Multi-modal Adapter for Medical Vision-and-Language Learning
MACHINE LEARNING IN MEDICAL IMAGING, MLMI 2023, PT I(2024)
摘要
Recently, medical vision-and-language learning has attracted great attention from biomedical communities. Thanks to the development of large pre-trained models, the performances on these medical multi-modal learning benchmarks have been greatly improved. However, due to the rapid growth of the model size, full fine-tuning these large pre-trained models has become costly in training and storing such huge parameters for each downstream task. Thus, we propose a parameter-efficient transfer learning method named Medical Multi-Modal Adapter (M(3)AD) to mediate this problem. We select the state-of-the-art M(3)AE model as our baseline, which is pre-trained on 30k medical image-text pairs with multiple proxy tasks and has about 340M parameters. To be specific, we first insert general adapters after multi-head attention layers and feed-forward layers in all transformer blocks of M(3)AE. Then, we specifically design a modality-fusion adapter that adopts multi-head attention mechanisms and we insert them in the cross-modal encoder to enhance the multi-modal interactions. Compared to full fine-tuning, we freeze most parameters in M(3)AE and only train these inserted adapters with much smaller sizes. Extensive experimental results on three medical visual question answering datasets and one medical multi-modal classification dataset demonstrate the effectiveness of our proposed method, where M(3)AD achieves competitive performances compared to full fine-tuning with much fewer training parameters and memory consumption.
更多查看译文
关键词
Medical Vision-and-Language Learning,Parameter-Efficient Transfer Learning,Multi-Modal Adapter
AI 理解论文
溯源树
样例
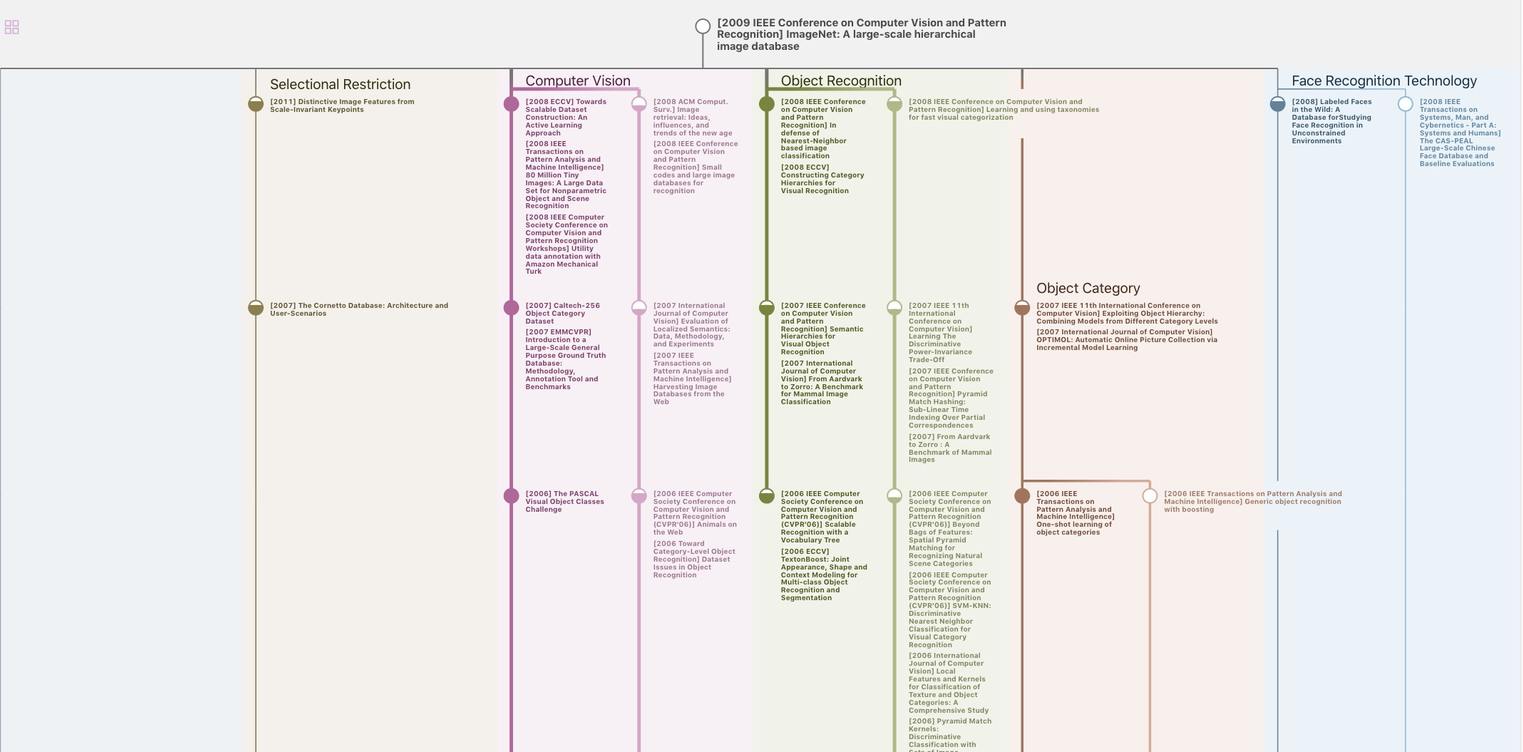
生成溯源树,研究论文发展脉络
Chat Paper
正在生成论文摘要