Predicting Consumer Service Price Evolution during the COVID-19 Pandemic: An Optimized Machine Learning Approach
ELECTRONICS(2023)
摘要
This research analyzes the impact of the COVID-19 pandemic on consumer service pricing within the European Union, focusing on the Transportation, Accommodation, and Food Service sectors. Our study employs various machine learning models, including multilayer perceptron, XGBoost, CatBoost, and random forest, along with genetic algorithms for comprehensive hyperparameter tuning and price evolution forecasting. We incorporate coronavirus cases and deaths as factors to enhance prediction accuracy. The dataset comprises monthly reports of COVID-19 cases and deaths, alongside managerial survey responses regarding company estimations. Applying genetic algorithms for hyperparameter optimization across all models results in significant enhancements, yielding optimized models that exhibit RMSE score reductions ranging from 3.35% to 5.67%. Additionally, the study demonstrates that XGBoost yields more accurate predictions, achieving an RMSE score of 17.07.
更多查看译文
关键词
consumer service price evolution,pandemic,optimized machine learning approach,machine learning approach,machine learning
AI 理解论文
溯源树
样例
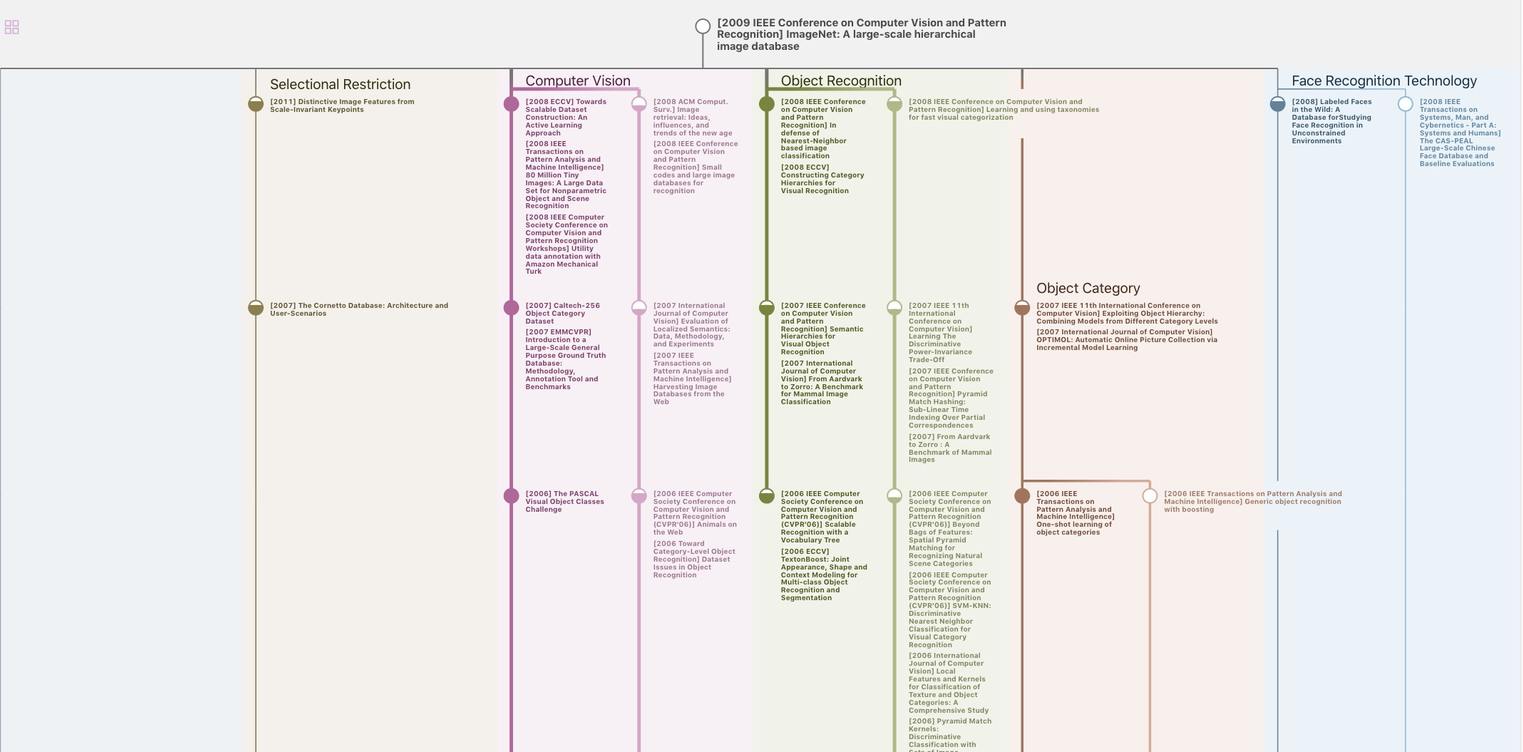
生成溯源树,研究论文发展脉络
Chat Paper
正在生成论文摘要