A Graph Neural Network with Multiple Auxiliary Tasks for Accurate Single Cell Classiଁcation.
SPML(2023)
摘要
Single-cell RNA sequencing (scRNA-seq) data provide new opportunities for many research directions in the field of biology, one of which is the identification of cell types. However, traditional cell identification methods are time-consuming, uneconomical, complex, and difficult to be applied to large data sets. Here, we propose a graph neural network with multiple auxiliary tasks (MATGNN) for cell classification. First, we construct the adjacency matrix between cells based on their gene expression data, which represents the interactions between cells. Next, a complete undirected graph between cells is obtained and it is fed into the graph neural network model, which is employed to aggregate the information of node's neighborhood. Then, we improve the robustness of the proposed model through three different auxiliary tasks. Specifically, the first auxiliary task balances the information in the adjacency matrix between cells. The second one captures inherent information about the interactions between cells of same type and the third one is responsible for mining the structural information from the undirected graph between cells. Those three auxiliary tasks enable the model to deeply dig into the structural information and link information of the cell graph. Finally, we conduct extensive experiments on the gold datasets and the results indicate that our models outperform the state-of-the-art methods for single cell classification. Compared with other state-of-the-art methods, our method achieves the highest accuracy on most datasets. In particular, our method improves the classification accuracy of datasets BaronMouse, Segerstolpe and BaronHuman by 0.5%, 1.2%$ and 0.7%, respectively. We prove the effectiveness of establishing adjacency matrix between cells in cell classification. Three auxiliary tasks show that we can mine more cellular properties from existing datasets.
更多查看译文
AI 理解论文
溯源树
样例
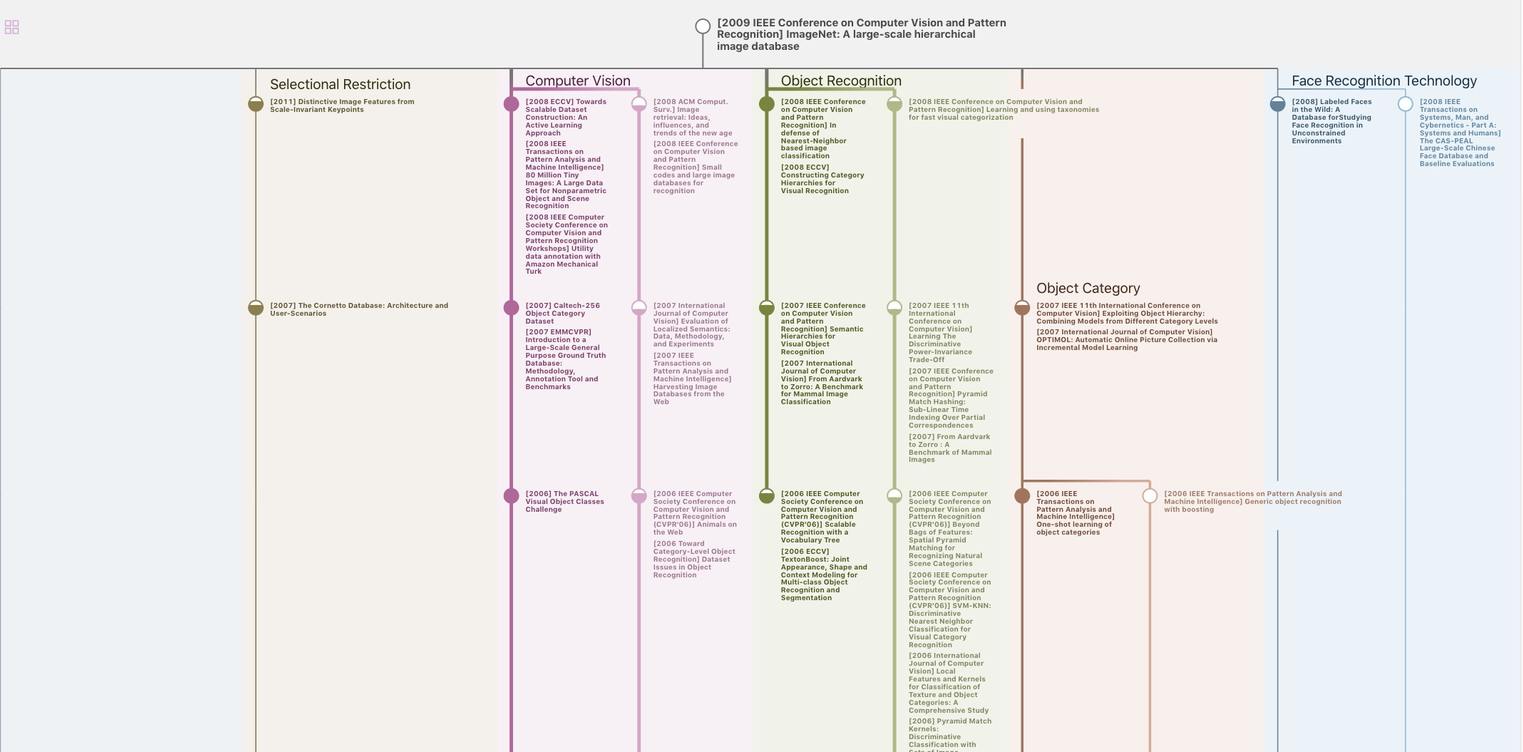
生成溯源树,研究论文发展脉络
Chat Paper
正在生成论文摘要