DeepMPSF: A Deep Learning Network for Predicting General Protein Phosphorylation Sites Based on Multiple Protein Sequence Features
JOURNAL OF CHEMICAL INFORMATION AND MODELING(2023)
摘要
Phosphorylation, as one of the most important post-translational modifications, plays a key role in various cellular physiological processes and disease occurrences. In recent years, computer technology has been gradually applied to the prediction of protein phosphorylation sites. However, most existing methods rely on simple protein sequence features that provide limited contextual information. To overcome this limitation, we propose DeepMPSF, a phosphorylation site prediction model based on multiple protein sequence features. There are two types of features: sequence semantic features, which comprise protein residue type information and relative position information within protein sequence, and protein background biophysical features, which include global semantic information containing more comprehensive protein background information obtained from pretrained models. To extract these features, DeepMPSF employs two separate subnetworks: the S71SFE module and the BBFE module, which automatically extract high-level semantic features. Our model incorporates a learning strategy for handling imbalanced datasets through ensemble learning during training and prediction. DeepMPSF is trained and evaluated on a well-established dataset of human proteins. Comparing the analysis with other benchmark methods reveals that DeepMPSF outperforms in predicting both S/T residues and Y residues. In particular, DeepMPSF showed excellent generalization performance in cross-species blind test performance, with an average improvement of 5.63%/5.72%, 22.28%/25.94%, 20.11%/17.49%, and 26.40%/28.33% for Mus musculus/Rattus norvegicus test sets in area under curves (AUCs) of ROC curve, AUC of the PR curve, F1-score, and MCC metrics, respectively. Furthermore, it also shows excellent performance in the latest updated case of natural proteins with functional phosphorylation sites. Through an ablation study and visual analysis, we uncover that the design of different feature modules significantly contributes to the accurate classification of DeepMPSF, which provides valuable insights for predicting phosphorylation sites and offers effective support for future downstream research.
更多查看译文
关键词
general protein phosphorylation sites,multiple protein sequence features,deepmpsf learning
AI 理解论文
溯源树
样例
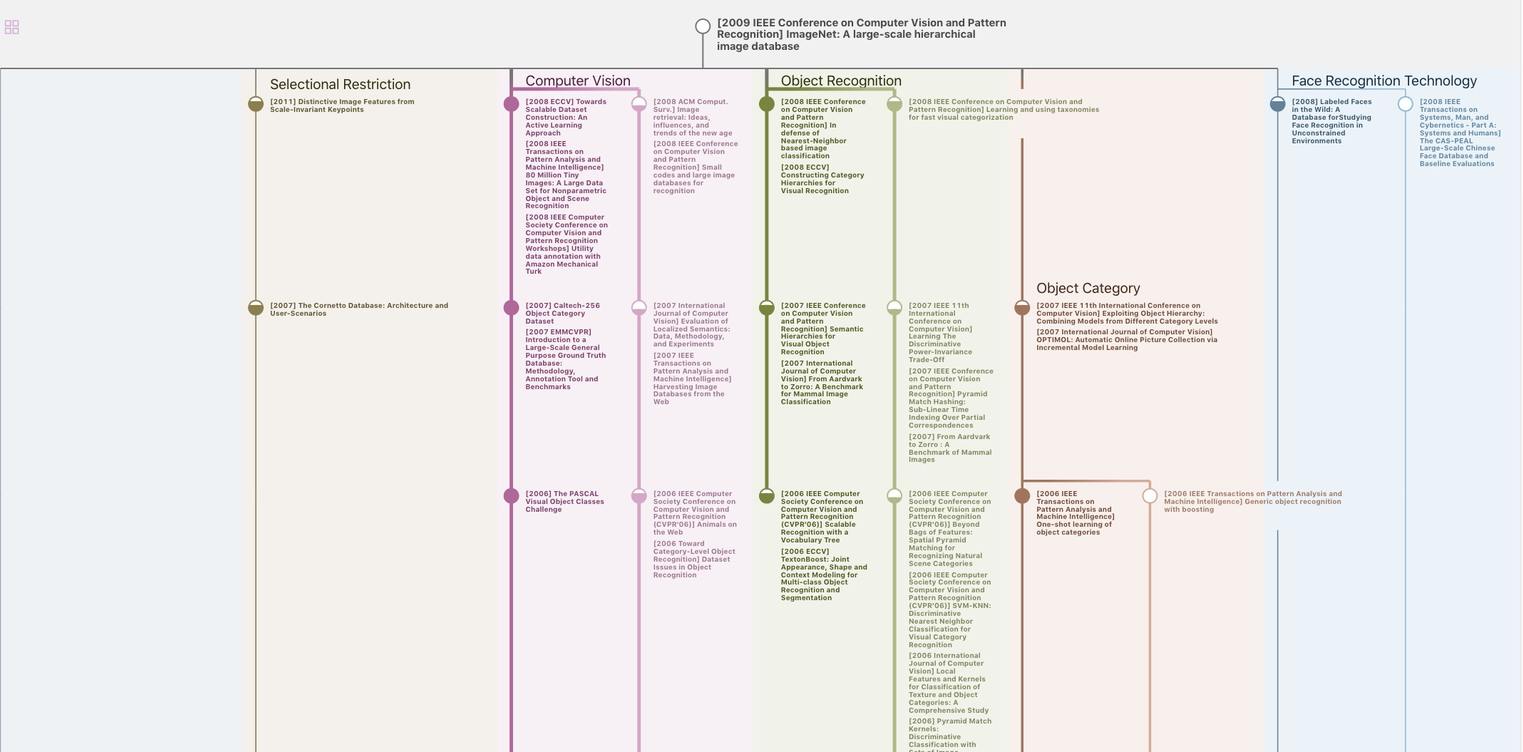
生成溯源树,研究论文发展脉络
Chat Paper
正在生成论文摘要