A graph neural network framework for spatial geodemographic classification
INTERNATIONAL JOURNAL OF GEOGRAPHICAL INFORMATION SCIENCE(2023)
摘要
Geodemographic classifications are exceptional tools for geographic analysis, business and policy-making, providing an overview of the socio-demographic structure of a region by creating an unsupervised, bottom-up classification of its areas based on a large set of variables. Classic approaches can require time-consuming preprocessing of input variables and are frequently a-spatial processes. In this study, we present a groundbreaking, systematic investigation of the use of graph neural networks for spatial geodemographic classification. Using Greater London as a case study, we compare a range of graph autoencoder designs with the official London Output Area Classification and baseline classifications developed using spatial fuzzy c-means. The results show that our framework based on a Node Attributes-focused Graph AutoEncoder (NAGAE) can perform similarly to classic approaches on class homogeneity metrics while providing higher spatial clustering. We conclude by discussing the current limitations of the proposed framework and its potential to develop into a new paradigm for creating a range of geodemographic classifications, from simple, local ones to complex classifications able to incorporate a range of spatial relationships into the process.
更多查看译文
关键词
spatial geodemographic classification,neural network framework
AI 理解论文
溯源树
样例
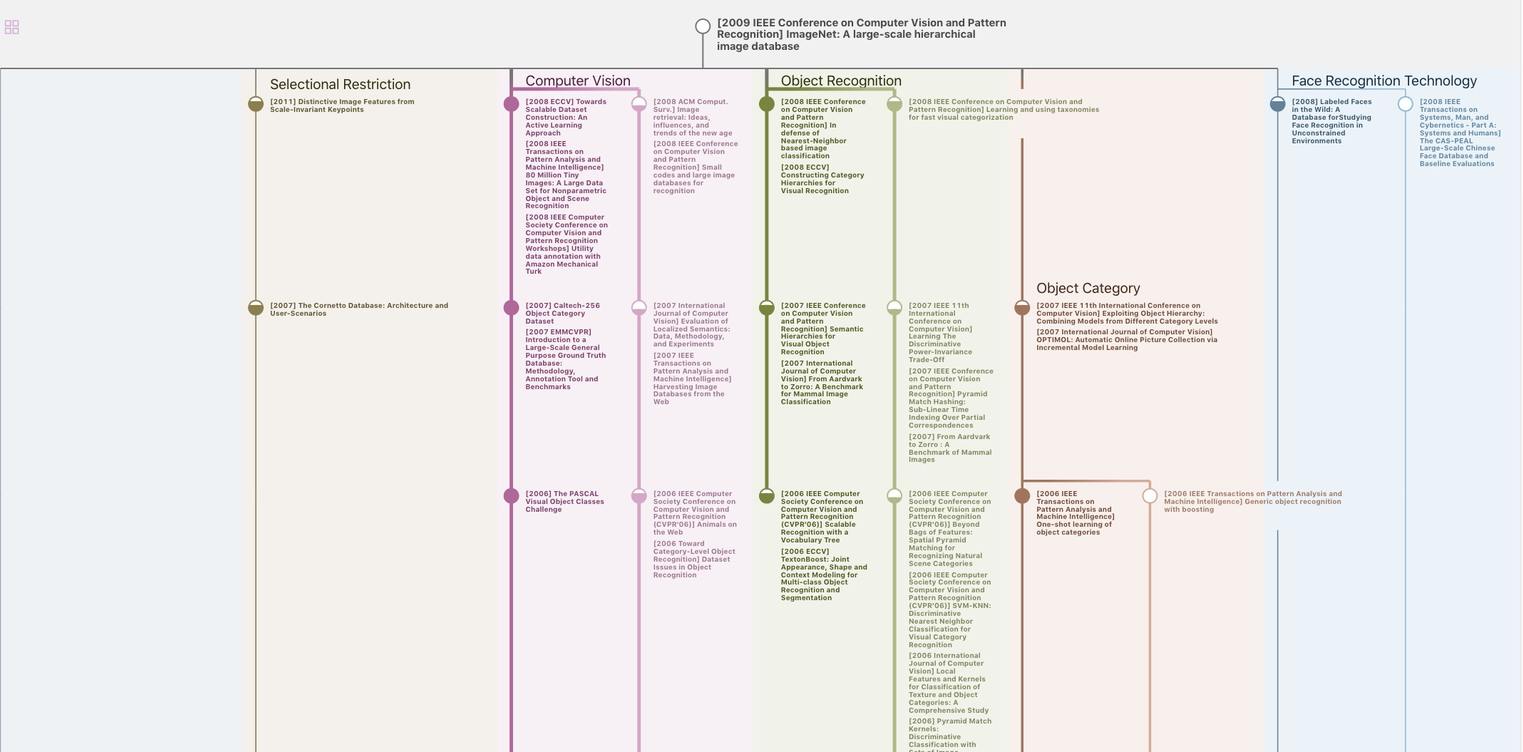
生成溯源树,研究论文发展脉络
Chat Paper
正在生成论文摘要