Oversample minority classes in Lung Ultrasound using Generative Adversarial Network
2023 IEEE International Ultrasonics Symposium (IUS)(2023)
摘要
The development of a strong methodological strategy focusing on class imbalance issues for Lung Ultrasound (LUS) data is lacking in the state of the art. Traditional oversampling methods such as geometric and affine transformations generate quasi-duplicates of the original data. This problem could be resolved through generative adversarial networks (GANs). However, in classic GANs the generator cannot handle mode collapse issues and the model’s performance remains unstable when images in different classes look similar. To address this problem, we proposed a novel approach based on a supervised autoencoder generative adversarial network (SA-GAN), which could help to oversample the minority classes. At first, we build a latent space with the help of a supervised autoencoder (SA) to handle the mode collaspe issues. Then the trained weights of the SA are used to construct the generator and discriminator models. During the adversarial training of both models, the loss function is calculated for real and generated images through a customized gradient penalty loss function. This also allows classifying each image into real or generated, and its corresponding classes. This approach was tested on a dataset comprising 5500 LUS images, which were collected from 35 COVID-19 patients. As a result, SA-GAN provided the 900 generated LUS samples in minority classes to balance with the majority class. The proposed approach significantly improved the classification accuracy by up to 5% using synthetic augmentation through SA-GAN over the classic data augmentation method.
更多查看译文
关键词
Lung Ultrasound,Generative Adversarial Network,Data Augmentation
AI 理解论文
溯源树
样例
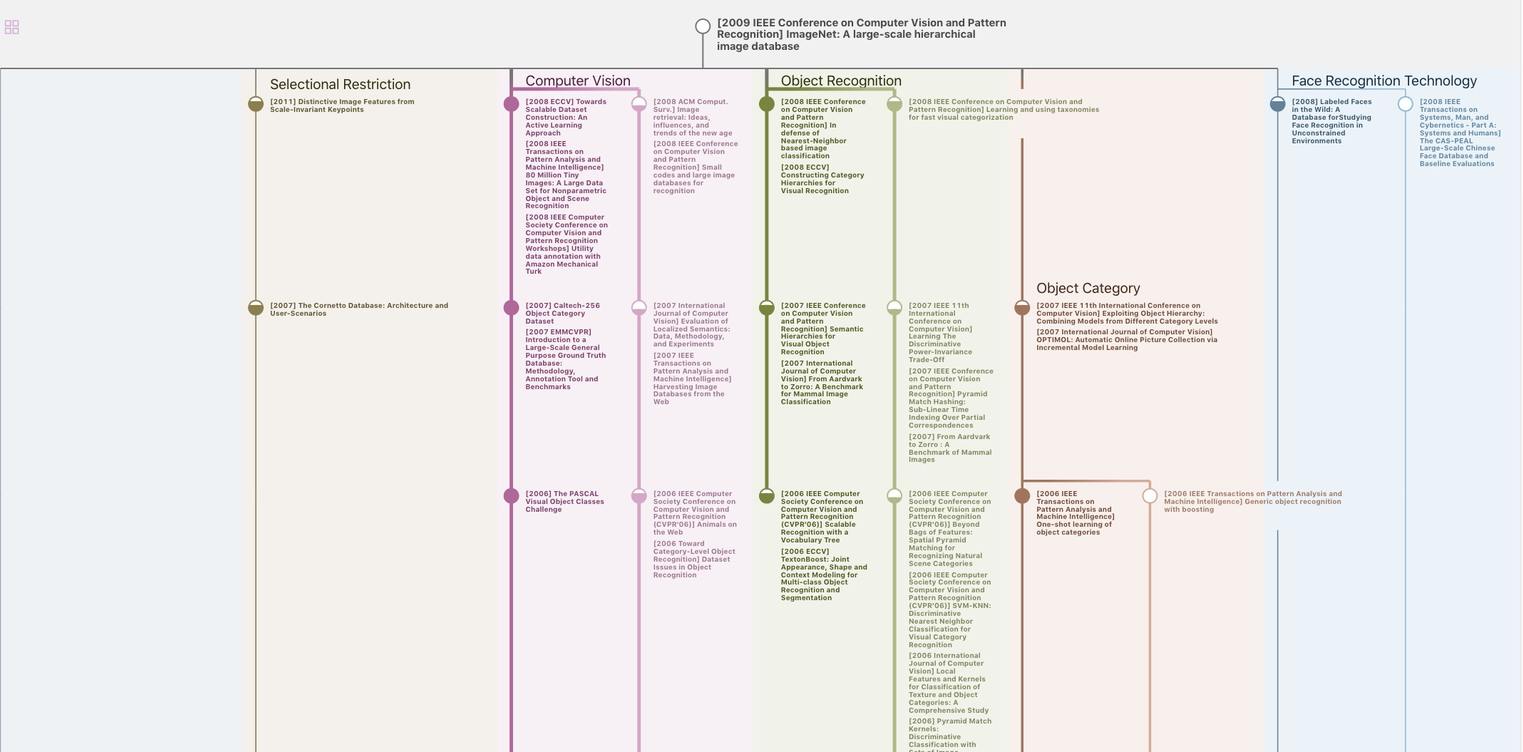
生成溯源树,研究论文发展脉络
Chat Paper
正在生成论文摘要