SW-YOLO: Improved YOLOv5s Algorithm for Blood Cell Detection.
ICIRA (3)(2023)
摘要
This paper proposes an improved target detection algorithm SW-YOLO based on the YOLOv5s framework to solve the problems of low detection accuracy, wrong detection and missed detection in blood cell detection tasks. To begin with, the end of the backbone network is fused with Swin Transformer to improve network feature extraction. Next, since blood cells are mostly small and medium-sized targets, resulting in poor detection of large cells, the network layer that identifies large cells is removed. In addition, the normal convolution in the PANet network is replaced with depth-separable convolution during the feature fusion process to ensure the accuracy and real-time detection while having better detection results for small targets. At last, the loss function of the prediction layer uses EIOU to solve the positive and negative sample imbalance problem of CIOU. Compared with existing target detection algorithms such as Faster-RCNN, YOLOv4 and YOLOv5s, SW-YOLO improves to 99.5%, 95.3% and 93.3% mAP on the blood cell dataset BCCD for white blood cells, red blood cells and platelets respectively. The experimental results are eximious and the algorithm is highly practical for blood cell detection.
更多查看译文
关键词
blood cell detection,yolov5s algorithm,sw-yolo
AI 理解论文
溯源树
样例
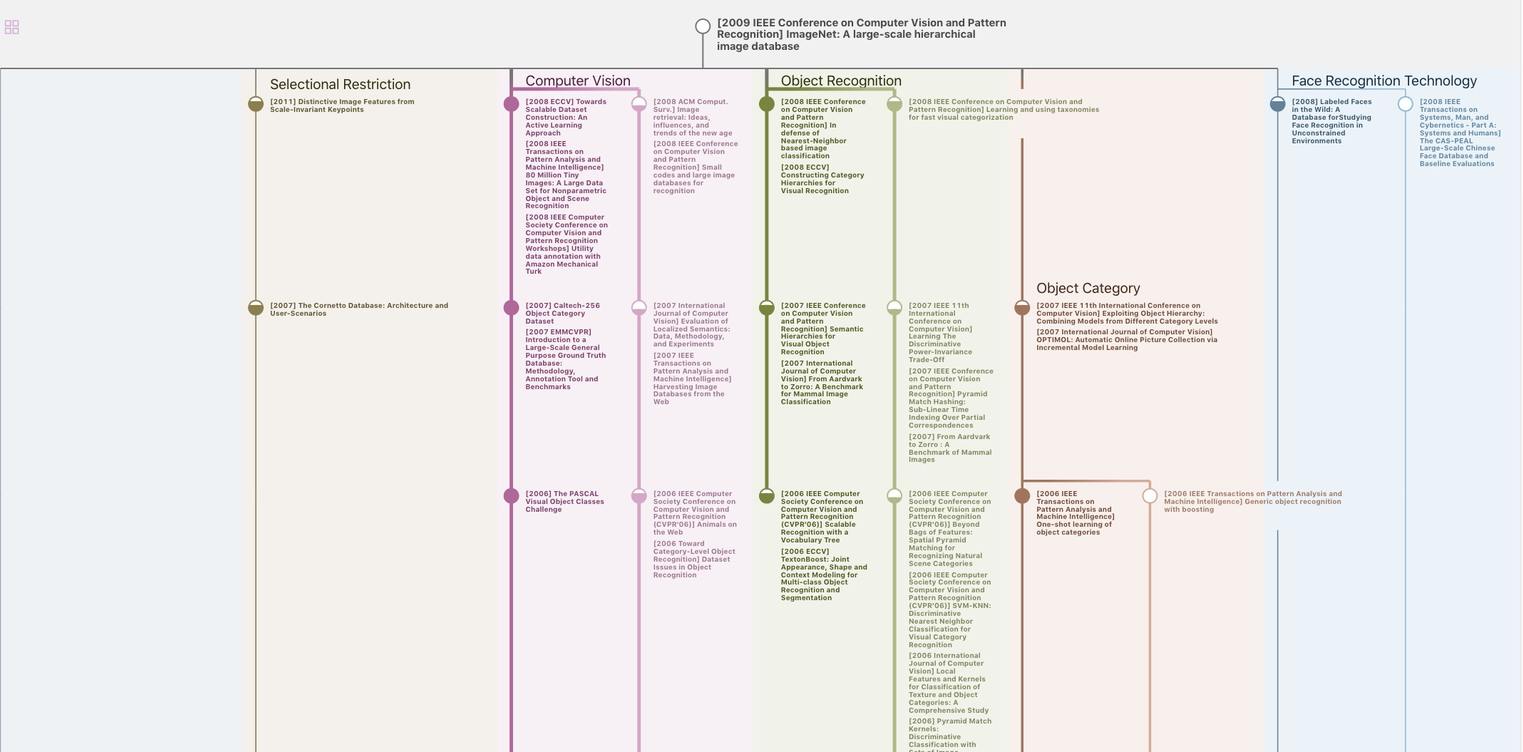
生成溯源树,研究论文发展脉络
Chat Paper
正在生成论文摘要