Unified Representation Learning for Multi-View Clustering by Between/Within View Deep Majorization
IEEE TRANSACTIONS ON EMERGING TOPICS IN COMPUTATIONAL INTELLIGENCE(2024)
摘要
Multi-view data are characterized by a variety of features that are semantically coherent, with different views usually complementing each other. Multi-view clustering seeks to learn a unified representation that is comprehensive yet discriminative to partition the samples. However, existing research ignored the possible redundancy of latent feature in each view, then yielded a unified task-oriented feature based on the correlation of these multi-view latent features without explicit consideration of the influence of the unified feature. Such a way failed to accurately measure the association among views. To fully take the association into account, we propose a deep multi-view Unified Representation Learning network, named by deep-URL, using measurement between multi-view data to realize desirable unified representation. Specifically, deep-URL employs a multi-view encoder-decoder framework that maps multi-scale data into a same latent space to obtain view-specific latent representation. Within each view, the view-specific features are pruned to eliminate the redundant information in the latent space by minimizing the within-view mutual information. Between the multiple views, a unified informative representation is derived to accurately estimate the nonlinear associations between views via maximizing conditional mutual information of the view-specific features. The deep unified representation is further elaborated to achieve the full reconstruction of each view to ensure its reliability. The proposed network is trained by between/within view deep representation majorization. It achieves redundant representation removal within each view, unified features integration across views, and universal reconstruction via decoding. Extensive experiments on five real datasets with largely varied sample sizes demonstrate that the deep-URL achieved superior clustering performance by comparing with fourteen baseline methods.
更多查看译文
关键词
Multi-view clustering,multi-view learning,unsupervised learning,conditional mutual information
AI 理解论文
溯源树
样例
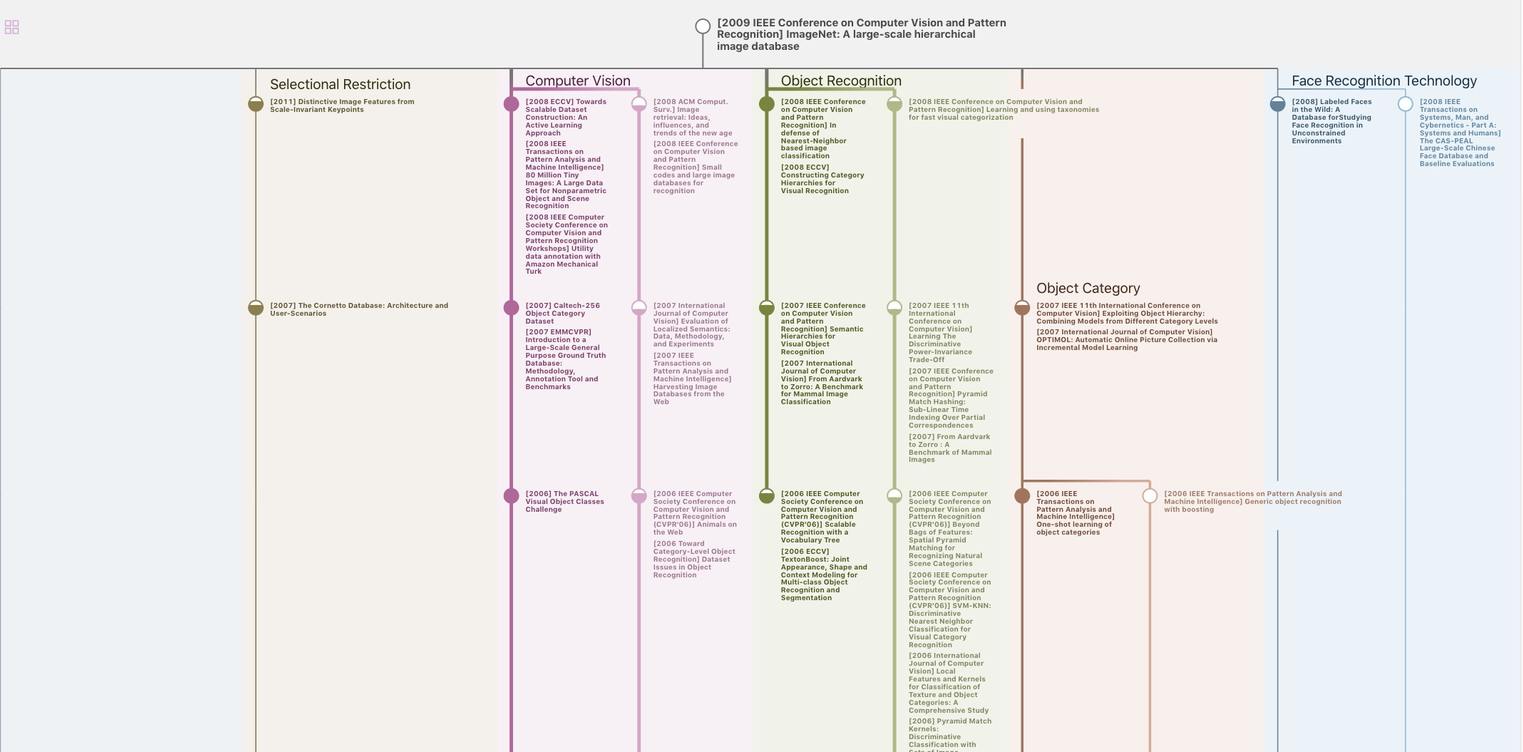
生成溯源树,研究论文发展脉络
Chat Paper
正在生成论文摘要