CIMNet: A Contextual Information Mining Network for Real-time Instance Segmentation.
CCRIS(2023)
摘要
Real-time instance segmentation seeks to partition and localize instances with desired efficiency, which has been applied to autonomous driving, robot navigation, and medical image analysis, and so forth. By learning hierarchical features, convolutional neural networks (CNNs) have dominated the instance segmentation task and achieved remarkable results. However, the inherent limitation of receptive field makes the CNNs capture deep semantic features at the costs of spatial details, leading to the lack of contextual information which are essential for learning discriminative representations of semantic instances. To address these problems, we propose a contextual information mining network, named CIMNet, to exploit extensive contexts for real-time instance segmentation. Firstly, a coarse-to-fine feature mining module (CFFM) combines channel attention with multi-scale pooling to explore multi-scale contextual information in the deepest feature. We then devise a multi-path feature enhancement module (MFEM) to expand effective receptive field, which captures spatial contextual information to enhance instances localization. Finally, aiming at improving the accuracy of small object, we design a dual-path FPN framework with a negligible extra computational cost to learn the scale invariance of each instance, achieving competitive speed while maintaining high segmentation accuracy. Extensive experiments have been conducted on the challenging COCO2017, our method can achieve 35.3 AP at 59.0 FPS, which shows that the proposed network achieves better accuracy/speed trade-off in real-time instance segmentation.
更多查看译文
AI 理解论文
溯源树
样例
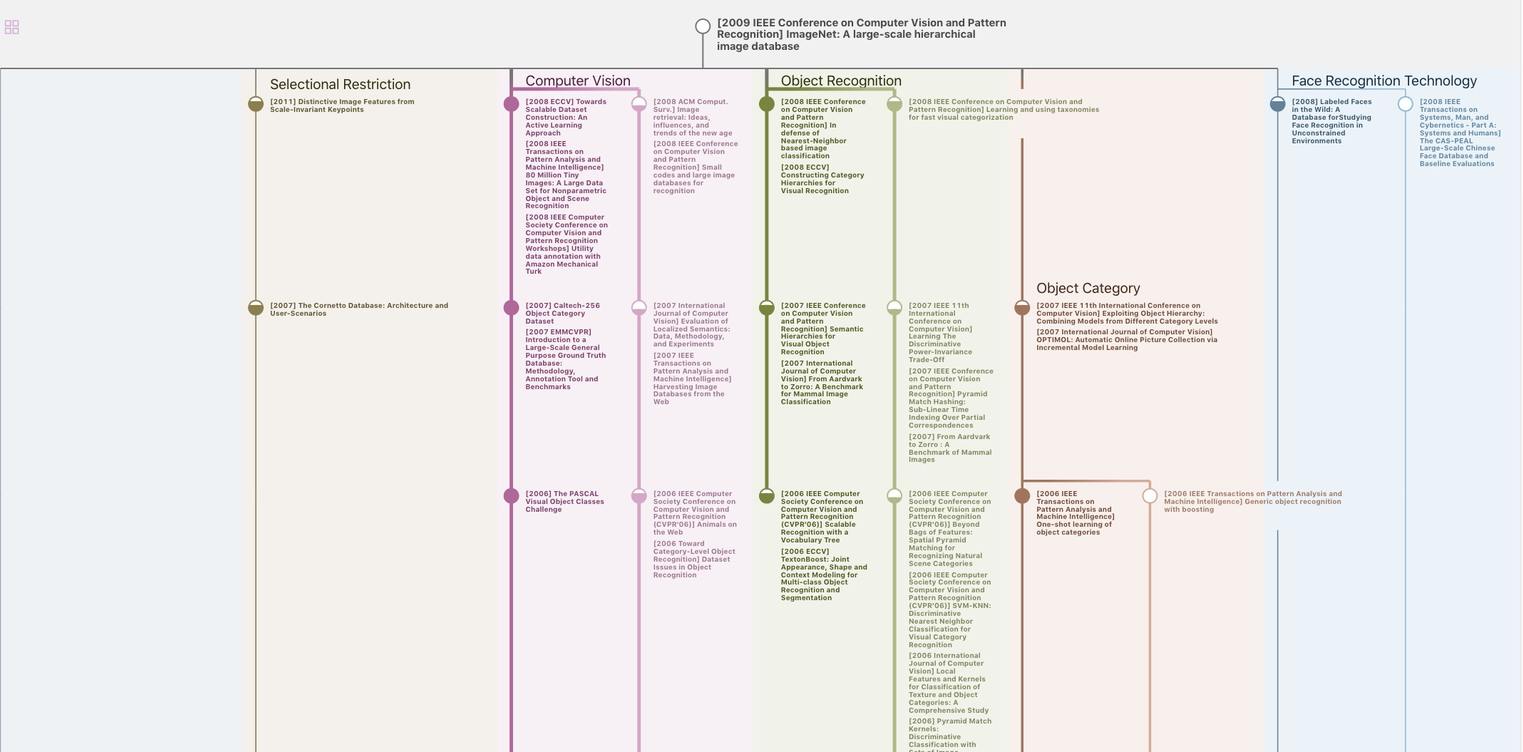
生成溯源树,研究论文发展脉络
Chat Paper
正在生成论文摘要