Trace-based Performance Analysis for Deep Learning in Edge Container Environments
2023 EIGHTH INTERNATIONAL CONFERENCE ON FOG AND MOBILE EDGE COMPUTING, FMEC(2023)
摘要
In recent years, container-based deep learning has emerged as a trending technology in edge environments. Containers offer several advantages over traditional virtual machines, including improved resource utilization and mobility. However, containers still hinder efficient use of system resources in deep learning workloads, so it is important to adopt effective resource management techniques to avoid resource conflicts. In this paper, we extract system call and event traces while executing deep learning workloads in a container and compare the results with those of running the same workloads on a host machine. By comparing system calls invoked between the two environments, we quantify the overhead of containers with respect to resource consumption and interference. We then explore the impact of running multiple containers concurrently and highlight the issues that arise in a multi-tenant environment. Our findings show that container-based deep learning can be a viable solution for deep learning workloads, but it is important to carefully consider the resource requirements and performance impact of containerization. We recommend that cloud or edge providers use a combination of resource management techniques, such as resource quotas and limits, to avoid resource wastes of containers and interference with each other.
更多查看译文
关键词
container,deep learning,virtual machine,system call,system resource
AI 理解论文
溯源树
样例
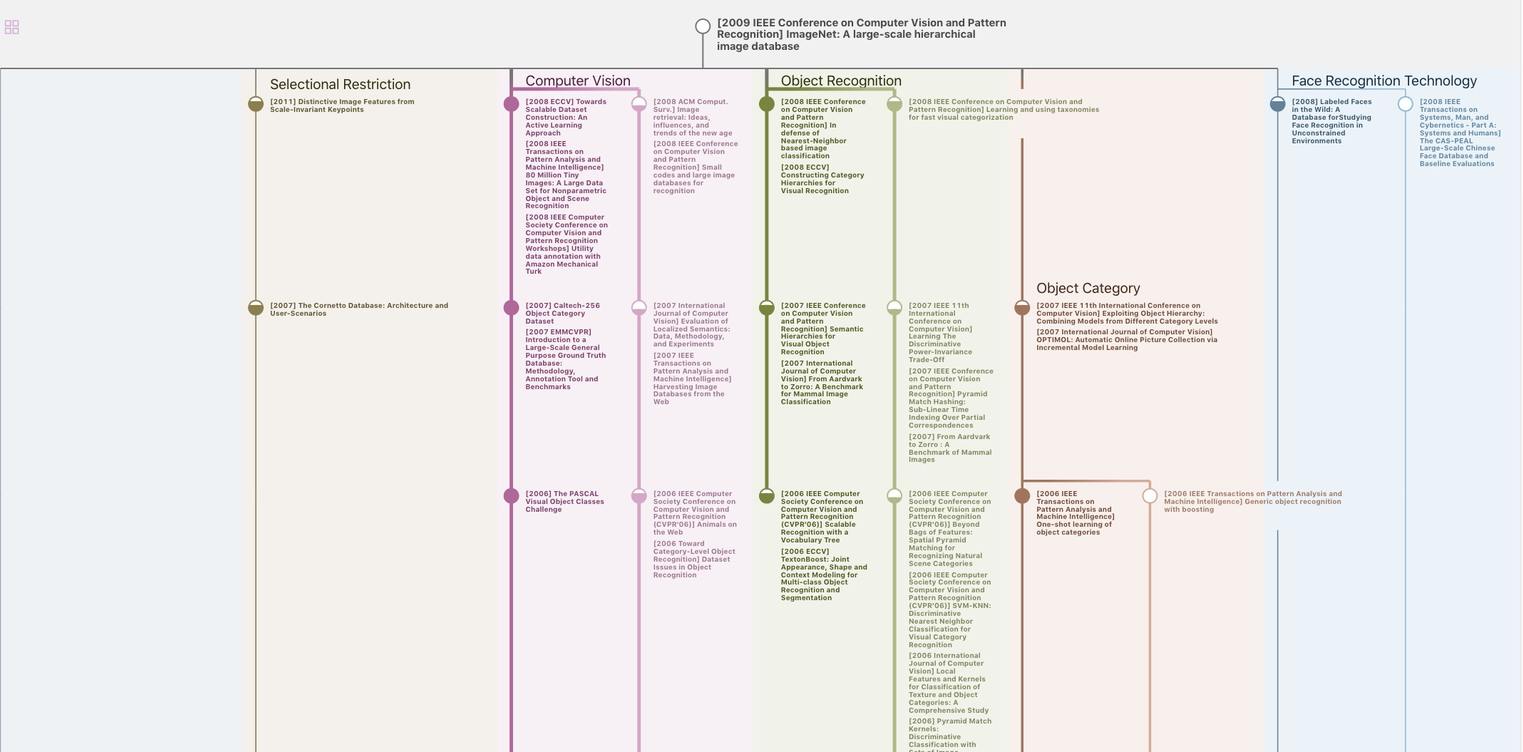
生成溯源树,研究论文发展脉络
Chat Paper
正在生成论文摘要