Evaluating Emerging AI/ML Accelerators: IPU, RDU, and NVIDIA/AMD GPUs
Companion of the 15th ACM/SPEC International Conference on Performance Engineering(2023)
摘要
The relentless advancement of artificial intelligence (AI) and machine\nlearning (ML) applications necessitates the development of specialized hardware\naccelerators capable of handling the increasing complexity and computational\ndemands. Traditional computing architectures, based on the von Neumann model,\nare being outstripped by the requirements of contemporary AI/ML algorithms,\nleading to a surge in the creation of accelerators like the Graphcore\nIntelligence Processing Unit (IPU), Sambanova Reconfigurable Dataflow Unit\n(RDU), and enhanced GPU platforms. These hardware accelerators are\ncharacterized by their innovative data-flow architectures and other design\noptimizations that promise to deliver superior performance and energy\nefficiency for AI/ML tasks.\n This research provides a preliminary evaluation and comparison of these\ncommercial AI/ML accelerators, delving into their hardware and software design\nfeatures to discern their strengths and unique capabilities. By conducting a\nseries of benchmark evaluations on common DNN operators and other AI/ML\nworkloads, we aim to illuminate the advantages of data-flow architectures over\nconventional processor designs and offer insights into the performance\ntrade-offs of each platform. The findings from our study will serve as a\nvaluable reference for the design and performance expectations of research\nprototypes, thereby facilitating the development of next-generation hardware\naccelerators tailored for the ever-evolving landscape of AI/ML applications.\nThrough this analysis, we aspire to contribute to the broader understanding of\ncurrent accelerator technologies and to provide guidance for future innovations\nin the field.
更多查看译文
AI 理解论文
溯源树
样例
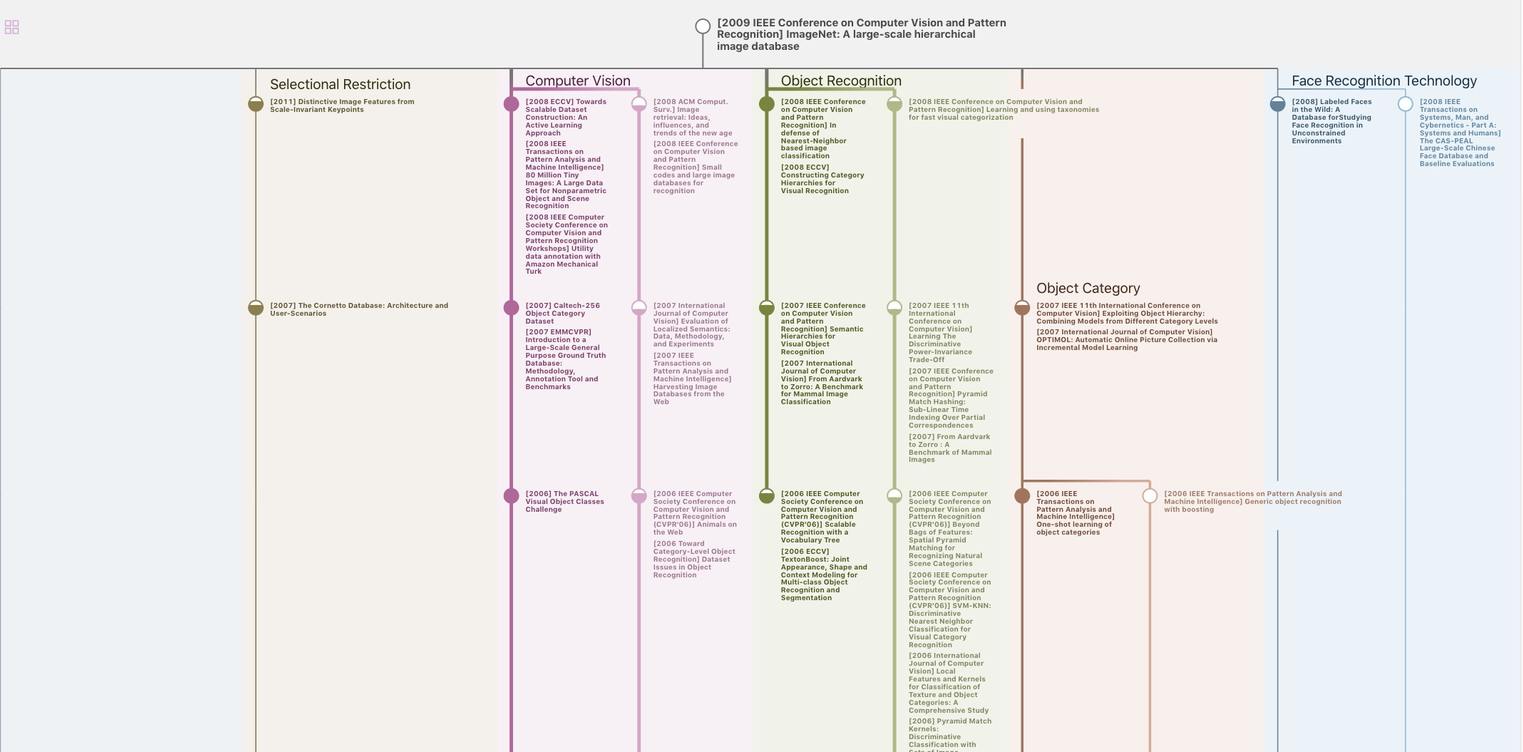
生成溯源树,研究论文发展脉络
Chat Paper
正在生成论文摘要