A mechanics-informed deep learning framework for data-driven nonlinear viscoelasticity
COMPUTER METHODS IN APPLIED MECHANICS AND ENGINEERING(2023)
摘要
A mechanics-informed, data-driven framework for learning the constitutive law of a nonlinear viscoelastic material from stress–strain data using deep artificial neural networks (ANNs) is proposed. In this framework, each ANN architecture is designed and its input and output are selected such that fundamental laws of mechanics and thermodynamics relevant to nonlinear viscoelasticity are strongly satisfied by the resulting data-driven constitutive model. The framework itself extends a previously developed mechanics-informed methodology for the data-driven constitutive modeling of nonlinear elasticity to account for the dependence of the stress on the entire strain history. It is grounded in an additive decomposition of the strain energy density and an internal variable approach to viscoelasticity. Collectively, these are characterized by three potentials whose ANN representations are taught the second law of thermodynamics, various stability conditions, consistency (preservation of rigid body modes), and the recovery of elastic limits. The proposed mechanics-informed deep learning framework for data-driven nonlinear viscoelasticity is illustrated and its performance is assessed for one academic problem where synthetic data is generated by a nonlinear convolution; a coupon-level application associated with a multi-scale homogenization of a viscoelastic woven fabric material; and two representative finite element analyses performed using the material law generated during the previous application, to highlight different features of the framework.
更多查看译文
关键词
Constitutive modeling,Data-driven,Physics-informed,Thermodynamics,Viscoelasticity,Neural network
AI 理解论文
溯源树
样例
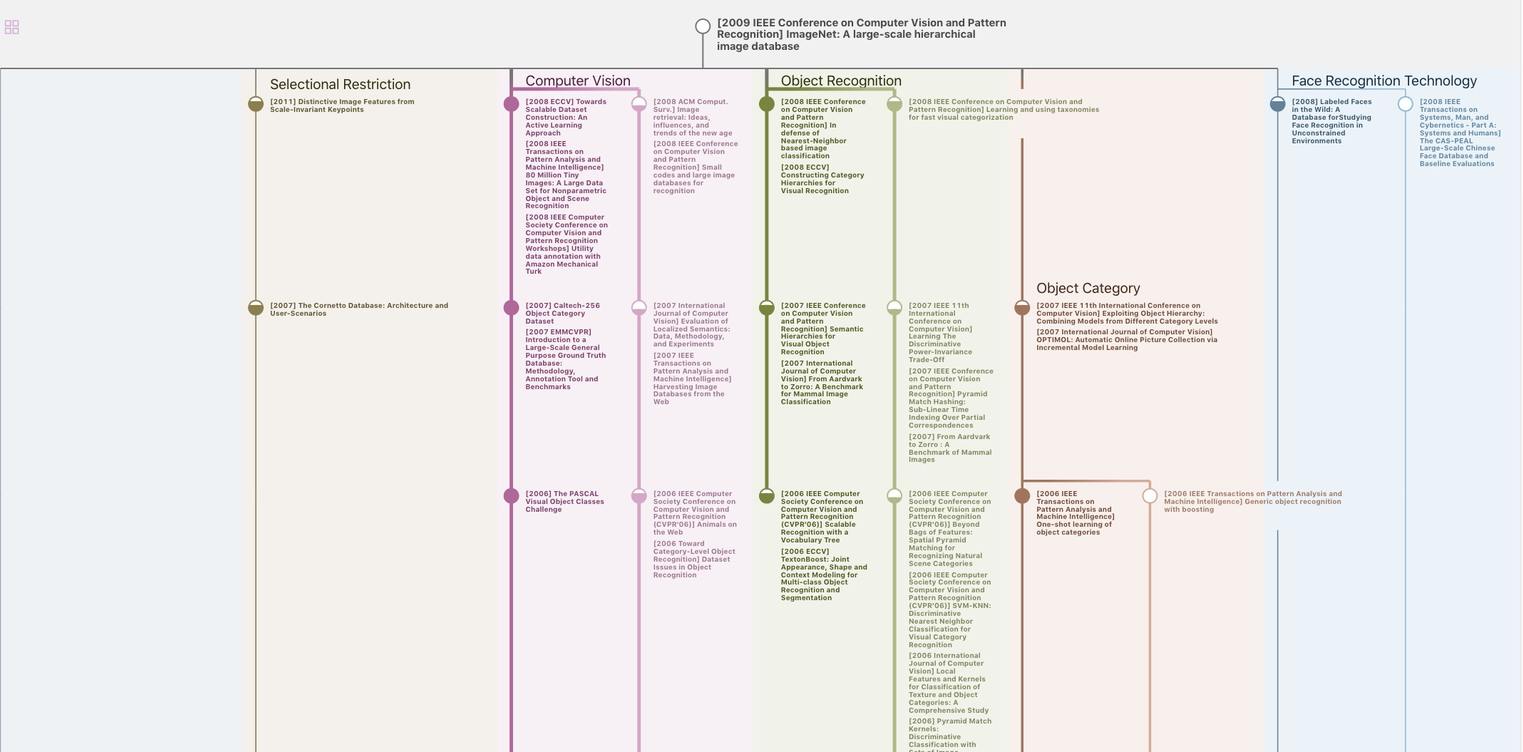
生成溯源树,研究论文发展脉络
Chat Paper
正在生成论文摘要