Correcting Temporal Inaccuracies in Labeled Training Data for Electromyographic Control Algorithms
2023 INTERNATIONAL CONFERENCE ON REHABILITATION ROBOTICS, ICORR(2023)
摘要
Electromyographic (EMG) control relies on supervised-learning algorithms that correlate EMG to motor intent. The quality of the training dataset is critical to the run-time performance of the algorithm, but labeling motor intent is imprecise and imperfect. Traditional EMG training data is collected while participants mimic predetermined movements of a virtual hand with their own hand. This assumes participants are perfectly synchronized with the predetermined movements, which is unlikely due to reaction time and signal-processing delays. Prior work has used cross-correlation to globally shift and re-align kinematic data and EMG. Here, we quantify the impact of this global re-alignment on both classification algorithms and regression algorithms with and without a human in the loop. We also introduce a novel trial-by-trial re-alignment method to re-align EMG with kinematics on a per-movement basis. We show that EMG and kinematic data are inherently misaligned, and that reaction time is inconsistent throughout data collection. Both global and trial-by-trial re-alignment significantly improved offline performance for classification and regression. Our trial-by-trial re-alignment further improved offline classification performance relative to global re-alignment. However, online performance, with a human actively in the loop, was no different with or without re-alignment. This work highlights inaccuracies in labeled EMG data and has broad implications for EMG-control applications.
更多查看译文
AI 理解论文
溯源树
样例
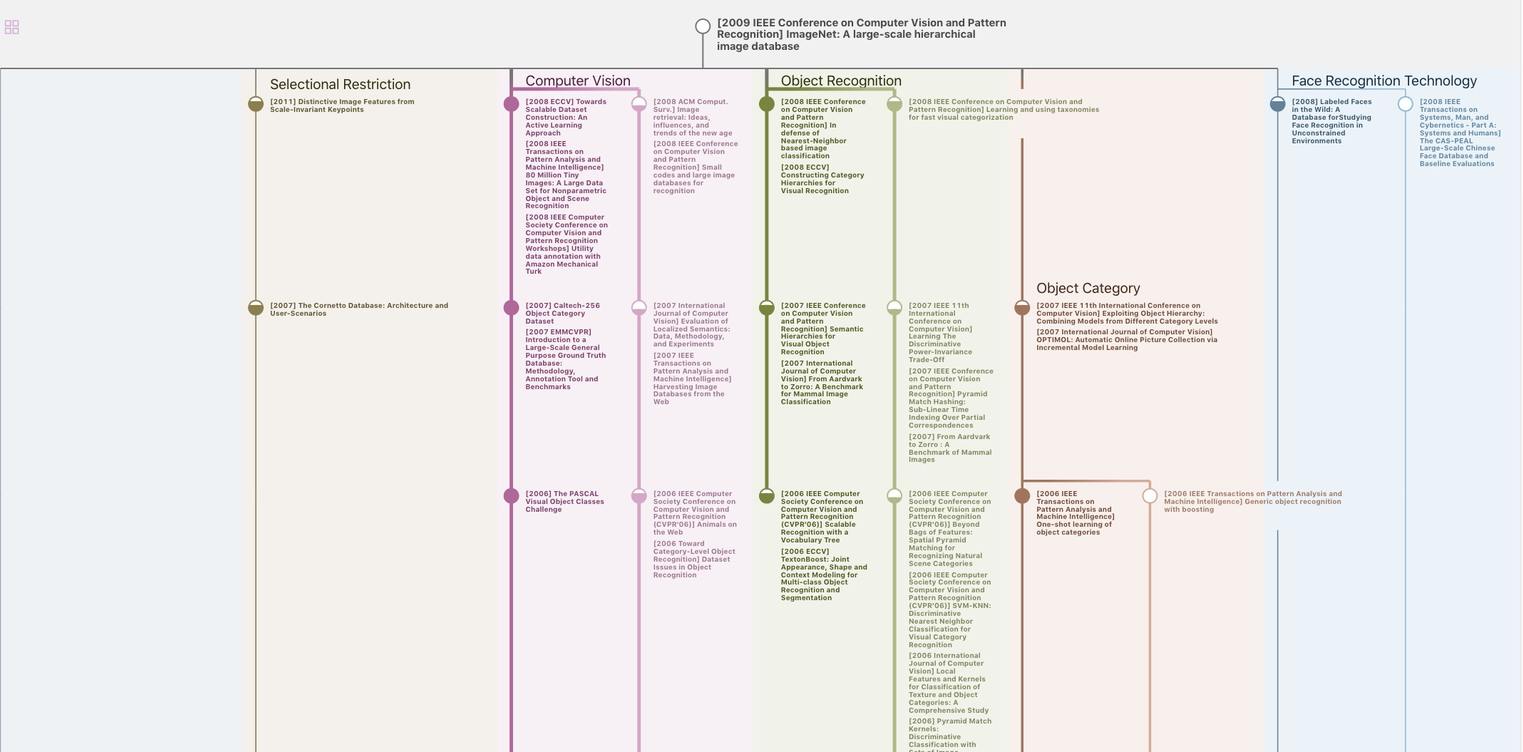
生成溯源树,研究论文发展脉络
Chat Paper
正在生成论文摘要