Growing Memory Network with Random Weight 3DCNN for Continuous Human Action Recognition
2023 IEEE INTERNATIONAL CONFERENCE ON FUZZY SYSTEMS, FUZZ(2023)
摘要
Recent research has shown that continuous learning models, which emulate the learning mechanisms of the human brain, can effectively learn new data over time. However, many of these models require significant amounts of task-specific data to extract visual and temporal features using convolutional neural networks (CNNs) and recurrent neural networks (RNNs). Additionally, pre-training these data feature extractors is often computationally expensive and time-consuming. Furthermore, traditional continuous learning models require data labeling before learning new data, which is difficult to achieve in real-world applications such as human action recognition. To address these limitations, we present a novel Random Weight Convolutional-Growing Memory Network (RWC-GMN) model that enables real-time continuous learning of new human actions in the real world. Our model uses a fixed random weight 3DCNN as a feature extractor, thereby eliminating the need for pre-training and enabling our model to start learning new actions immediately. The random weight features will be fed into the Growing Memory Network (GMN) for learning. GMN is a self-organizing incremental network that emulates human episodic memory. The network size can grow and shrink to adapt to input data continuously. Moreover, our model does not require pre-labeling of new human actions, allowing for label-free learning in real-time. Finally, our model can learn new actions continuously while retaining previously acquired knowledge, avoiding the problem of catastrophic forgetting.
更多查看译文
关键词
Random Weight 3DCNN,Contimuous Learning,Human Action Recognition
AI 理解论文
溯源树
样例
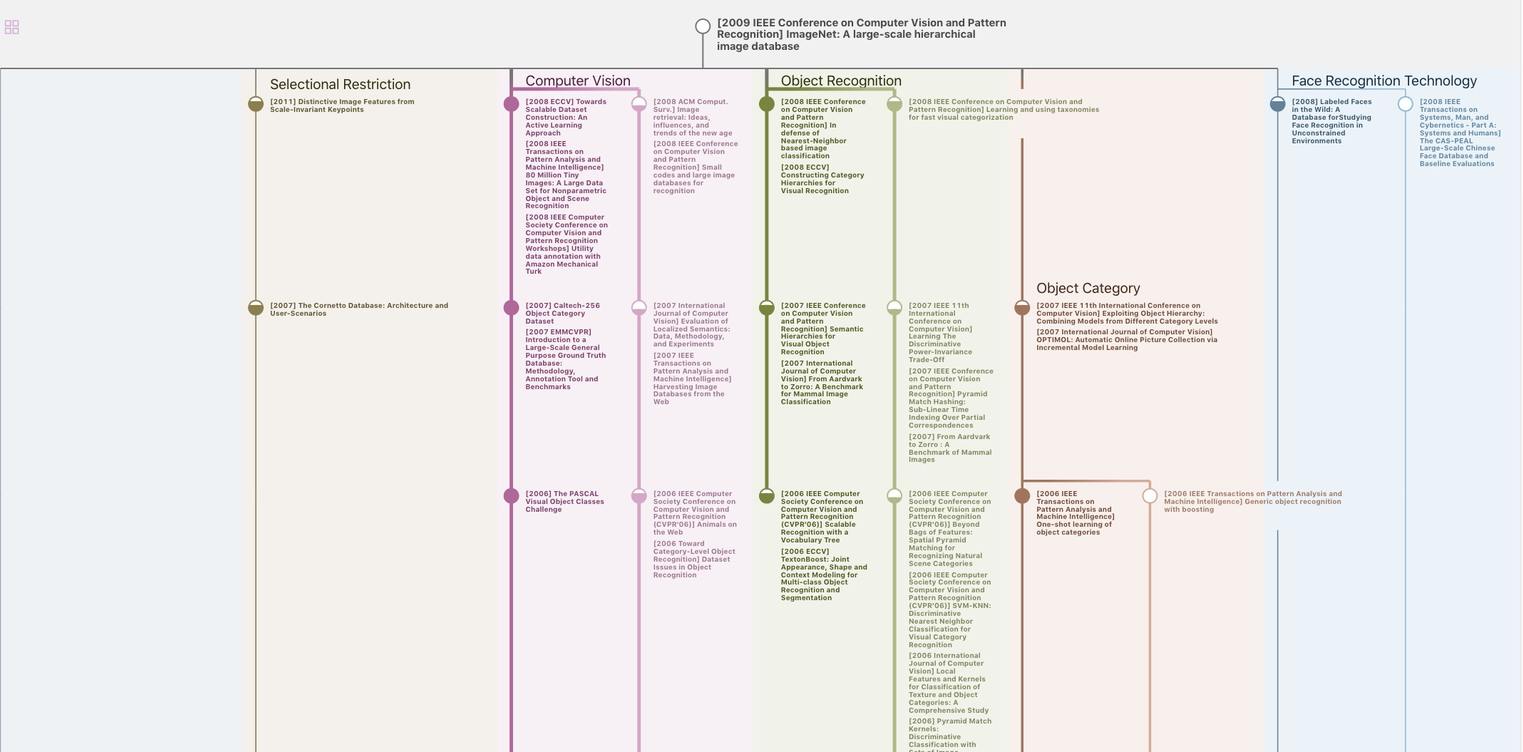
生成溯源树,研究论文发展脉络
Chat Paper
正在生成论文摘要