Neuron synergy based explainable neural network for manufacturing cycle time forecasting
JOURNAL OF MANUFACTURING SYSTEMS(2023)
摘要
Manufacturing cycle time prediction plays a critical role in manufacturing systems to ensure on-time product delivery. This article proposes an explainable deep learning method called PACER for parsing key circuits in the cycle time prediction network to deal with dynamic environments. PACER is designed with 1) the key neuron identification algorithm is proposed to filter out key neurons with the comprehensive consideration of global output contribution and local connection strength; and 2) the key neural circuit search algorithm is proposed to find the optimal neural circuits in a prediction network through hierarchical synergy. The experimental results demonstrate that PACER can effectively identify key neurons with a higher removal accuracy loss of 76.7% for searching the key circuit.
更多查看译文
关键词
Neural network,Interpretable learning,Neuron synergy,Cycle time forecasting,Manufacturing system optimization
AI 理解论文
溯源树
样例
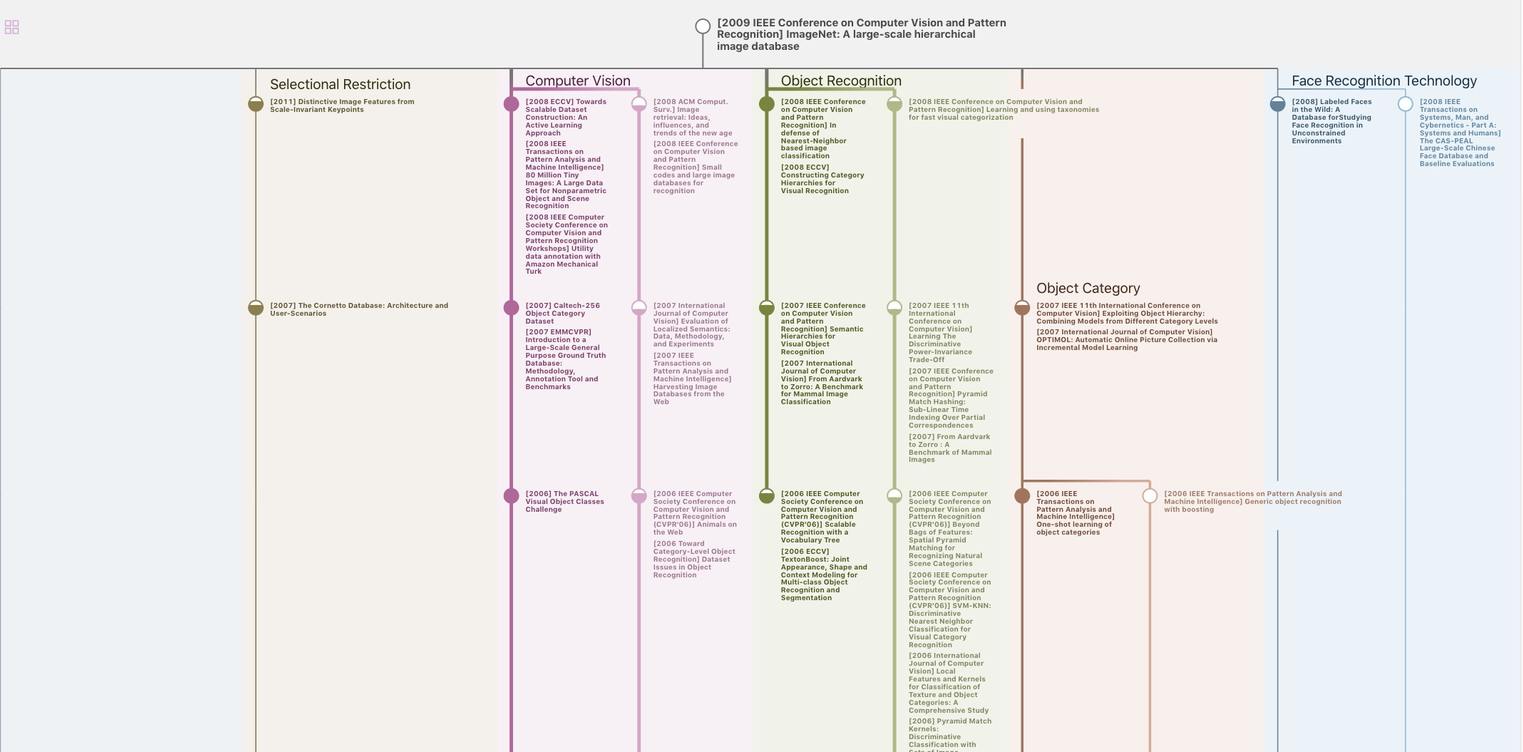
生成溯源树,研究论文发展脉络
Chat Paper
正在生成论文摘要