DIN: A Decentralized Inexact Newton Algorithm for Consensus Optimization
ICC 2023 - IEEE INTERNATIONAL CONFERENCE ON COMMUNICATIONS(2023)
摘要
In this paper, we consider a decentralized consensus optimization problem defined over a network of inter-connected devices that collaboratively solve the problem using only local data and information exchange with their neighbours. Despite their fast convergence, Newton-type methods require sending Hessian information between devices, making them communication inefficient while violating the devices' privacy. By formulating the Newton direction learning problem as a sum of separable functions subjected to a consensus constraint, our proposed approach learns an inexact Newton direction alongside the global model using the proximal primal-dual (Prox-PDA) algorithm. Our algorithm, coined DIN, avoids sharing Hessian information between devices since each device shares a model-sized vector, concealing the first- and second-order information, reducing the network's burden and improving communication and energy efficiencies. Numerical simulations corroborate that DIN exhibits higher communication efficiency in terms of communication rounds while consuming less communication and computation energy compared to existing second-order decentralized base-lines.
更多查看译文
关键词
Distributed optimization,decentralized learning,communication-efficient federated learning,second-order methods
AI 理解论文
溯源树
样例
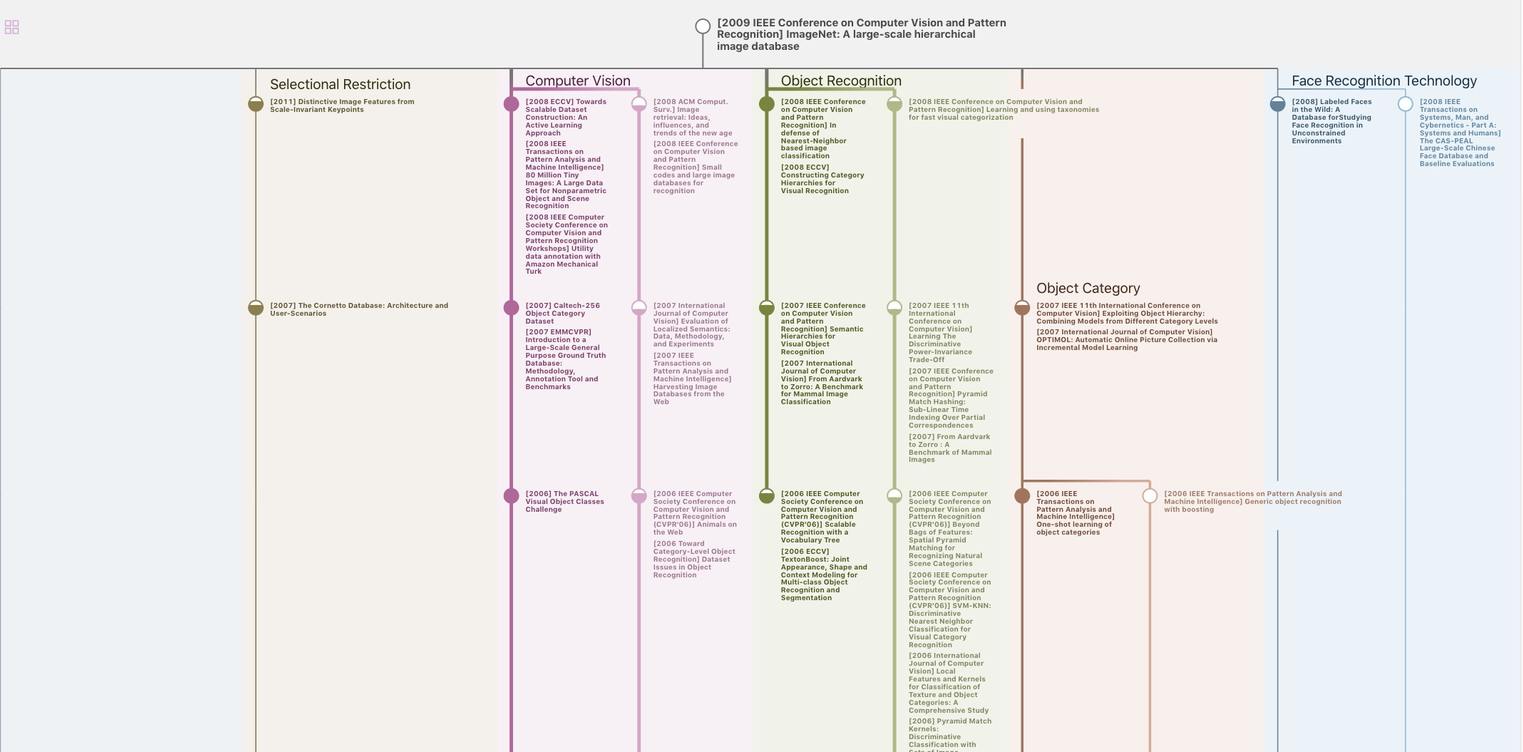
生成溯源树,研究论文发展脉络
Chat Paper
正在生成论文摘要