Enhancing Detailed Feedback to Chinese Writing Learners Using a Soft-Label Driven Approach and Tag-Aware Ranking Model.
NLPCC (1)(2023)
摘要
This paper focuses on providing detailed and specific feedback for Chinese writing learners, which is challenging due to the uncertainty of the feedback space. 30 common tags are identified based on clustering 363k comment phrases. By predicting the corresponding tags for an input, learners can gain insights on how to improve their work. However, the various tag types and non-exhaustive annotation pose challenges for model training. To address this, we propose a soft-label-driven approach to construct a more accurate relationship between samples and the tag space. We use a relevance matrix between different tags to adjust the positive label’s confidence across the entire tag space. Additionally, we propose a tag-aware regression-based ranking model that further improves performance. Our experiments demonstrate that the proposed soft-label approach performs better than a hard-label approach, and the proposed ranking model enhances performance. Our data and code are available at: https://github.com/Zhe0311/DetailedFeedback2ChineseWritingLearners .
更多查看译文
关键词
chinese writing learners,ranking,detailed feedback,soft-label,tag-aware
AI 理解论文
溯源树
样例
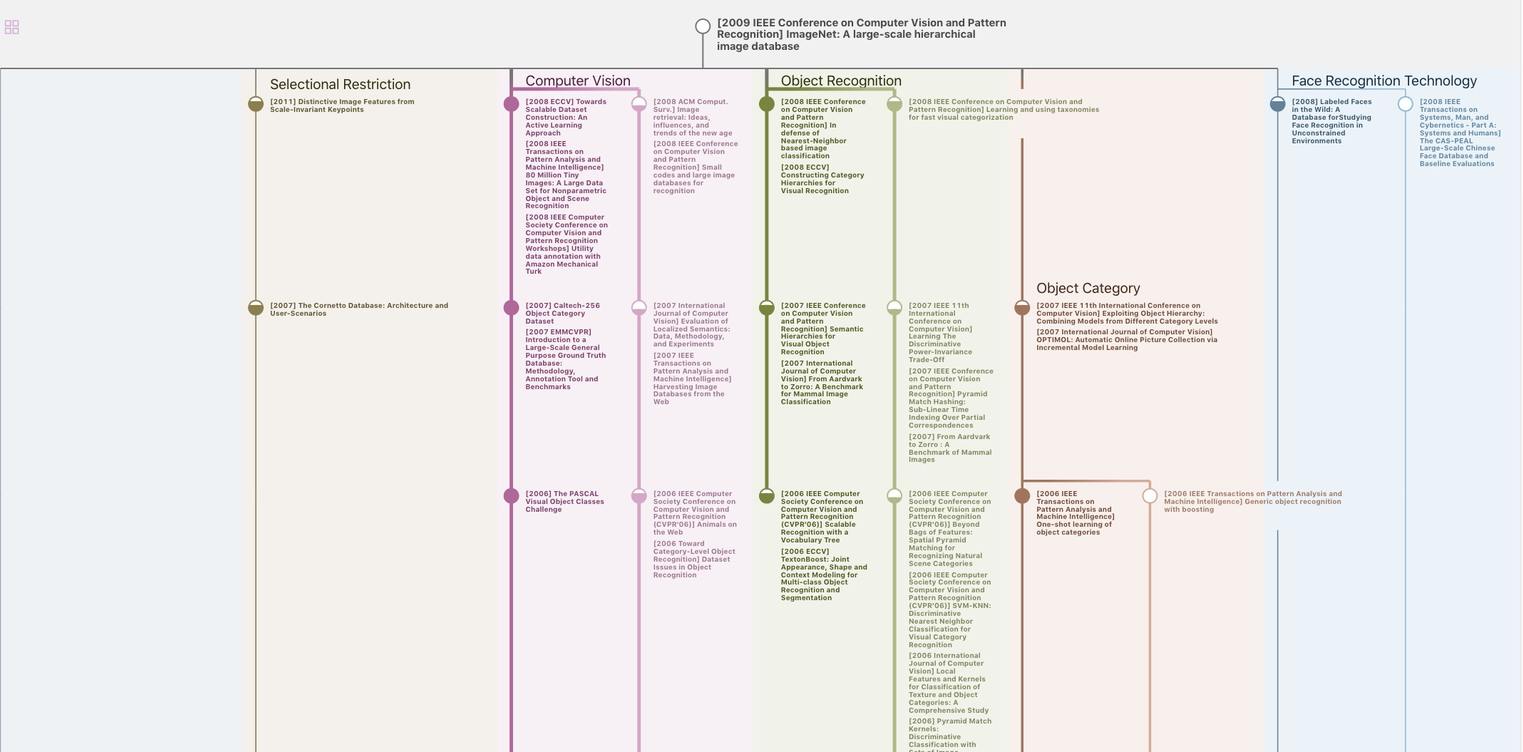
生成溯源树,研究论文发展脉络
Chat Paper
正在生成论文摘要