要