Temporal-Aware Neural Network for Dense Non-Rigid Structure from Motion
ELECTRONICS(2023)
摘要
Modern neural networks addressing dense Non-Rigid Structure from Motion (NRSFM) dilemmas often grapple with intricate a priori constraints, deterring scalability, or overlook the imperative of consistent application of a priori knowledge throughout the entire input sequence. In this paper, an innovative neural network architecture is introduced. Initially, the complete 2D sequence image undergoes embedding into a low-dimensional space. Subsequently, multiple self-attention layers are employed to extract inter-frame features, with the objective of deriving a more continuous and temporally smooth low-dimensional structure closely resembling real data's intrinsic structure. Moreover, it has been demonstrated by others that gradient descent during the training of multilayer linear networks yields minimum rank solutions, implicitly providing regularization that is equally applicable to this task. Benefiting from the excellence of the proposed network architecture, no additional a priori knowledge is mandated, barring the constraint of temporal smoothness. Extensive experimentation confirms the method's exceptional performance in addressing dense NRSFM challenges, outperforming recent results across various dense benchmark datasets.
更多查看译文
关键词
neural network,structure,temporal-aware,non-rigid
AI 理解论文
溯源树
样例
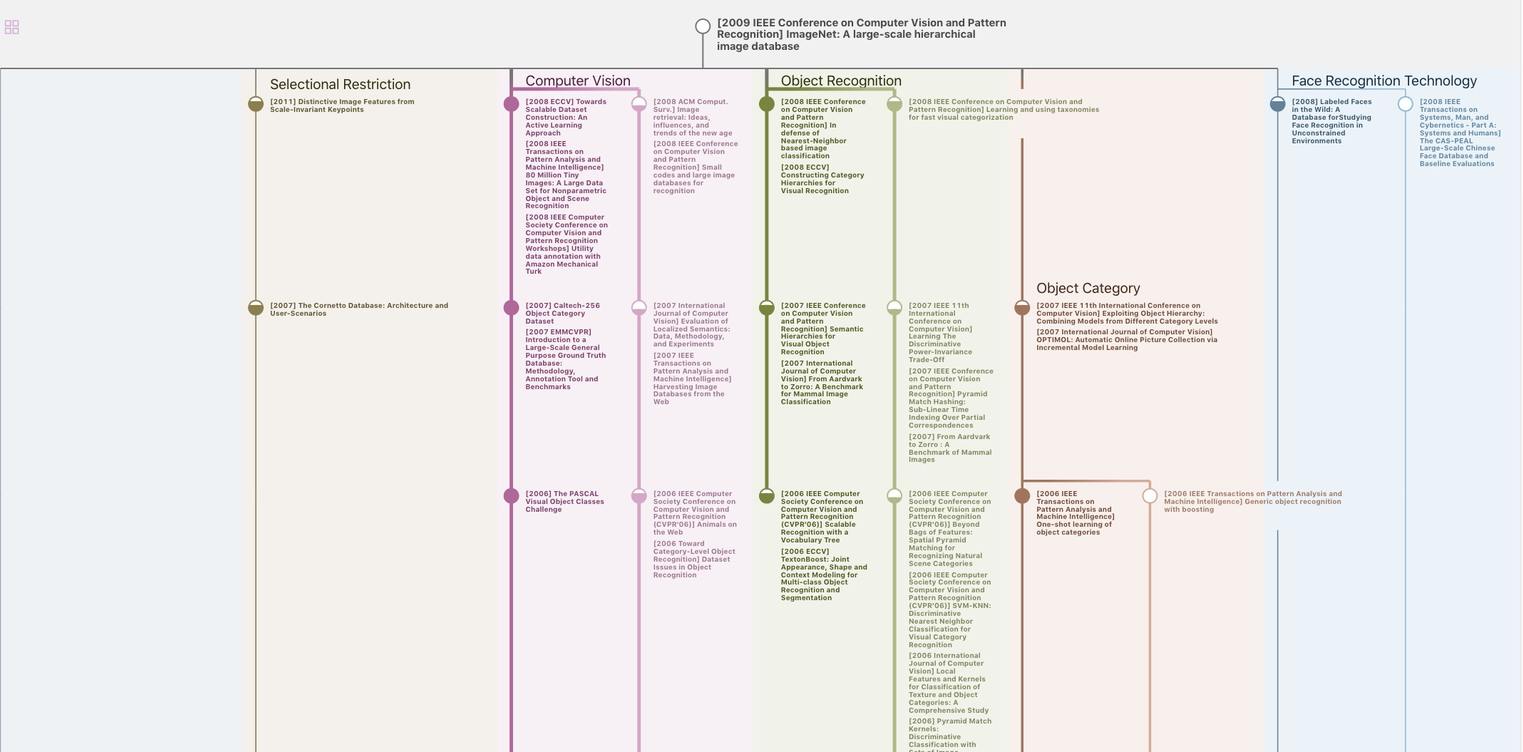
生成溯源树,研究论文发展脉络
Chat Paper
正在生成论文摘要