Cascaded binary classifiers for blind Beam Alignment in mmWave MIMO using one-bit quantization
2023 IEEE INTERNATIONAL CONFERENCE ON COMMUNICATIONS WORKSHOPS, ICC WORKSHOPS(2023)
摘要
This paper proposes a new approach for partial and blind Machine Learning (ML)-based Beam Alignment (BA) for massive mmWave MIMO. It models an uplink scenario using one-bit quantization through a low-complexity fully-analog system architecture. The proposed BA is based on sub-sampled codebooks holding possible beam patterns at UE and BS. We propose to sound a small subset of beams based on instantaneous Received Signal Energies (RSE). These sounded RSE values are then quantized into binary integers. The proposed cascaded structure of Binary Logistic Regression (BLR) aims to iteratively filter the large dataset input-matrix (by deleting low-RSE beams) into a smaller one where our benchmark, the Exhaustive BA is feasible and the signaling overhead remains low. In addition to the theoretical monotonicconvergence guarantees, BLR has good classification quality and low computational complexity. Our extensive numerical simulations illustrate encountering the large signaling overhead problem with high prediction accuracy using one-bit quantization scheme and 14% of the total beam samples.
更多查看译文
关键词
Millimeter Wave MIMO,massive antennas,(ML)-based Beam Alignment,blind BA,one-bit quantization,binary classification,logistic regression
AI 理解论文
溯源树
样例
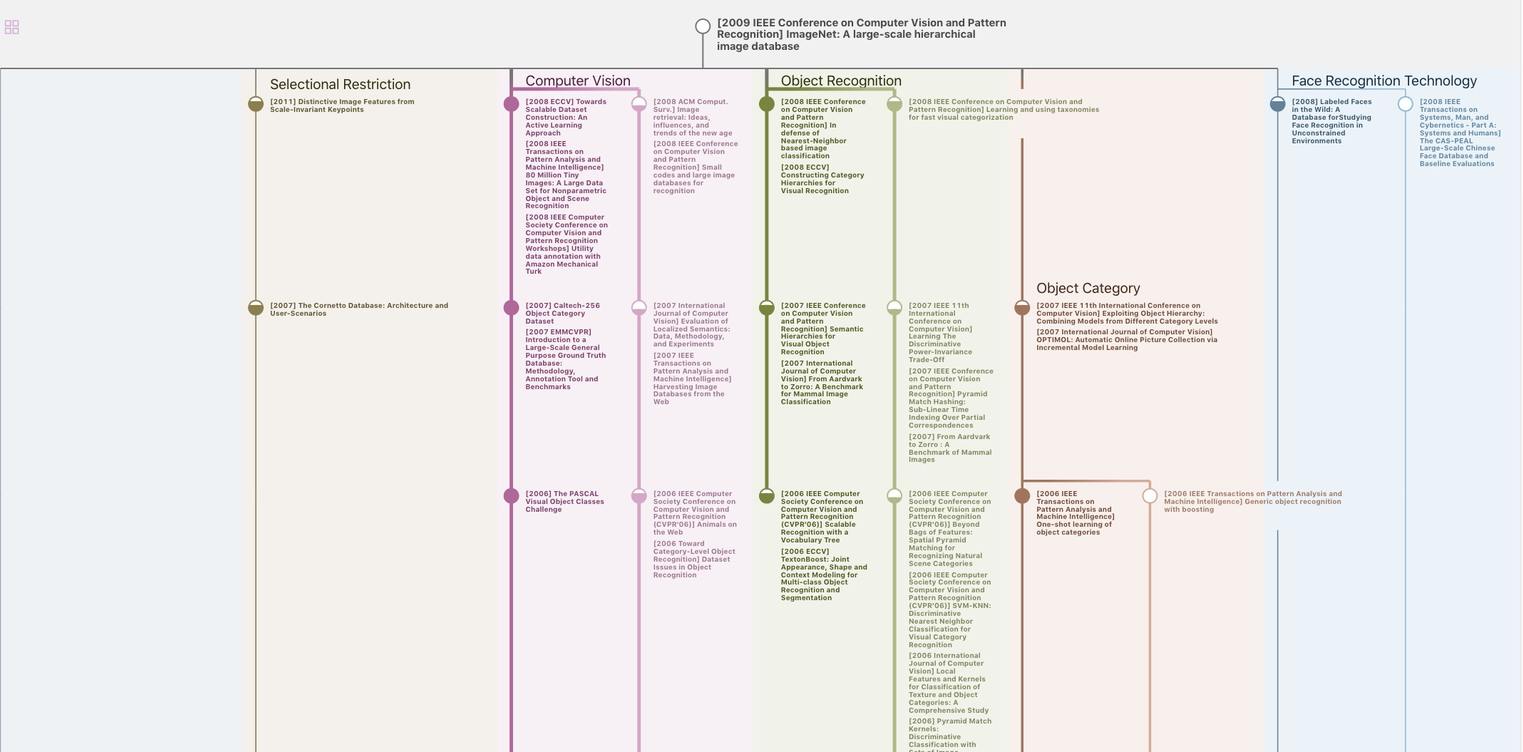
生成溯源树,研究论文发展脉络
Chat Paper
正在生成论文摘要