Deep Bayesian Quantization for Supervised Neuroimage Search
MACHINE LEARNING IN MEDICAL IMAGING, MLMI 2023, PT II(2024)
摘要
Neuroimage retrieval plays a crucial role in providing physicians with access to previous similar cases, which is essential for case-based reasoning and evidence-based medicine. Due to low computation and storage costs, hashing-based search techniques have been widely adopted for establishing image retrieval systems. However, these methods often suffer from nonnegligible quantization loss, which can degrade the overall search performance. To address this issue, this paper presents a compact coding solution namely Deep Bayesian Quantization (DBQ), which focuses on deep compact quantization that can estimate continuous neuroimage representations and achieve superior performance over existing hashing solutions. Specifically, DBQ seamlessly combines the deep representation learning and the representation compact quantization within a novel Bayesian learning framework, where a proxy embedding-based likelihood function is developed to alleviate the sampling issue for traditional similarity supervision. Additionally, a Gaussian prior is employed to reduce the quantization losses. By utilizing pre-computed lookup tables, the proposed DBQ can enable efficient and effective similarity search. Extensive experiments conducted on 2, 008 structural MRI scans from three benchmark neuroimage datasets demonstrate that our method outperforms previous state-of-the-arts.
更多查看译文
关键词
Neuroimage search,Deep Bayesian learning,Quantization
AI 理解论文
溯源树
样例
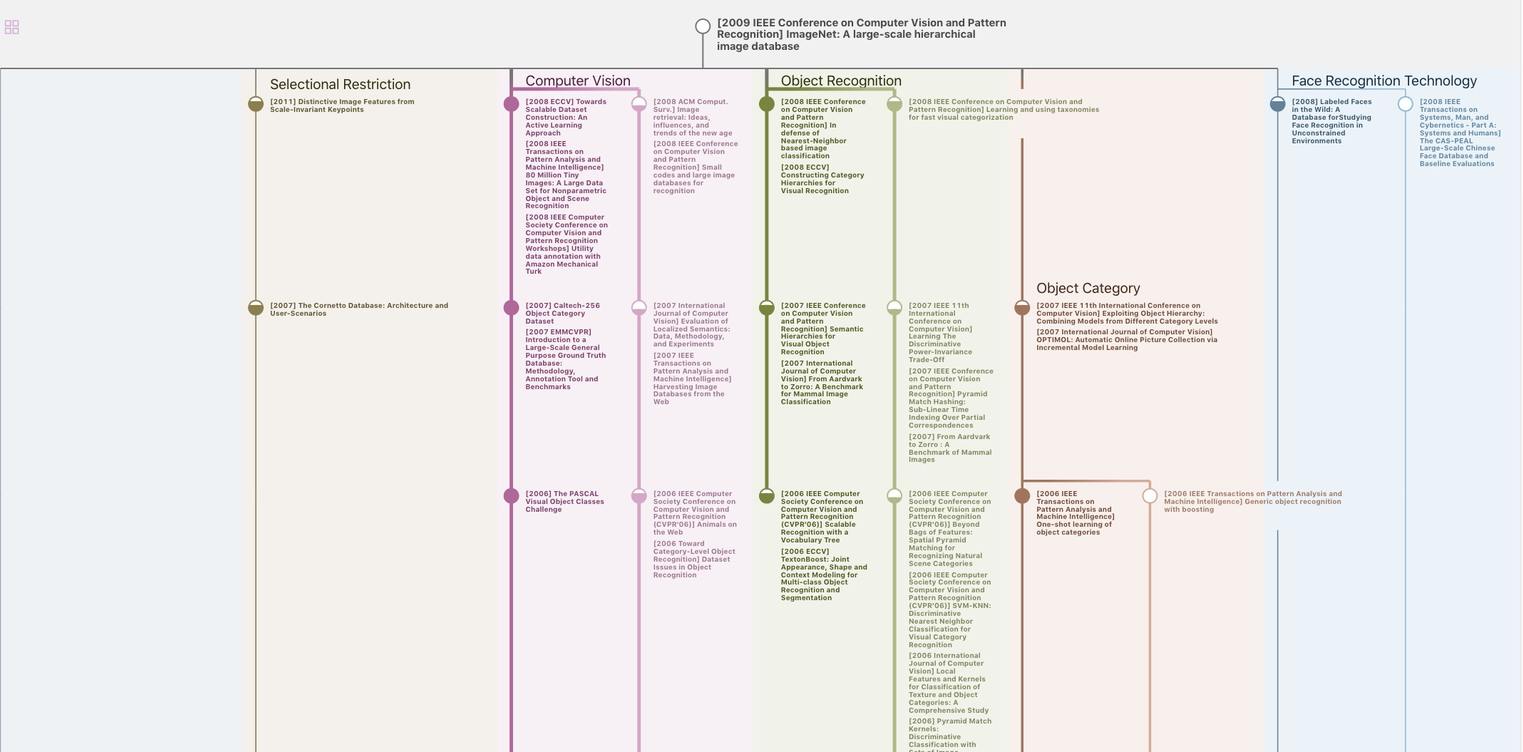
生成溯源树,研究论文发展脉络
Chat Paper
正在生成论文摘要