SAFER: Safe Collision Avoidance Using Focused and Efficient Trajectory Search with Reinforcement Learning.
CASE(2023)
摘要
Collision avoidance is key for mobile robots and agents to operate safely in the real world. In this work we present SAFER, an efficient and effective collision avoidance system that is able to improve safety by correcting the control commands sent by an operator. It combines real-world reinforcement learning (RL), search-based online trajectory planning, and automatic emergency intervention, e.g. automatic emergency braking (AEB). The goal of the RL is to learn an effective corrective control action that is used in a focused search for collision-free trajectories, and to reduce the frequency of triggering automatic emergency braking. This novel setup enables the RL policy to learn safely and directly on mobile robots in a real-world indoor environment, minimizing actual crashes even during training. Our real-world experiments show that, when compared with several baselines, our approach enjoys a higher average speed, lower crash rate, less emergency intervention, smaller computation overhead, and smoother overall control.
更多查看译文
关键词
automatic emergency braking,collision-free trajectories,control commands,effective collision avoidance system,effective corrective control action,efficient collision avoidance system,efficient trajectory search,emergency intervention,focused search,mobile robots,real-world experiments,real-world indoor environment,reinforcement learning,RL policy,safe collision avoidance,SAFER,smoother overall control,trajectory planning
AI 理解论文
溯源树
样例
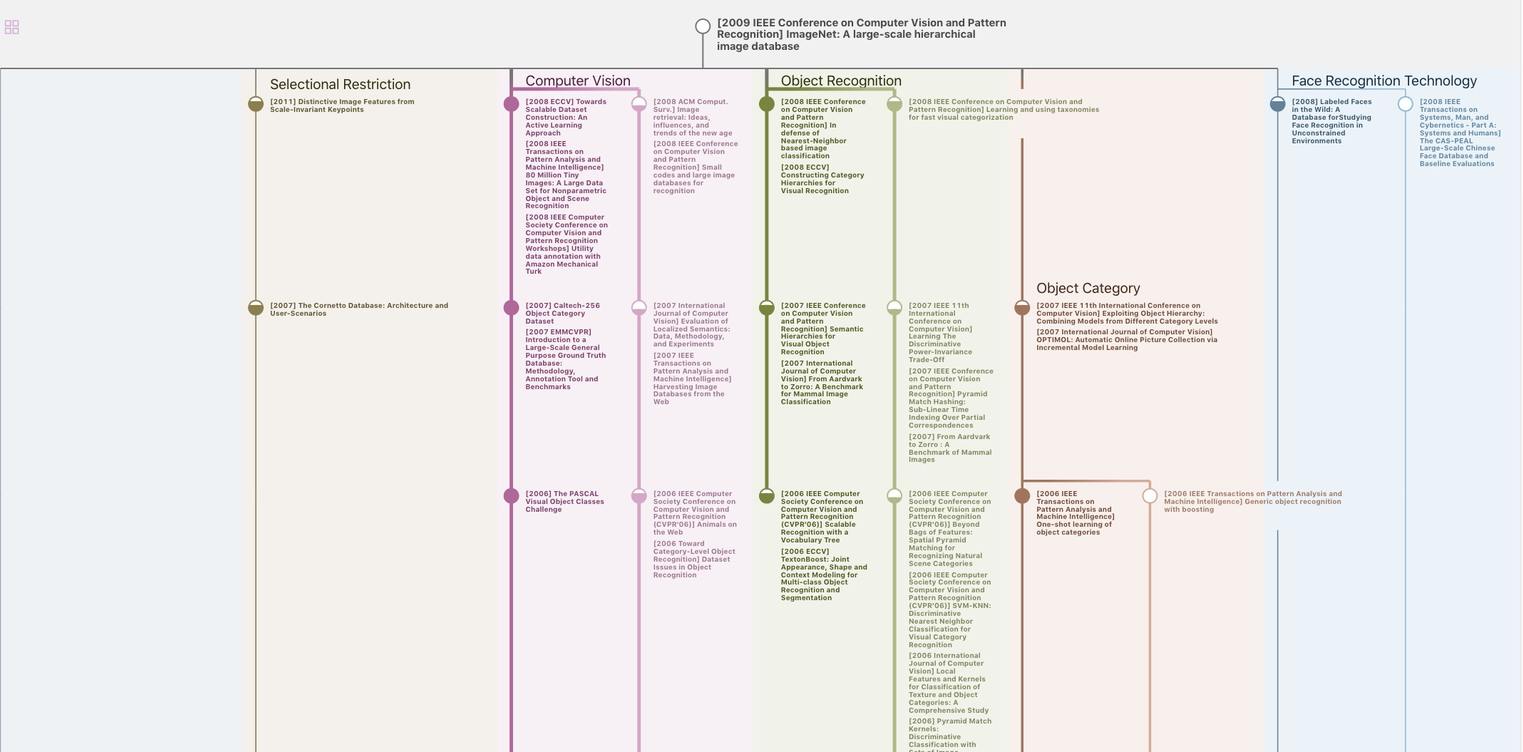
生成溯源树,研究论文发展脉络
Chat Paper
正在生成论文摘要