Synthetic aperture radar ground target image generation based on improved Wasserstein generative adversarial networks with gradient penalty
JOURNAL OF APPLIED REMOTE SENSING(2023)
摘要
The traditional generative adversarial network (GAN) is widely used in the field of synthetic aperture radar (SAR) ground target image generation. However, GAN has the problem of unstable gradient update, which can easily cause the loss of image feature information, resulting in a low similarity between the generated image and the real image. To solve these problems, we propose an improved Wasserstein GAN with gradient penalty (IWGAN-GP), which introduces dense connection in the generator, integrates feature information at different levels to achieve feature reuse, and alleviates the gradient disappearance problem caused by deep networks. Moreover, by introducing the squeeze-and-excitation (SE) module into the densely connected network, on the basis of considering high-level semantic information and low-level geometric texture details, the optimal fusion weights of each channel can be automatically obtained to fully explore important target information in SAR images. IWGAN-GP alleviates the gradient disappearance caused by the depth of the network, strengthens feature propagation, and realizes feature reuse. It can automatically obtain the optimal fusion weight of each channel and improve the similarity between the generated image and the real image. The superiority of IWGAN-GP is verified on the datasets of MSTAR.
更多查看译文
关键词
synthetic aperture radar ground target image generation,improved WGAN with gradient penalty,squeeze-and-excitation module,dense connection,multi-layered optimal feature fusion
AI 理解论文
溯源树
样例
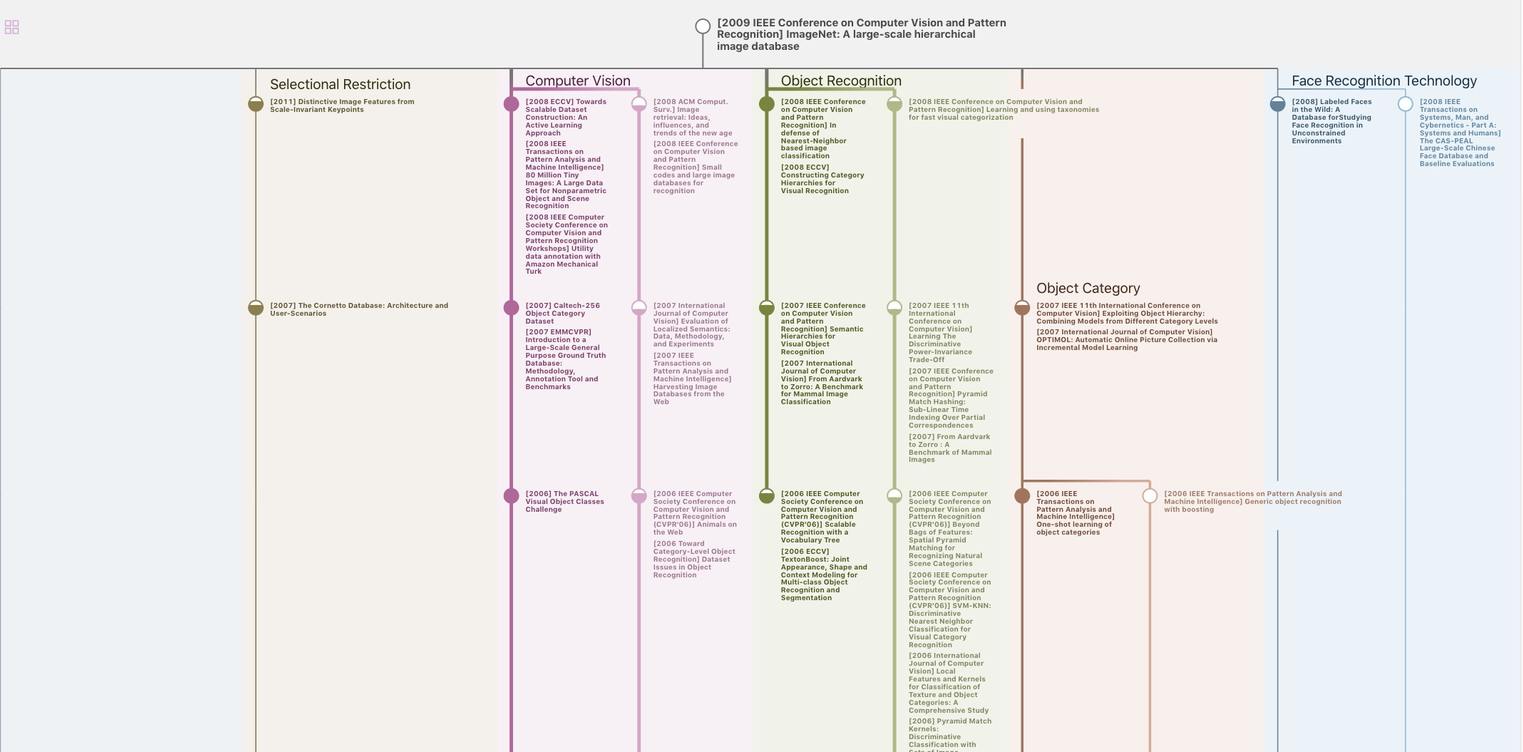
生成溯源树,研究论文发展脉络
Chat Paper
正在生成论文摘要