1-step Speech Processing and Understanding Using CTC Loss
arxiv(2023)
摘要
Recent studies have made some progress in refining end-to-end (E2E) speech recognition encoders by applying Connectionist Temporal Classification (CTC) loss to enhance named entity recognition within transcriptions. However, these methods have been constrained by their exclusive use of the ASCII character set, allowing only a limited array of semantic labels. Our proposed solution extends the E2E automatic speech recognition (ASR) system's vocabulary by adding a set of unused placeholder symbols, conceptually akin to the tokens used in sequence modeling. These placeholders are then assigned to represent semantic tags and are integrated into the transcription process as distinct tokens. We demonstrate notable improvements in entity tagging, intent discernment, and transcription accuracy on the SLUE benchmark and yields results that are on par with those for the SLURP dataset. Additionally, we provide a visual analysis of the system's proficiency in accurately pinpointing meaningful tokens over time, illustrating the enhancement in transcription quality through the utilization of supplementary semantic tags.
更多查看译文
AI 理解论文
溯源树
样例
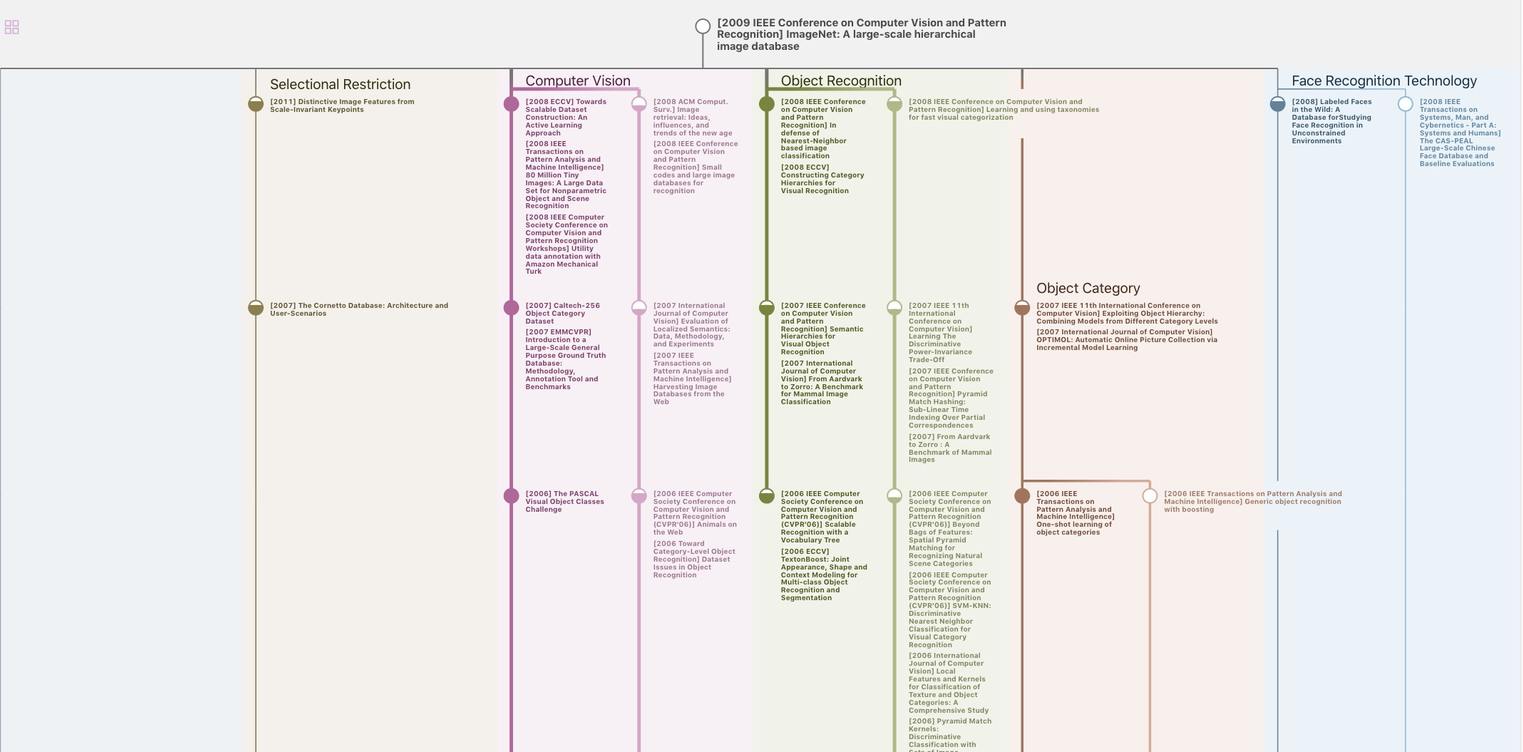
生成溯源树,研究论文发展脉络
Chat Paper
正在生成论文摘要