No Recipe for Quantitative Systems Pharmacology Model Validation, but a Balancing Act Between Risk and Cost
CLINICAL PHARMACOLOGY & THERAPEUTICS(2024)
摘要
Quantitative systems pharmacology (QSP) models generate predictions by formalizing biological understanding and integrating multiscale data, leading to beneficial extrapolation abilities, but may require careful balancing of the model's scope with prediction uncertainties. A workshop at the 2022 American Conference of Pharmacometrics (QSP modelers and conventional pharmacometricians meet at the nexus of late-stage clinical development: expanding the scope of MID3, Concurrent Session 3C, The Thirteenth American Conference on Pharmacometrics (ACoP13), October 30 to November 2, 2022, Aurora, Colorado) brought together practitioners from QSP and pharmacometrics with the aim to develop better understandings and leverage learnings between the two disciplines. The current perspective is based on the concepts that were discussed at the workshop. The significant potential impact of model-informed drug development (MIDD) on cost and efficiency of late-stage pharmaceutical R&D was already reported nearly two decades ago.1 However, despite adoption across industry and regulatory agencies, the attrition during earlier stages of drug development and discovery remains high, with phase II success rates running well below 30% for most portfolios.2 This issue was recognized by the US National Institutes of Health, who organized two workshops in response, which brought together leaders from academia, industry, and government in the fields of systems biology and pharmacology/pharmacometrics. The white paper that arose from this initiative can be considered one of the early milestones that defined quantitative systems pharmacology (QSP) as a subdiscipline within MIDD as “an approach to translational medicine that combines computational and experimental methods to elucidate, validate, and apply new pharmacological concepts to the development and use of small molecule and biologic drugs”.3 Thus, it can be argued that a main objective of QSP (at least from an industry perspective) was to contribute to the improvement of phase II success. Recent examples suggest that this has indeed been achievable.2 The focus on early development, where typically there is a high degree of biological uncertainty (in particular for novel therapeutic approaches or mechanisms of action), implies that a key objective of QSP is to extrapolate to untested clinical scenarios, such as phase II outcomes in the first cohort of patients based on (limited) preclinical and healthy volunteer data. This often results in QSP models being associated with a significant degree of “uncertainty” and explains why it may not be suitable to directly apply well-established validation approaches from pharmacometrics (PMx) to QSP. The former usually relies on the availability of rich data sets, and the model validation focuses on statistical evaluation of how well the model captures the existing data, not on its ability to extrapolate and predict untested scenarios. The difference between PMx and QSP lies in the nature of the questions they aim to address and the available data for answering them. Proper parameter estimation and model validation contribute to the robustness of models and can guide future experimental design by highlighting areas where more data might be needed. However, this is challenging for QSP models due to the lack of comprehensive data sets and the need for cross-validation with diverse sources of information. A simple way to put it is: “When the answer to the question is within available clinical data sets, use PMx. When the answer to the question is spread across multiple data types, use QSP.” In this regard, QSP and PMx should be considered complementary rather than competing approaches within the wider MIDD context, and hybrid approaches could be a way to leverage the benefits of the two methodologies. Some key differences between PMx and QSP models and their uses in drug development are summarized in Table 1. Understanding how QSP is different from PMx and why this may lead to different validation approaches is important, in particular when being included in regulatory submissions. Relatively parsimonious models describing (population) pharmacokinetics and exposure–response relationship from well-controlled clinical trials for a given drug Virtual population represents variability (although in practice often confused with uncertainty) QSP models have been incorporated in applications of the US Food and Drug Administration (FDA) for some time now. A recent survey of communications to the FDA between 2013 and 2020 revealed that 157 of them mentioned QSP, with almost 60 of those submissions in 2020.4 Between 2017 and 2019 the submissions increased from 20 to almost 40 per year. In these years, the FDA has also engaged in two workshops, including a 2019 ASCPT preconference workshop and an FDA–Industry scientific exchange in 2020.5 This is consistent with the rapidly growing application of MIDD approaches in both preclinical and clinical stages. Of the 157 communications containing QSP, 7 had played a central role in addressing regulatory requirements of safety concerns. Three of those seven requested clinical waivers of a study based on QSP. The other four predicted short or long-term safety in lieu of conducting clinical studies. There are also situations in which QSP was acknowledged, but ultimately, other data were sufficient to be relied on for the approval or dosing decisions. Interestingly, QSP appears to have a presence in nearly all therapeutic areas and phases of clinical development. The overarching experience with quantitative modeling and PMx approaches has led the agency to propose adopting a risk-based credibility framework for deciding what verification and validation tasks may be expected for a range of quantitative applications depending on context of use and the risk to patients if a wrong decision was made (originally derived in the context of physiologically-based pharmacokinetics modeling).6 A couple of recent examples highlight the varied nature of impact of QSP in regulatory decisions and their associated validation approaches. The FDA asked for sensitivity analyses to gain additional confidence in the small systems model predictions of hypoglycemia for a rapid-insulin lispro product in patients with either type 1 or type 2 diabetes mellitus.7 The applicant varied carbohydrate intake and pre-meal vs. post-meal dose administration. Results were supportive for safety (characterizing hypoglycemia) expectations when it was not evaluated for post meal administration in the pivotal phase III trial. Additionally, QSP was recently utilized to establish similarity in disease progression between pediatric and adult patients for olipudase alfa in patients with acid sphingomyelinase deficiency.8 Along with a number of validation steps, parameter estimates were compared between children and adults to support the comparison of disease progression in each population. In another example, reasonable prediction of the COVID vaccine response was used as an external validation for the larger systems model to predict immunogenicity and vaccine response. These QSP models have been applied to support various drug development and approval steps (Table 2). Throughout history, various validation approaches have been attempted in PMx development. While the term “validation” has never been precisely defined, in principle, it should reduce uncertainty in model applications. In PMx, uncertainty is often confused with variability, in practice although not in concept.9 In QSP, the confusion seems even more prevalent, and “uncertainty” in QSP model predictions often includes both variability and uncertainty. Clearly, the interpretation will affect the intended model use, and further clarifications are needed in both PMx and QSP. Despite the controversies surrounding PMx models, well-established evaluation standards exist for them. However, PMx model “validation” has been essentially reduced to nonspecific model diagnostics.10 Nowadays, models are usually accepted without validation being questioned, likely due to familiarity instead of sound scientific rationale. The current attention on validation in QSP seems to be retracing the PMx development path. Perhaps this will abate when QSP models become more familiar, if history repeats itself. Nevertheless, model credibility analysis and validation are a critical step in ensuring confidence in the predictions made by models before they can be used to support clinical development, especially in regulatory submissions. The extent of validation should always be fit-for-purpose and tailored to the associated decision risk level and should not exceed what is necessary (by providing confidence commensurate to the risk level). Complete confidence in a model's predictions is neither possible nor desirable. And, as such, it is important to strike a balance between the level of confidence in the predictions and the cost and time involved in developing and validating the models. If the incremental increase in confidence comes at a high cost, it may be counterproductive and may not align with the desired level of de-risking it aims to achieve. There is often the impression that, because QSP models can be complex, they should be regarded with higher scrutiny and distrust. But it is important to emphasize that confidence requirements for regulatory submissions should all be risk-based and should not depend on the type of method used, whether it is QSP modeling, PMx, or clinical analysis, because the relationship between confidence and risk is the same. However, while the path to achieve the required level of confidence may differ widely between methods, the overall level of confidence for QSP models should not be greater or lesser compared to other approaches. Three examples of model credibility assessment covering a wide spectrum of PMx and QSP models are summarized in Table 2, showing how models with different goals may require customized validation approaches. In summary, proper QSP model validation is vital for expanding its application in drug development as well as ensuring regulatory compliance. Though QSP models differ substantially in their scope, approach, and intended uses from other models, a QSP model validation strategy should be fit-for-purpose and tailored to the associated decision risks. The few case examples above have shown the broad range of possible ways to assess QSP credibility and validation to serve the regulatory needs. While a consensus on standard QSP model assessment methods may not be feasible in the short term, more awareness is needed on the appropriate and systematic use of fit-for-purpose and practical QSP model validation methods. The authors would like to thank Justin C. Earp for his valuable input and assistance in the writing of this manuscript. No funding was received for this work. The authors declared no competing interests for this work. As Editor-in-Chief of Clinical Pharmacology & Therapeutics, Piet H. van der Graaf was not involved in the review or decision process for this paper.
更多查看译文
AI 理解论文
溯源树
样例
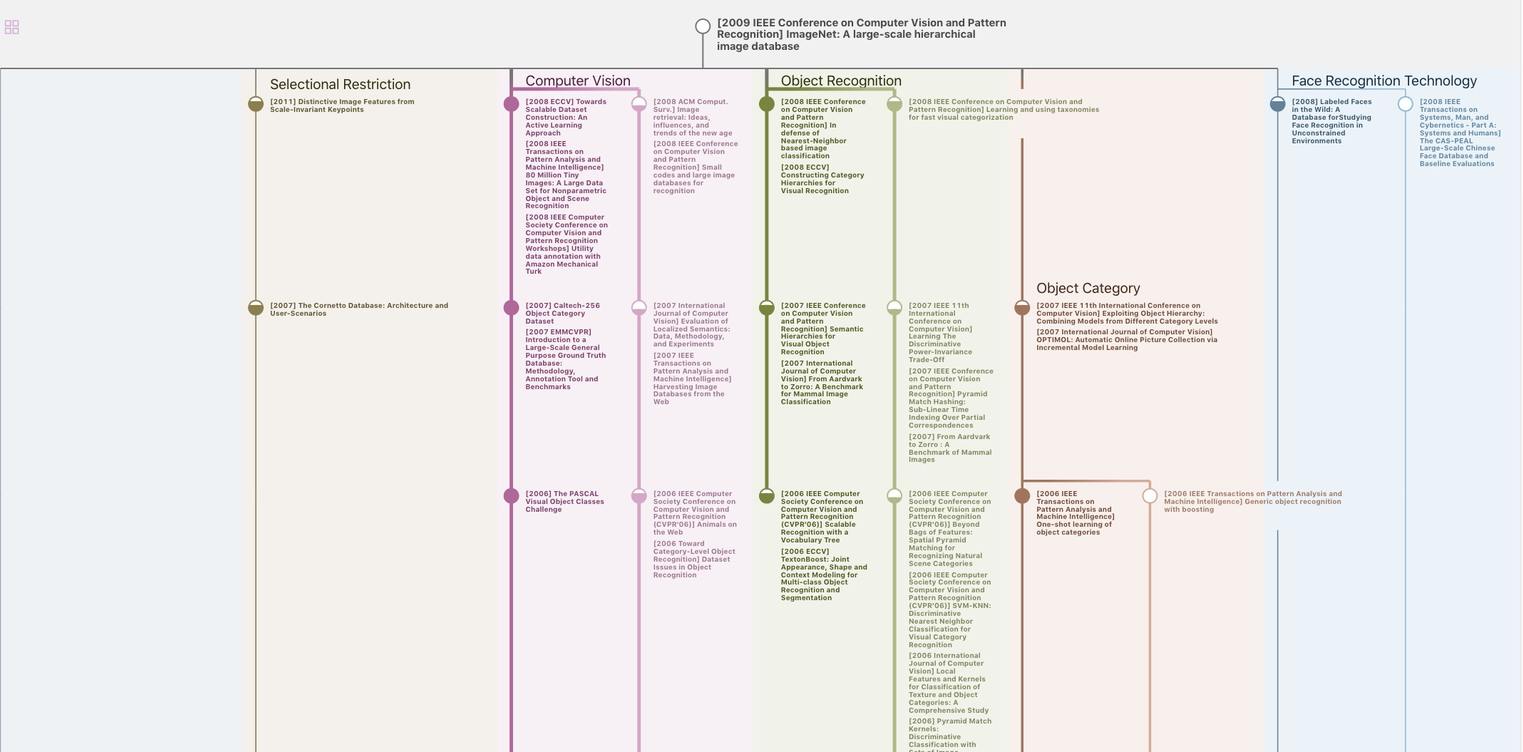
生成溯源树,研究论文发展脉络
Chat Paper
正在生成论文摘要