Transfer Learning Dehazing Network by Gaussian Process Mapping
SIGNAL PROCESSING-IMAGE COMMUNICATION(2023)
摘要
Haze seriously interferes with the high-quality imaging process, resulting in a significant difference from a haze-free scene to a hazy status. This phenomenon makes dehazing challenging. To make real data dehazing effective, we propose a novel Transfer Learning Dehazing Network (TLDN) with Gaussian Process Mapping (GPM) for the single image dehazing in this paper. Under our observation, we believe there is a correlation between hazy and haze-free scenes in the middle hidden channels. Therefore, we suppose a transfer functional relationship in some latent features encoded by two kinds of networks and embedded into a latent vector space. The two encoder–decoder networks are introduced to learn the corresponding high-dimensional feature representation between pairs of hazy and haze-free images. These features would be employed to build a mapping relationship under the Gaussian process regression. Finally, experimental results on synthetic data and real-world samples demonstrate the effectiveness of our proposed method.
更多查看译文
关键词
Image dehazing,Gaussian process,Transfer learning
AI 理解论文
溯源树
样例
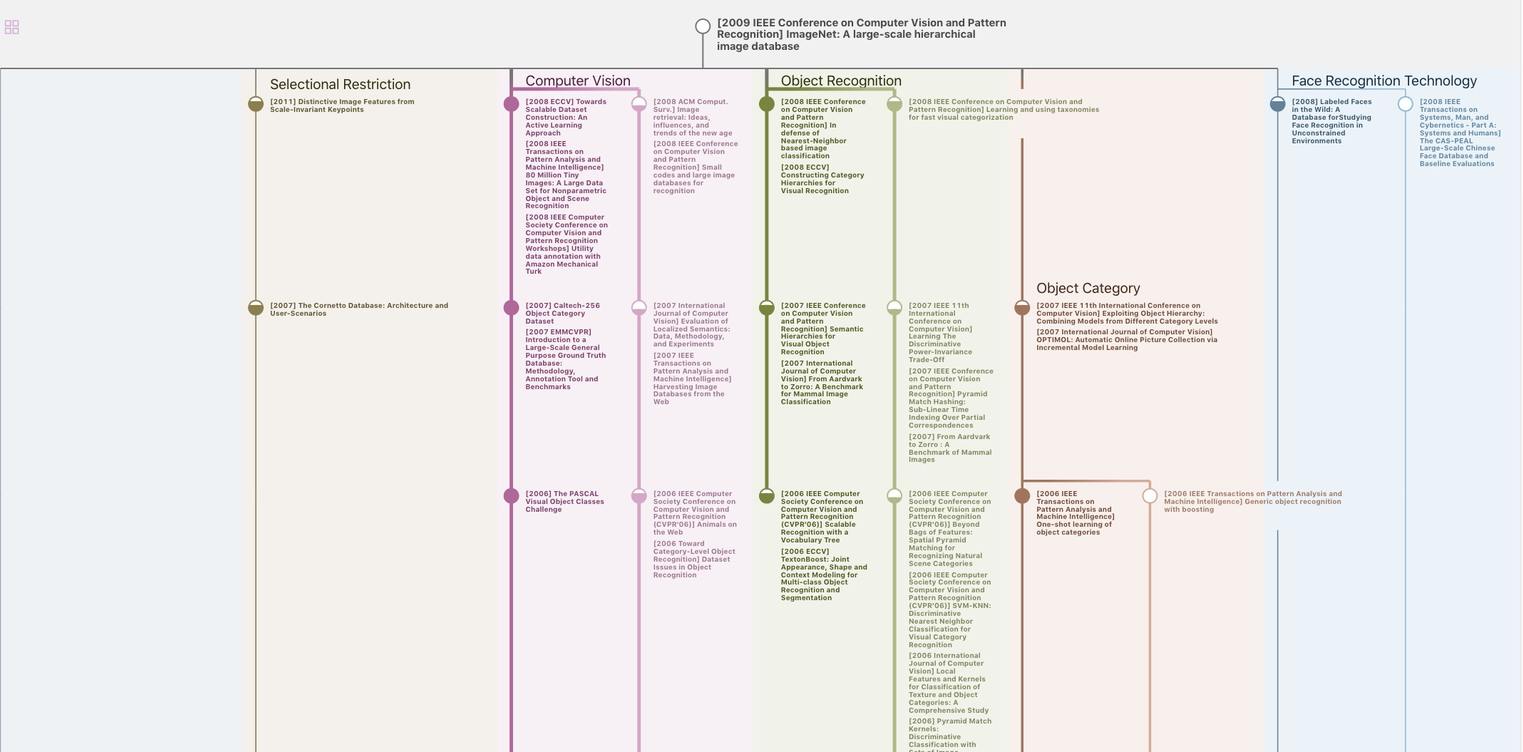
生成溯源树,研究论文发展脉络
Chat Paper
正在生成论文摘要