A 22nm 56TOPS/W 6/8-bit Linearly-scalable R-2R Multiply-and-Accumulate Architecture with 2.2ns Latency.
ESSCIRC(2023)
摘要
This paper presents a current-domain compute-in-memory (CIM) architecture for acceleration of Artfficial Intelligence (AI) edge inferencing. A novel multiply-and-accumulate (MAC) scheme is introduced by exploiting the R-2R resistor ladder as a binary-weighted current recombiner. The area and power of the proposed scheme scale linearly as numerical precision increases for both input activation and weight. Computation latency is maintained single cycle. A prototype in 22nm FDSOI CMOS process achieves 2. 2ns system latency, 56TOPS/W energy efficiency and 4TOPS/m$\mathrm{m}^{2}$ area efficiency with 6-bit input activation and 8-bit weight.
更多查看译文
关键词
in-memory computation,analog approximate computing,neural network,current-domain
AI 理解论文
溯源树
样例
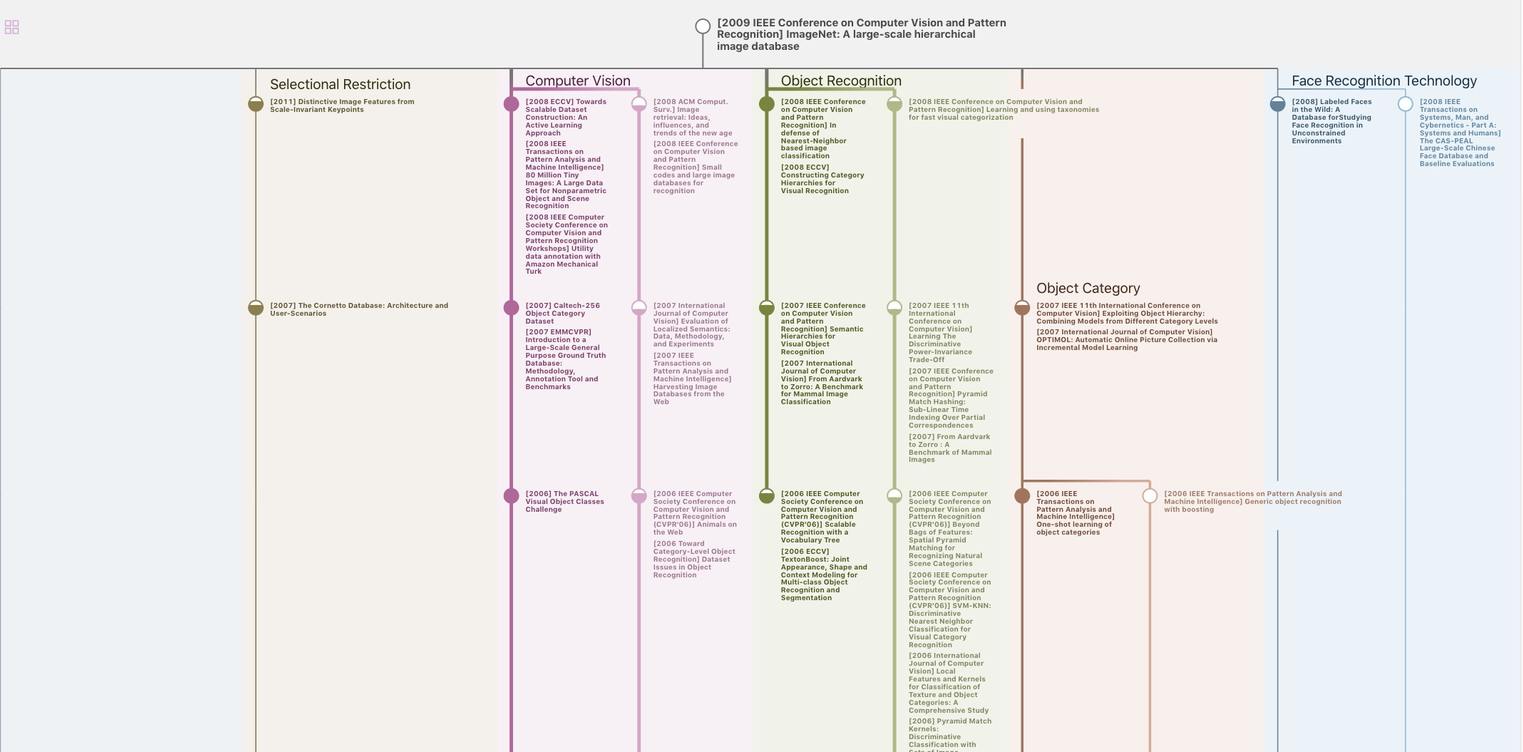
生成溯源树,研究论文发展脉络
Chat Paper
正在生成论文摘要