Multi-resolution Time-Series Transformer for Long-term Forecasting.
CoRR(2023)
摘要
The performance of transformers for time-series forecasting has improved significantly. Recent architectures learn complex temporal patterns by segmenting a time-series into patches and using the patches as tokens. The patch size controls the ability of transformers to learn the temporal patterns at different frequencies: shorter patches are effective for learning localized, high-frequency patterns, whereas mining long-term seasonalities and trends requires longer patches. Inspired by this observation, we propose a novel framework, Multi-resolution Time-Series Transformer (MTST), which consists of a multi-branch architecture for simultaneous modeling of diverse temporal patterns at different resolutions. In contrast to many existing time-series transformers, we employ relative positional encoding, which is better suited for extracting periodic components at different scales. Extensive experiments on several real-world datasets demonstrate the effectiveness of MTST in comparison to state-of-the-art forecasting techniques.
更多查看译文
关键词
transformer,forecasting,time-series time-series,multi-resolution,long-term
AI 理解论文
溯源树
样例
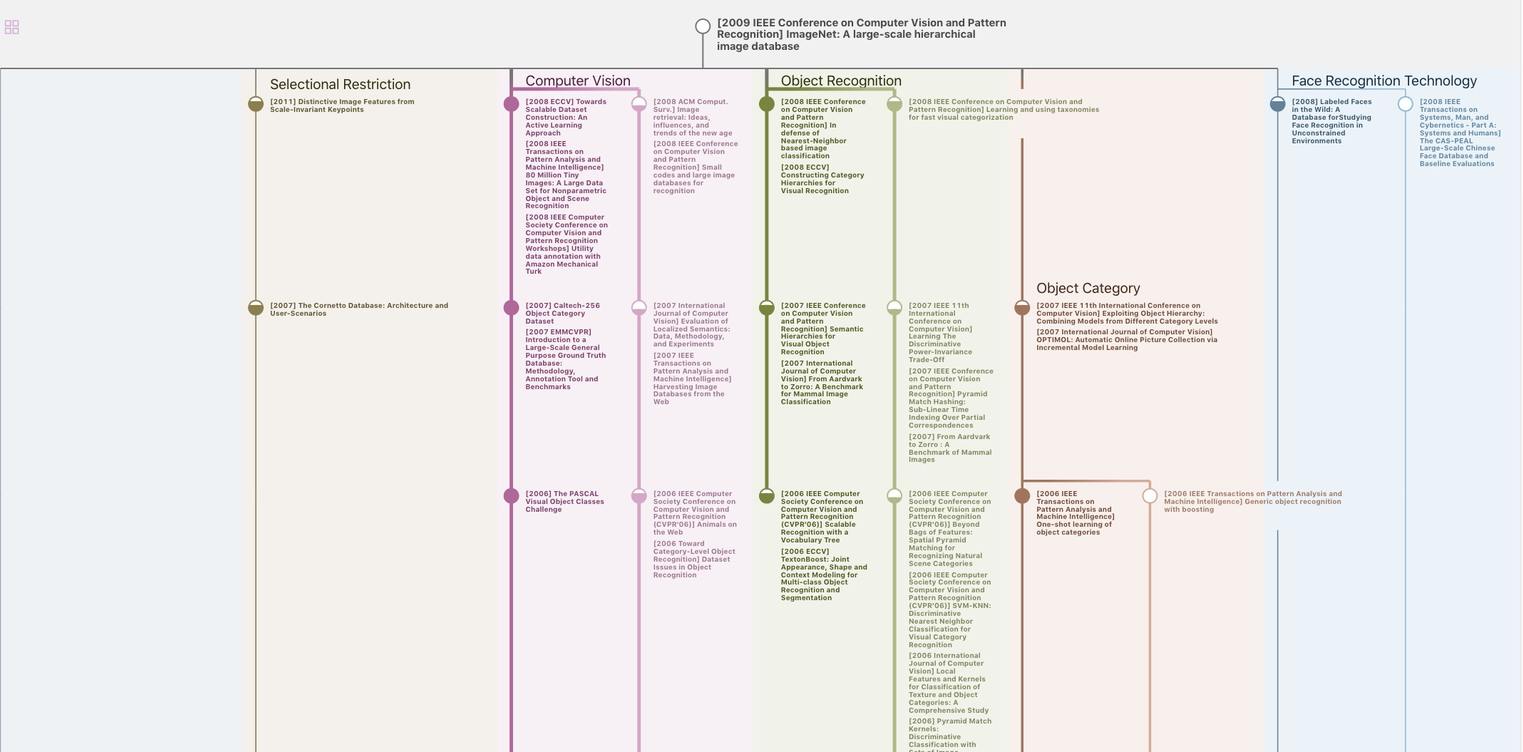
生成溯源树,研究论文发展脉络
Chat Paper
正在生成论文摘要