Developing a Breast Cancer Resistance Protein Substrate Prediction System Using Deep Features and LDA
CMC-COMPUTERS MATERIALS & CONTINUA(2023)
摘要
Breast cancer resistance protein (BCRP) is an important resistance protein that significantly impacts anticancer drug discovery, treatment, and rehabilitation. Early identification of BCRP substrates is quite a challenging task. This study aims to predict early substrate structure, which can help to optimize anticancer drug development and clinical diagnosis. For this study, a novel intelligent approach-based methodology is developed by modifying the ResNet101 model using transfer learning (TL) for automatic deep feature (DF) extraction followed by classification with linear discriminant analysis algorithm (TLRNDF-LDA). This study utilized structural fingerprints, which are exploited by DF contrary to conventional molecular descriptors. The proposed in silico model achieved an outstanding accuracy performance of 98.56% on test data compared to other state-of-the-art approaches using standard quality measures. Furthermore, the model's efficacy is validated via a statistical analysis ANOVA test. It is demonstrated that the developed model can be used effectively for early prediction of the substrate structure. The pipeline of this study is flexible and can be extended for in vitro assessment efficacy of anticancer drug response, identification of BCRP functions in transport experiments, and prediction of prostate or lung cancer cell lines.
更多查看译文
关键词
BCRP,drug response,deep learning,transfer learning,LDA,In silico
AI 理解论文
溯源树
样例
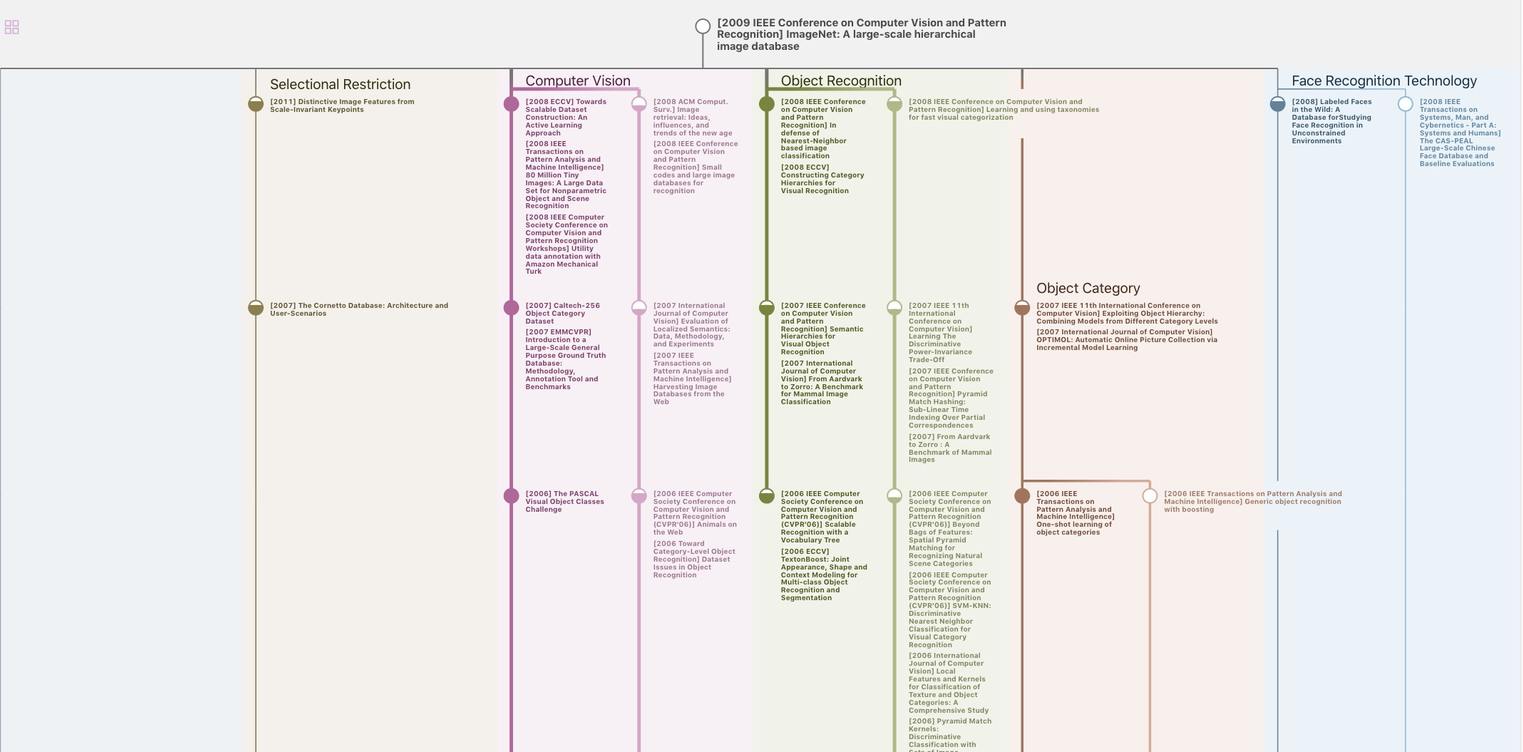
生成溯源树,研究论文发展脉络
Chat Paper
正在生成论文摘要