Bayesian Networks as Approximations of Biochemical Networks.
EPEW(2023)
摘要
Biochemical networks are usually modeled by Ordinary Differential Equations (ODEs) that describe time evolution of the concentrations of the interacting (biochemical) species for specific initial concentrations and certain values of the interaction rates. The uncertainty in the measurements of the model parameters ( i.e. interaction rates) and the concentrations ( i.e. state variables) is not an uncommon occurrence due to biological variability and noise. So, there is a great need to predict the evolution of the species for some intervals or probability distributions instead of specific initial conditions and parameter values. To this end, one can employ either phase portrait method together with bifurcation analysis as a dynamical system approach, or Dynamical Bayesian Networks (DBNs) in a probabilistic domain. The first approach is restricted to the case of a few number of parameters, while DBNs have recently been used for large biochemical networks. In this paper, we show that time-homogeneous ODE parameters can be efficiently estimated with Bayesian Networks. The accuracy and computation time of our approach is compared to two-slice time-invariant DBNs that have already been used for this purpose. The efficiency of our approach is demonstrated on two toy examples and the EGF-NGF signaling pathway.
更多查看译文
关键词
networks,biochemical,approximations
AI 理解论文
溯源树
样例
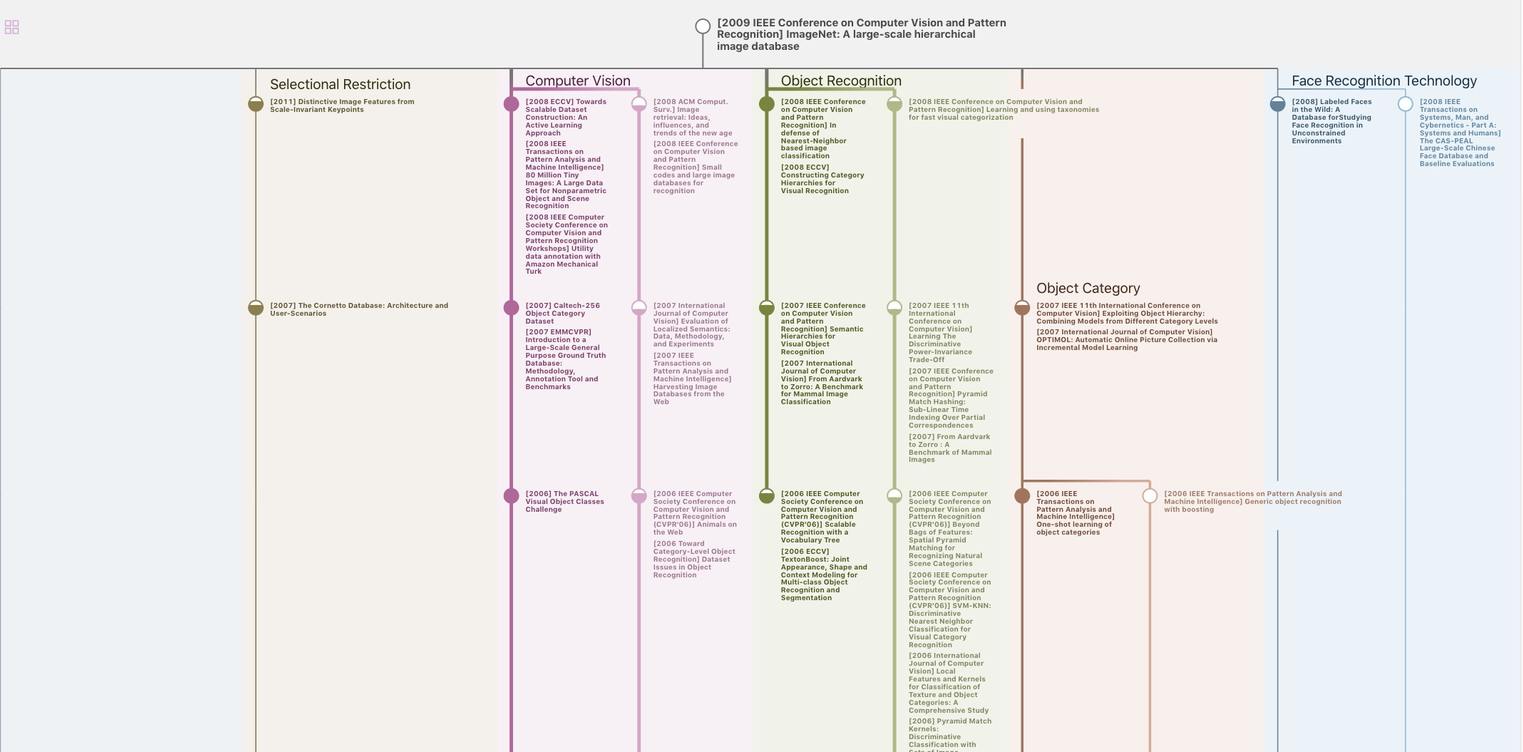
生成溯源树,研究论文发展脉络
Chat Paper
正在生成论文摘要