Unfooling SHAP and SAGE: Knockoff Imputation for Shapley Values.
xAI (1)(2023)
摘要
Abstract Shapley values have achieved great popularity in explainable artificial intelligence. However, with standard sampling methods, resulting feature attributions are susceptible to adversarial attacks. This originates from target function evaluations at extrapolated data points, which are easily detectable and hence, enable models to behave accordingly. In this paper, we introduce a novel strategy for increased robustness against adversarial attacks of both local and global explanations: Knockoff imputed Shapley values. Our approach builds on the model-X knockoff methodology, which generates synthetic data that preserves statistical properties of the original samples. This enables researchers to flexibly choose an appropriate model to generate on-manifold data for the calculation of Shapley values upfront, instead of having to estimate a large number of conditional densities or make strong parametric assumptions. Through real and simulated data experiments, we demonstrate the effectiveness of knockoff imputation against adversarial attacks.
更多查看译文
关键词
shapley values,knockoff imputation,shap,sage
AI 理解论文
溯源树
样例
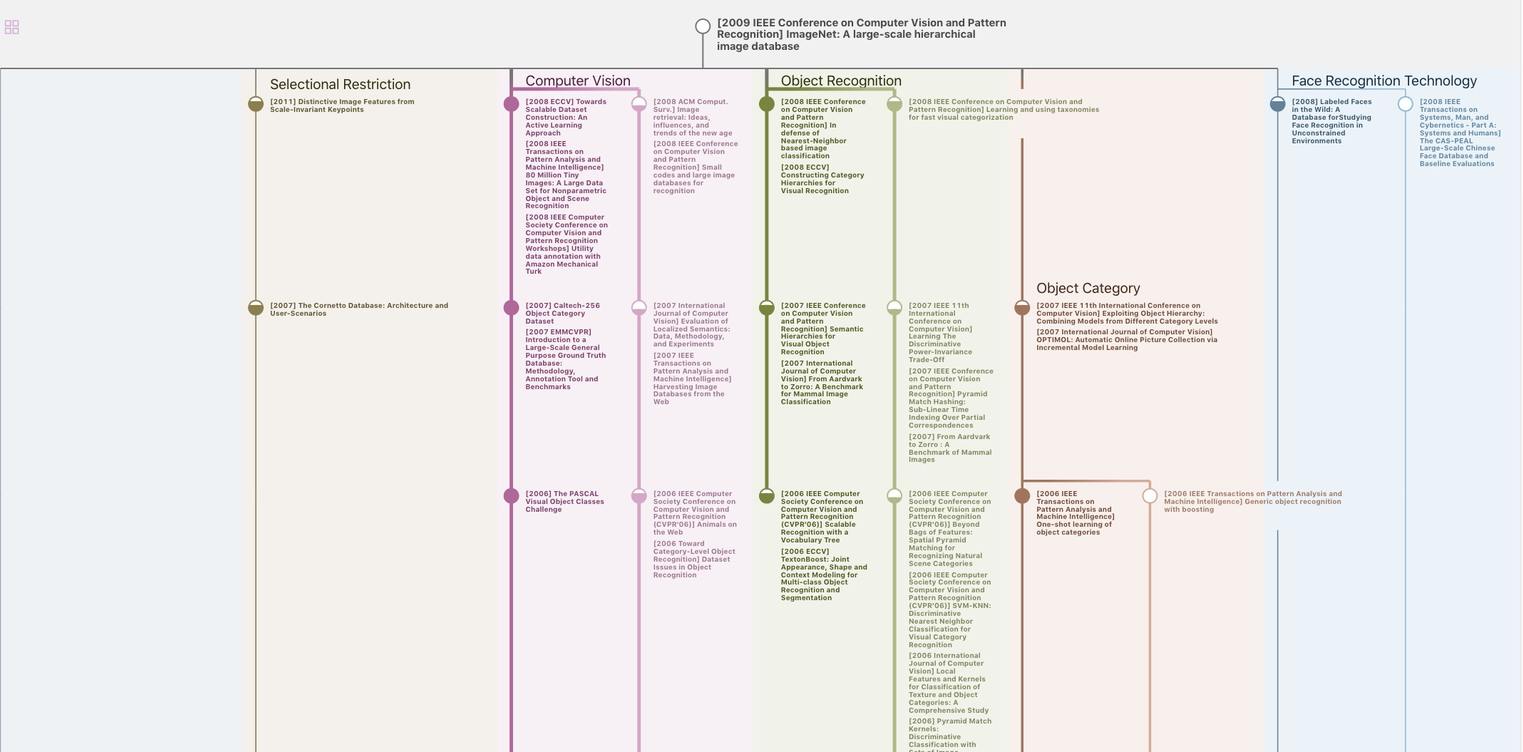
生成溯源树,研究论文发展脉络
Chat Paper
正在生成论文摘要