Better value estimation in Q-learning-based multi-agent reinforcement learning
Soft Computing(2024)
摘要
In many real-life scenarios, multiple agents necessitate cooperation to accomplish tasks. Benefiting from the significant success of deep learning, many single-agent deep reinforcement learning algorithms have been extended to multi-agent scenarios. Overestimation in value estimation of Q-learning is a significant issue that has been studied comprehensively in the single-agent domains, but rarely in multi-agent reinforcement learning. In this paper, we first demonstrate that Q-learning-based multi-agent reinforcement learning (MARL) methods generally have notably serious overestimation issues, which cannot be alleviated by current methods. To tackle this problem, we introduce the double critic networks structure and the delayed policy update to Q-learning-based multi-agent MARL methods, which reduce the overestimation and enhance the quality of policy updating. To demonstrate the versatility of our proposed method, we select several Q-learning based MARL methods and evaluate them on several multi-agent tasks on the multi-agent particle environment and SMAC. Experimental results demonstrate that the proposed method can avoid the overestimation problem and significantly improve performance. Besides, application in the Traffic Signal Control verifies the feasibility of applying the proposed method in real-world scenarios.
更多查看译文
关键词
Multi-agent reinforcement learning,Value estimation,Overestimation issue,Q-Learning
AI 理解论文
溯源树
样例
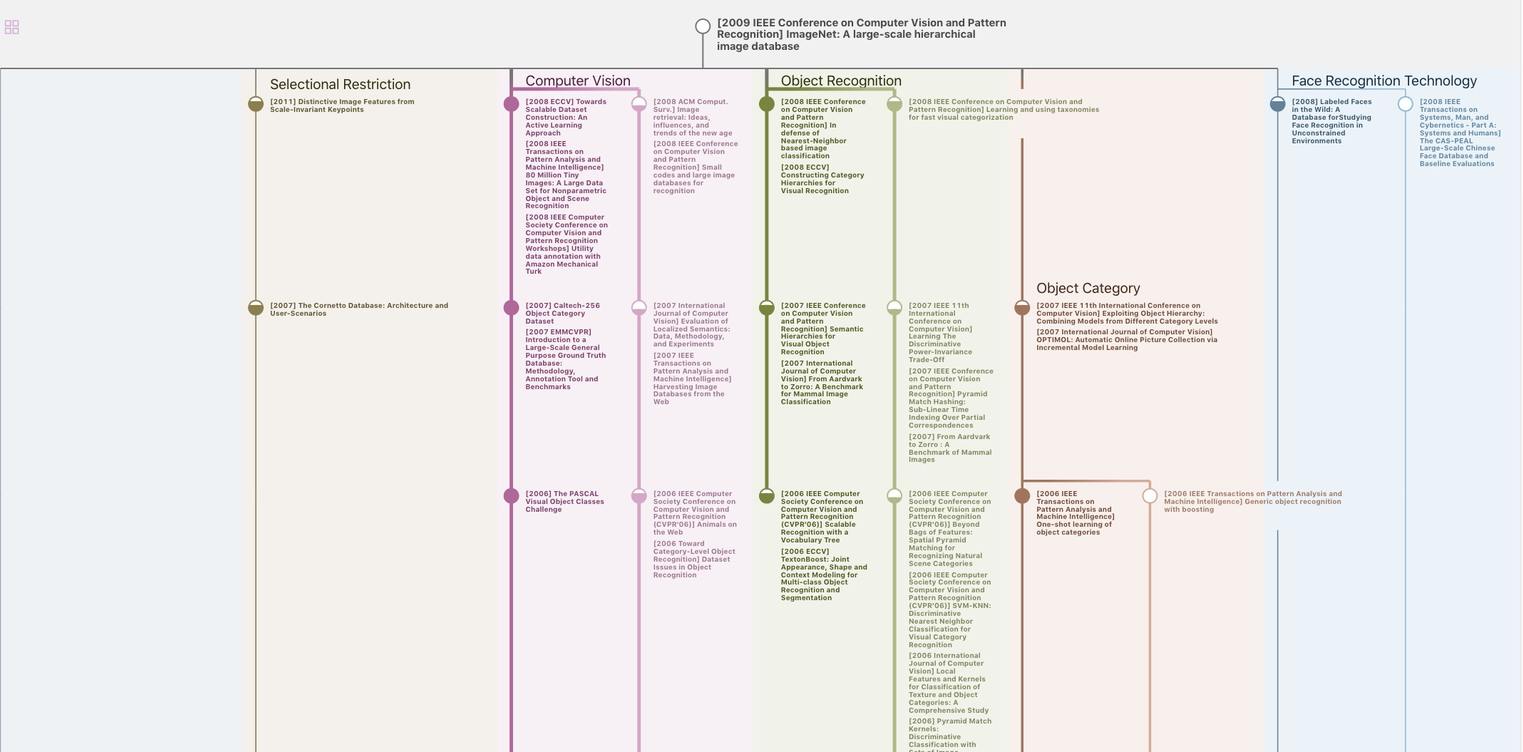
生成溯源树,研究论文发展脉络
Chat Paper
正在生成论文摘要