Structure Mapping Generative Adversarial Network for Multi-View Information Mapping Pattern Mining
IEEE TRANSACTIONS ON PATTERN ANALYSIS AND MACHINE INTELLIGENCE(2024)
摘要
Multi-view learning is dedicated to integrating information from different views and improving the generalization performance of models. However, in most current works, learning under different views has significant independency, overlooking common information mapping patterns that exist between these views. This paper proposes a Structure Mapping Generative adversarial network (SM-GAN) framework, which utilizes the consistency and complementarity of multi-view data from the innovative perspective of information mapping. Specifically, based on network-structured multi-view data, a structural information mapping model is proposed to capture hierarchical interaction patterns among views. Subsequently, three different types of graph convolutional operations are designed in SM-GAN based on the model. Compared with regular GAN, we add a structural information mapping module between the encoder and decoder wthin the generator, completing the structural information mapping from the micro-view to the macro-view. This paper conducted sufficient validation experiments using public imaging genetics data in Alzheimer's Disease Neuroimaging Initiative (ADNI) dataset. It is shown that SM-GAN outperforms baseline and advanced methods in multi-label classification and evolution prediction tasks.
更多查看译文
关键词
Multi-view learning,structural information mapping,graph convolution,generative adversarial network
AI 理解论文
溯源树
样例
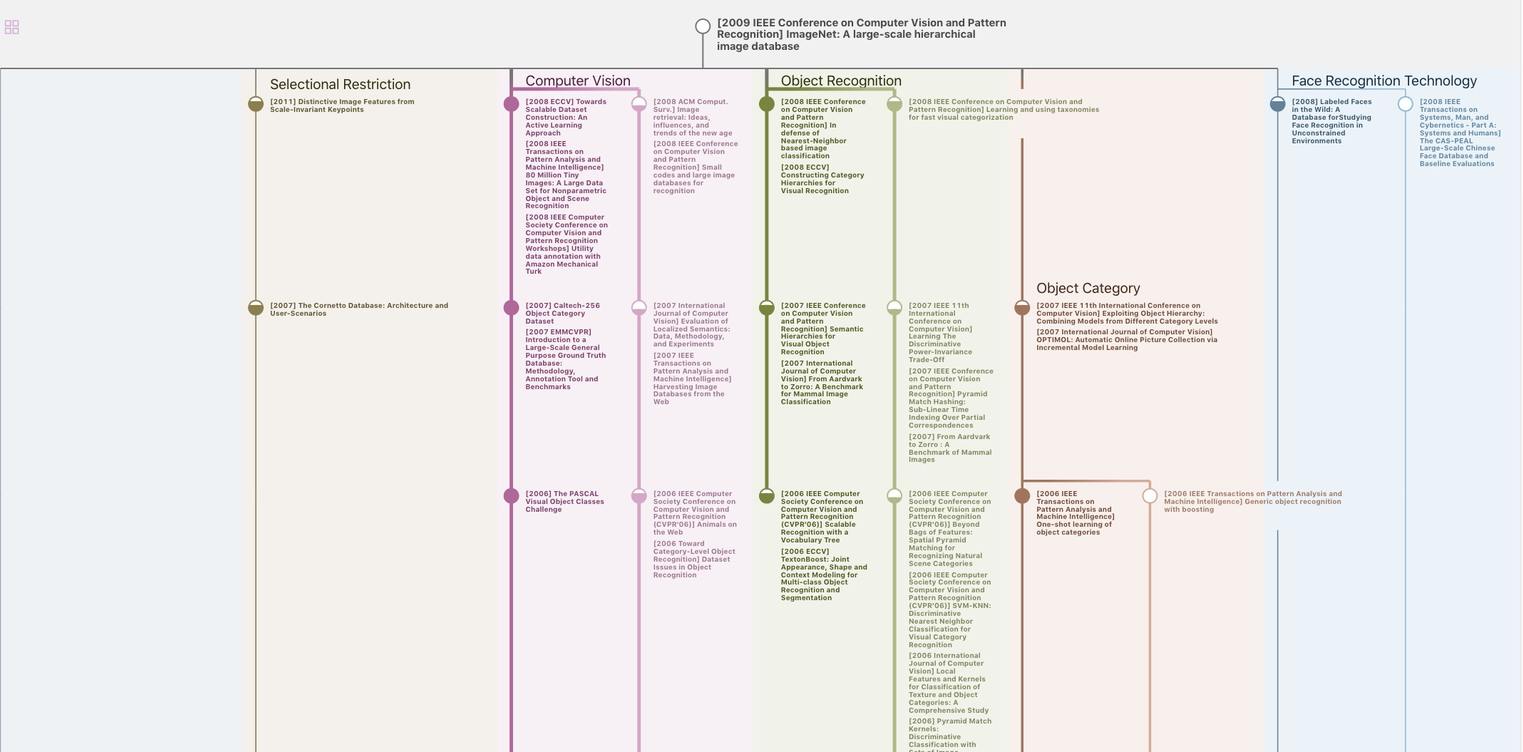
生成溯源树,研究论文发展脉络
Chat Paper
正在生成论文摘要