ART4FL: An Agent-based Architectural Approach for Trustworthy Federated Learning in the IoT
2023 EIGHTH INTERNATIONAL CONFERENCE ON FOG AND MOBILE EDGE COMPUTING, FMEC(2023)
摘要
The integration of the Internet of Things (IoT) and Machine Learning (ML) technologies has opened up for the development of novel types of systems and services. Federated Learning (FL) has enabled the systems to collaboratively train their ML models while preserving the privacy of the data collected by their IoT devices and objects. Several FL frameworks have been developed, however, they do not enable FL in open, distributed, and heterogeneous IoT environments. Specifically, they do not support systems that collect similar data to dynamically discover each other, communicate, and negotiate about the training terms (e.g., accuracy, communication latency, and cost). Towards bridging this gap, we propose ART4FL, an end-to-end framework that enables FL in open IoT settings. The framework enables systems' users to configure agents that participate in FL on their behalf. Those agents negotiate and make commitments (i.e., contractual agreements) to dynamically form federations. To perform FL, the framework deploys the needed services dynamically, monitors the training rounds, and calculates agents' trust scores based on the established commitments. ART4FL exploits a blockchain network to maintain the trust scores, and it provides those scores to negotiating agents' during the federations' formation phase.
更多查看译文
关键词
Trustworthy Federated Learning,Internet of Things,Machine Learning,Agents
AI 理解论文
溯源树
样例
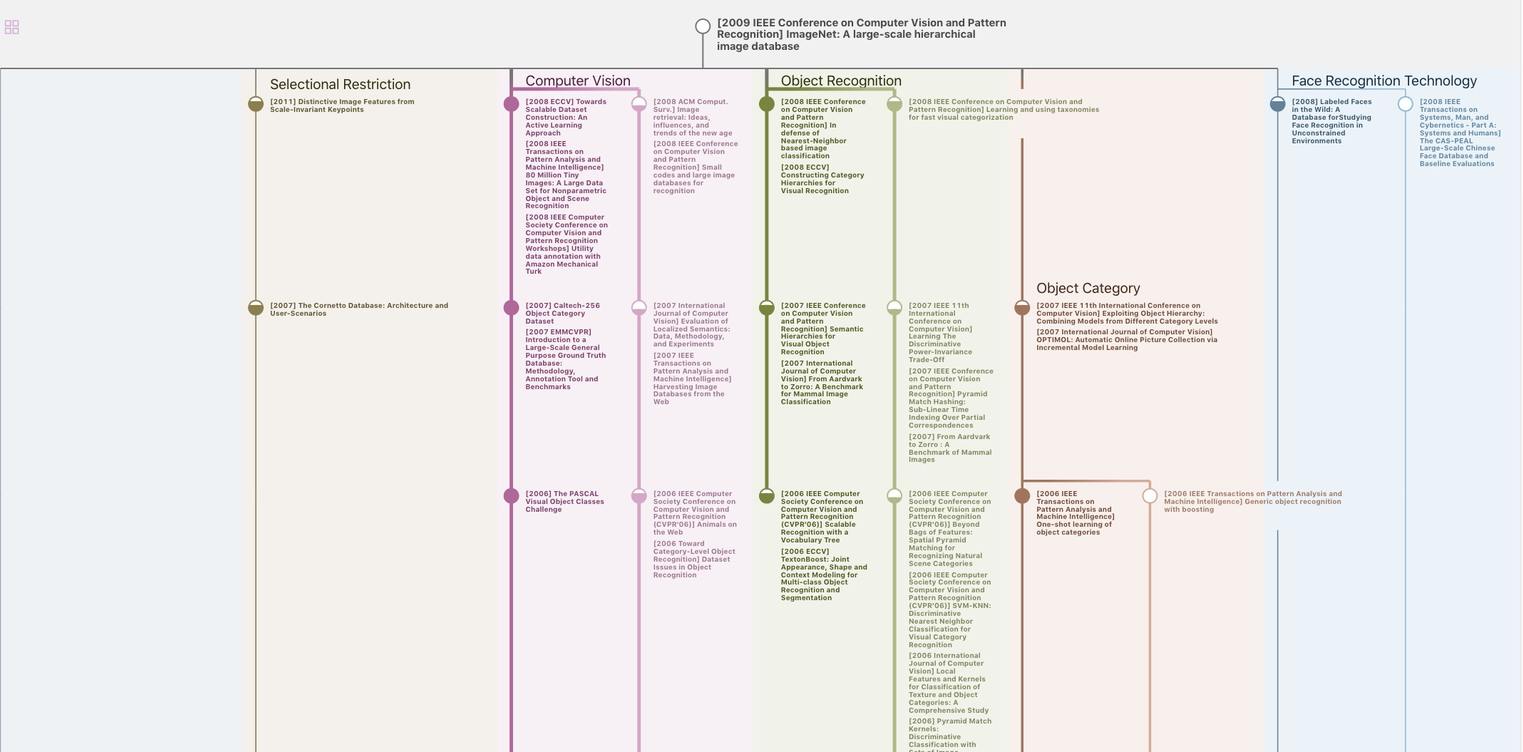
生成溯源树,研究论文发展脉络
Chat Paper
正在生成论文摘要