Boolean matrix factorization for symmetric binary variables
KNOWLEDGE-BASED SYSTEMS(2023)
摘要
Binary variables classify into two types: asymmetric variables, where one state (1 or 0) is significantly more valuable than the other, and symmetric variables, where both states are equally valuable. Boolean matrix factorization (BMF), a popular methodology of preprocessing and analyzing tabular binary data, handles its input as asymmetric variables. In the paper, we develop an alternative that handles Boolean matrices as symmetric variables. Our method differs from traditional BMF in that the factors are linearly ordered by priority, and factors can contradict each other, meaning that one factor can assign a value of 1 while the other assigns a value of 0. In such a case, the factor with higher priority is the relevant one. Through experiments, we demonstrate that our approach provides a more compact data description than a straightforward application of the traditional BMF methods. Moreover, it is even able to overcome the Schein rank. (c) 2023 Elsevier B.V. All rights reserved.
更多查看译文
关键词
Decomposition,Boolean data,Symmetric attribute,Formal concept
AI 理解论文
溯源树
样例
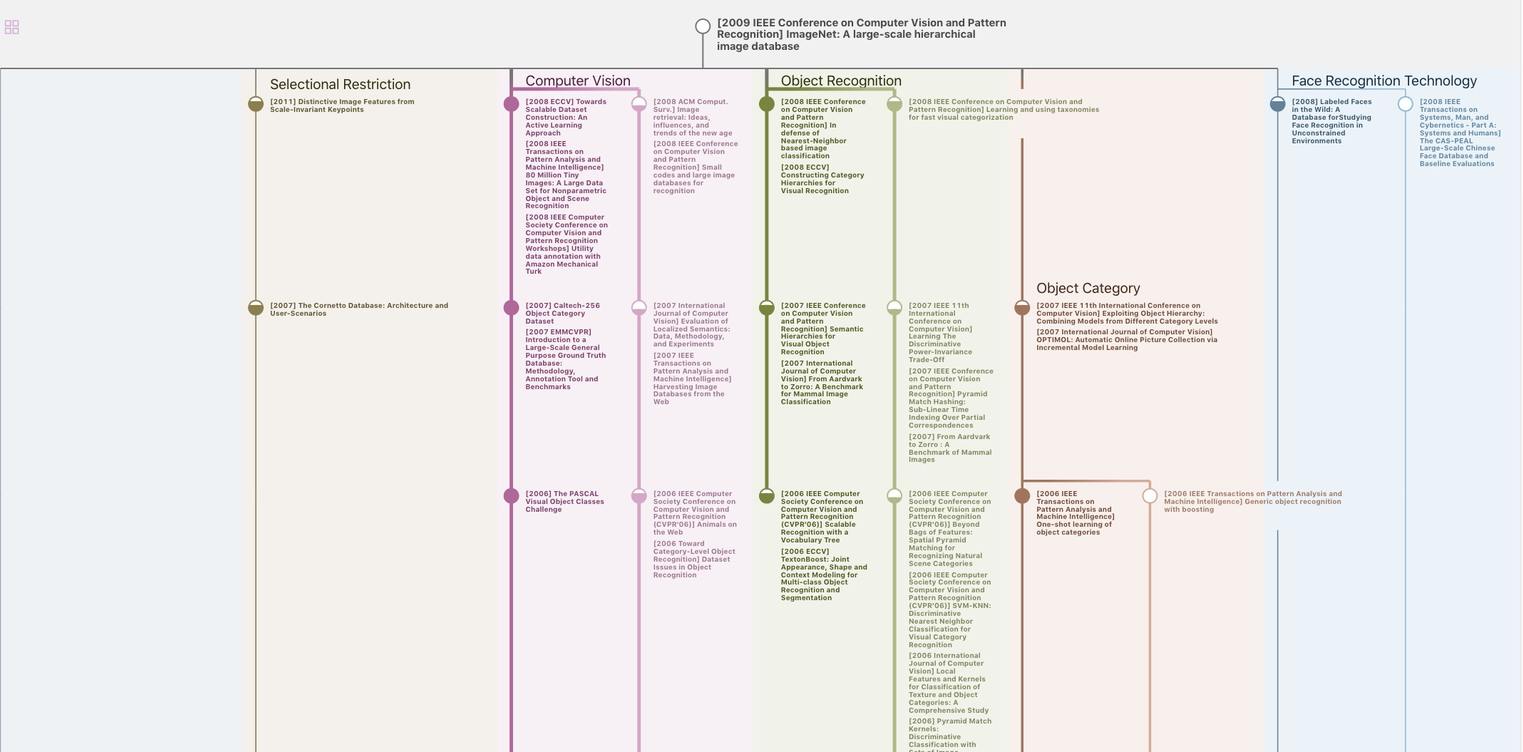
生成溯源树,研究论文发展脉络
Chat Paper
正在生成论文摘要