Accelerating accurate simulations of calorimeter showers with normalizing flows and probability density distillation
PHYSICAL REVIEW D(2023)
摘要
Recently, we introduced CaloFlow, a high-fidelity generative model for GEANT4 calorimeter shower emulation based on normalizing flows. Here, we present CaloFlow v2, an improvement on our original framework that speeds up shower generation by a further factor of 500 relative to the original. The improvement is based on a technique called probability density distillation, originally developed for speech synthesis in the machine learning literature, and which we develop further by introducing a set of powerful new loss terms. We demonstrate that CaloFlow v2 preserves the same high fidelity as the original using qualitative (average images, histograms of high-level features) and quantitative (classifier metric between GEANT4 and generated samples) measures. The result is a generative model for calorimeter showers that matches the state of the art in speed (a factor of 104 faster than GEANT4) and greatly surpasses the previous state of the art in fidelity.
更多查看译文
关键词
calorimeter showers,normalizing flows,accurate simulations
AI 理解论文
溯源树
样例
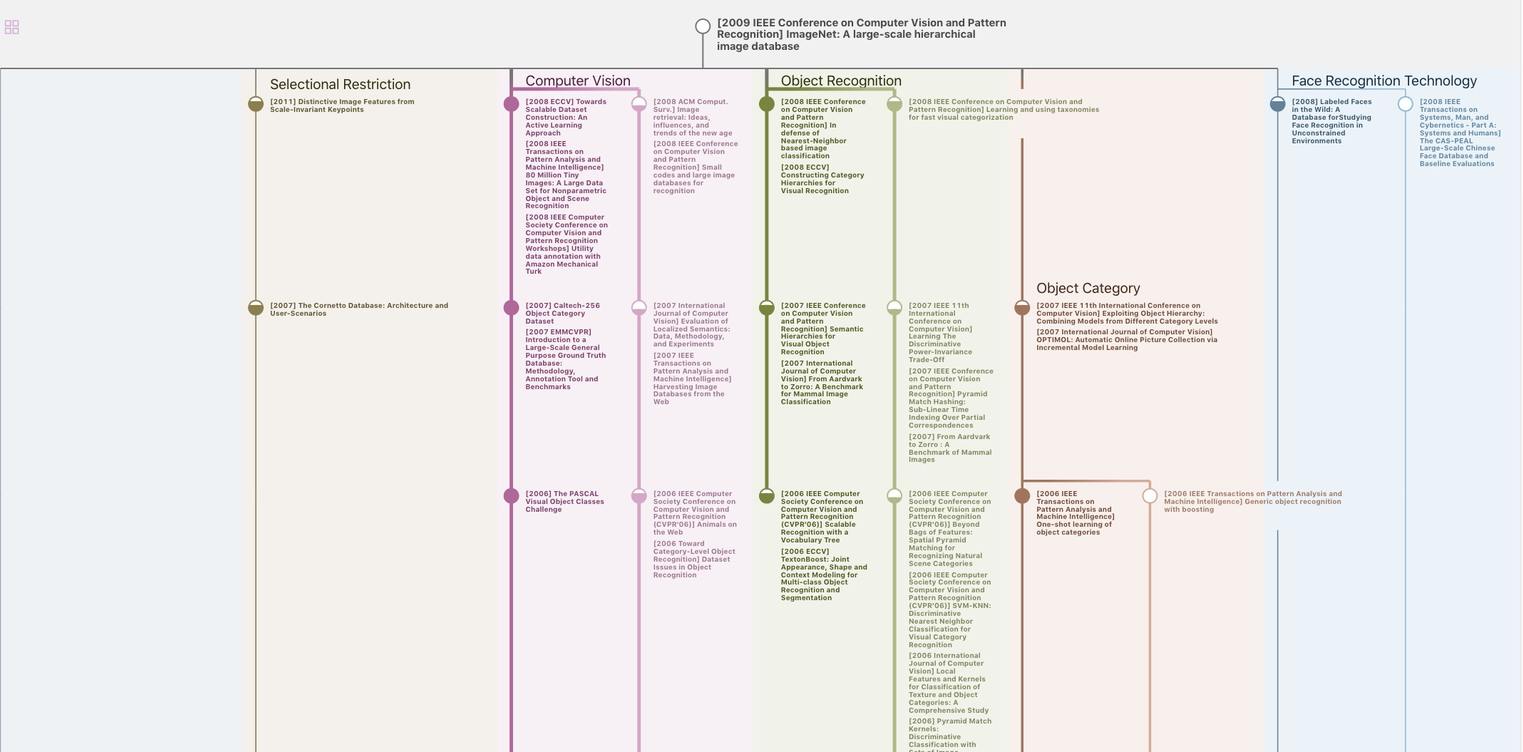
生成溯源树,研究论文发展脉络
Chat Paper
正在生成论文摘要